Characterising Heavy-Tailed Networks Using Q-Generalised Entropy And Q-Adjacency Kernels
PHYSICA A-STATISTICAL MECHANICS AND ITS APPLICATIONS(2021)
摘要
Heavy-tailed networks, which have degree distributions characterised by slower than exponentially bounded tails, are common in many different situations. Some interesting cases, where heavy tails are characterised by inverse powers A in the range 1 < lambda < 2, arise for associative knowledge networks, and semantic and linguistic networks. In these cases, the differences between the networks are often delicate, calling for robust methods to characterise the differences. Here, we introduce a method for comparing networks using a density matrix based on q-generalised adjacency matrix kernels. It is shown that comparison of networks can then be performed using the q-generalised Kullback-Leibler divergence. In addition, the q-generalised divergence can be interpreted as a q-generalised free energy, which enables the thermodynamic-like macroscopic description of the heavy-tailed networks. The viability of the q-generalised adjacency kernels and the thermodynamic-like description in characterisation of complex networks is demonstrated using a simulated set of networks, which are modular and heavy-tailed with a degree distribution of inverse power law in the range 1 < lambda < 2. (C) 2020 Elsevier B.V. All rights reserved.
更多查看译文
关键词
Heavy tailed networks, Adjacency kernels, q-entropy, Generalised thermodynamics
AI 理解论文
溯源树
样例
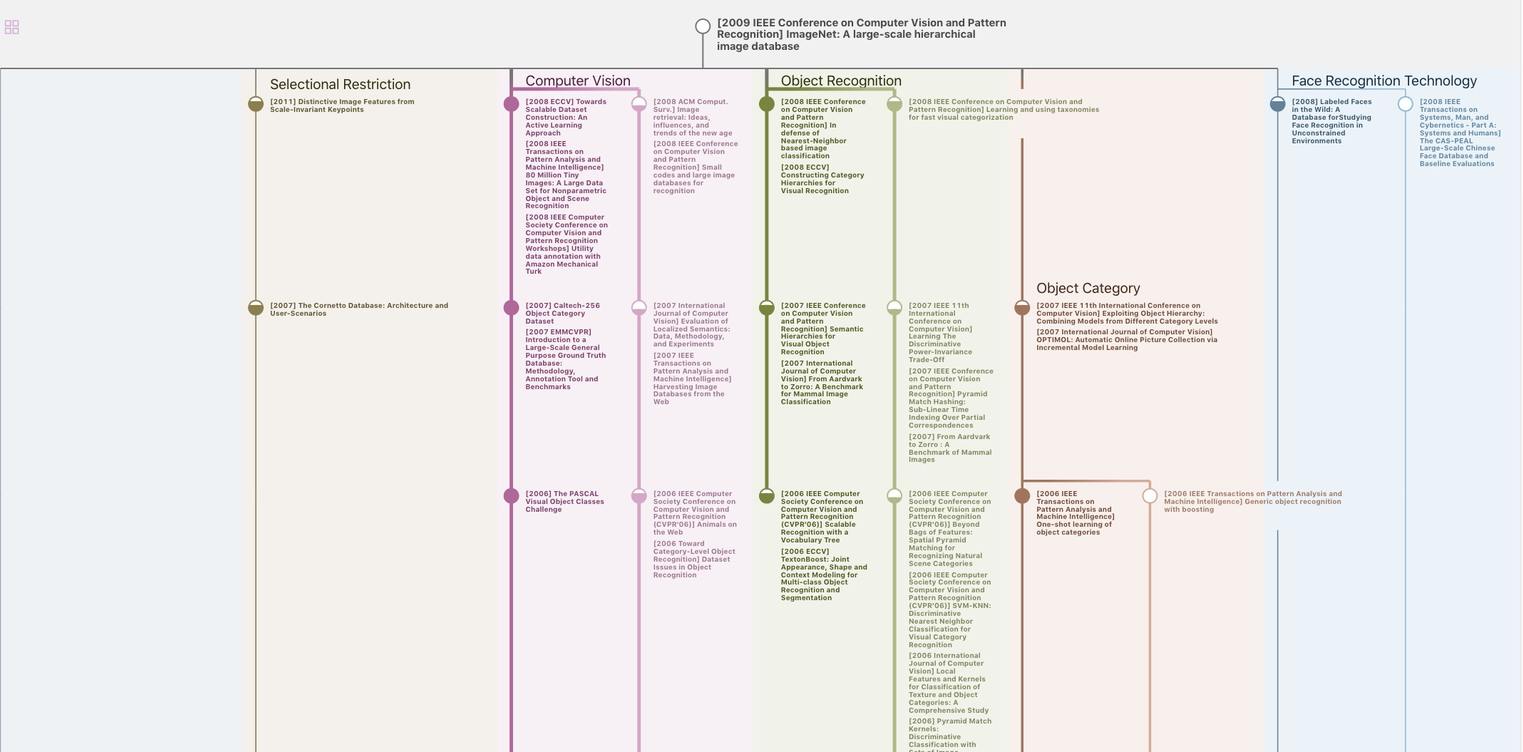
生成溯源树,研究论文发展脉络
Chat Paper
正在生成论文摘要