Secrets of 3D Implicit Object Shape Reconstruction in the Wild
arxiv(2021)
摘要
Reconstructing high-fidelity 3D objects from sparse, partial observation is of crucial importance for various applications in computer vision, robotics, and graphics. While recent neural implicit modeling methods show promising results on synthetic or dense datasets, they perform poorly on real-world data that is sparse and noisy. This paper analyzes the root cause of such deficient performance of a popular neural implicit model. We discover that the limitations are due to highly complicated objectives, lack of regularization, and poor initialization. To overcome these issues, we introduce two simple yet effective modifications: (i) a deep encoder that provides a better and more stable initialization for latent code optimization; and (ii) a deep discriminator that serves as a prior model to boost the fidelity of the shape. We evaluate our approach on two real-wold self-driving datasets and show superior performance over state-of-the-art 3D object reconstruction methods.
更多查看译文
关键词
3d,shape,wild
AI 理解论文
溯源树
样例
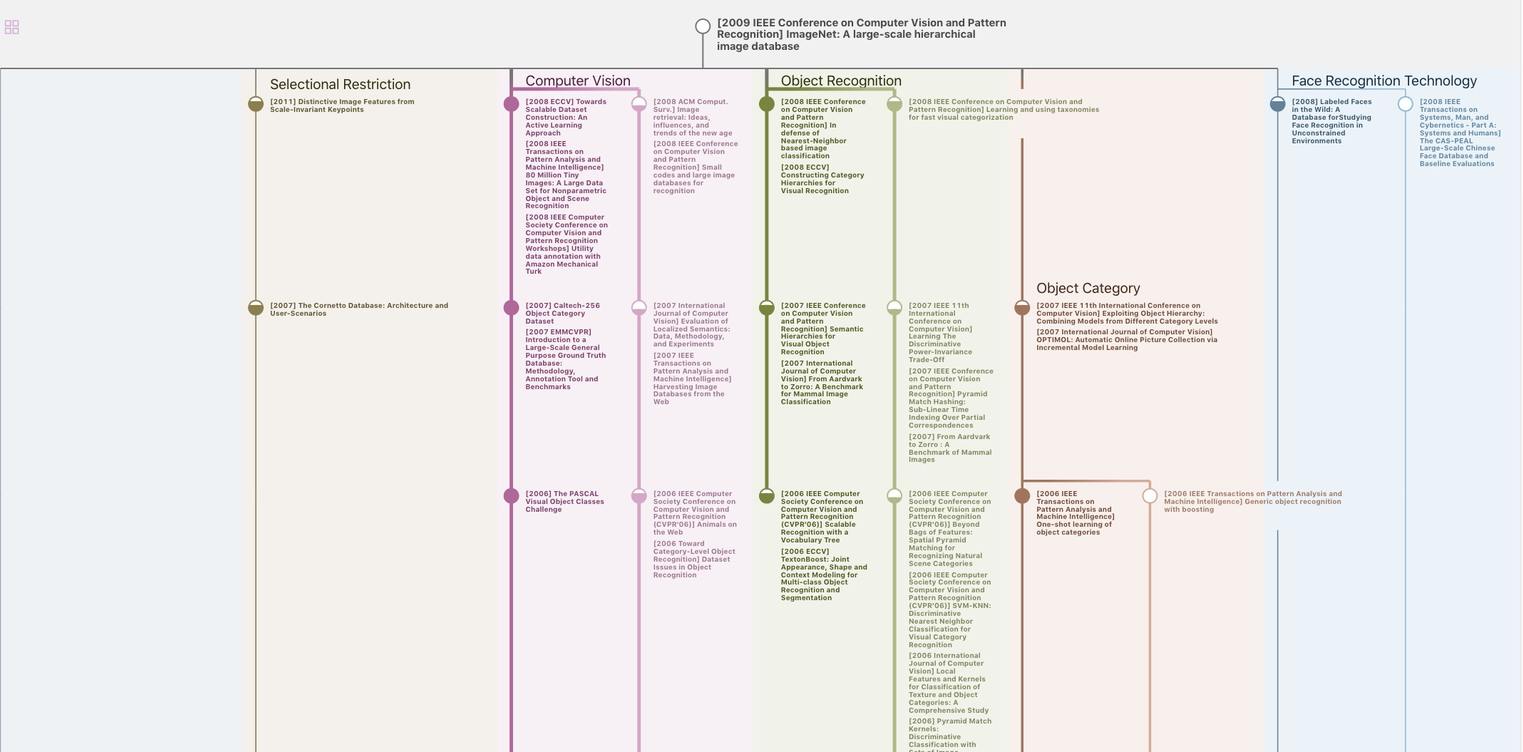
生成溯源树,研究论文发展脉络
Chat Paper
正在生成论文摘要