Auto4D: Learning to Label 4D Objects from Sequential Point Clouds
arxiv(2021)
摘要
In the past few years we have seen great advances in 3D object detection thanks to deep learning methods. However, they typically rely on large amounts of high-quality labels to achieve good performance, which often require time-consuming and expensive work by human annotators. To address this we propose an automatic annotation pipeline that generates accurate object trajectories in 3D (ie, 4D labels) from LiDAR point clouds. Different from previous works that consider single frames at a time, our approach directly operates on sequential point clouds to combine richer object observations. The key idea is to decompose the 4D label into two parts: the 3D size of the object, and its motion path describing the evolution of the object's pose through time. More specifically, given a noisy but easy-to-get object track as initialization, our model first estimates the object size from temporally aggregated observations, and then refines its motion path by considering both frame-wise observations as well as temporal motion cues. We validate the proposed method on a large-scale driving dataset and show that our approach achieves significant improvements over the baselines. We also showcase the benefits of our approach under the annotator-in-the-loop setting.
更多查看译文
AI 理解论文
溯源树
样例
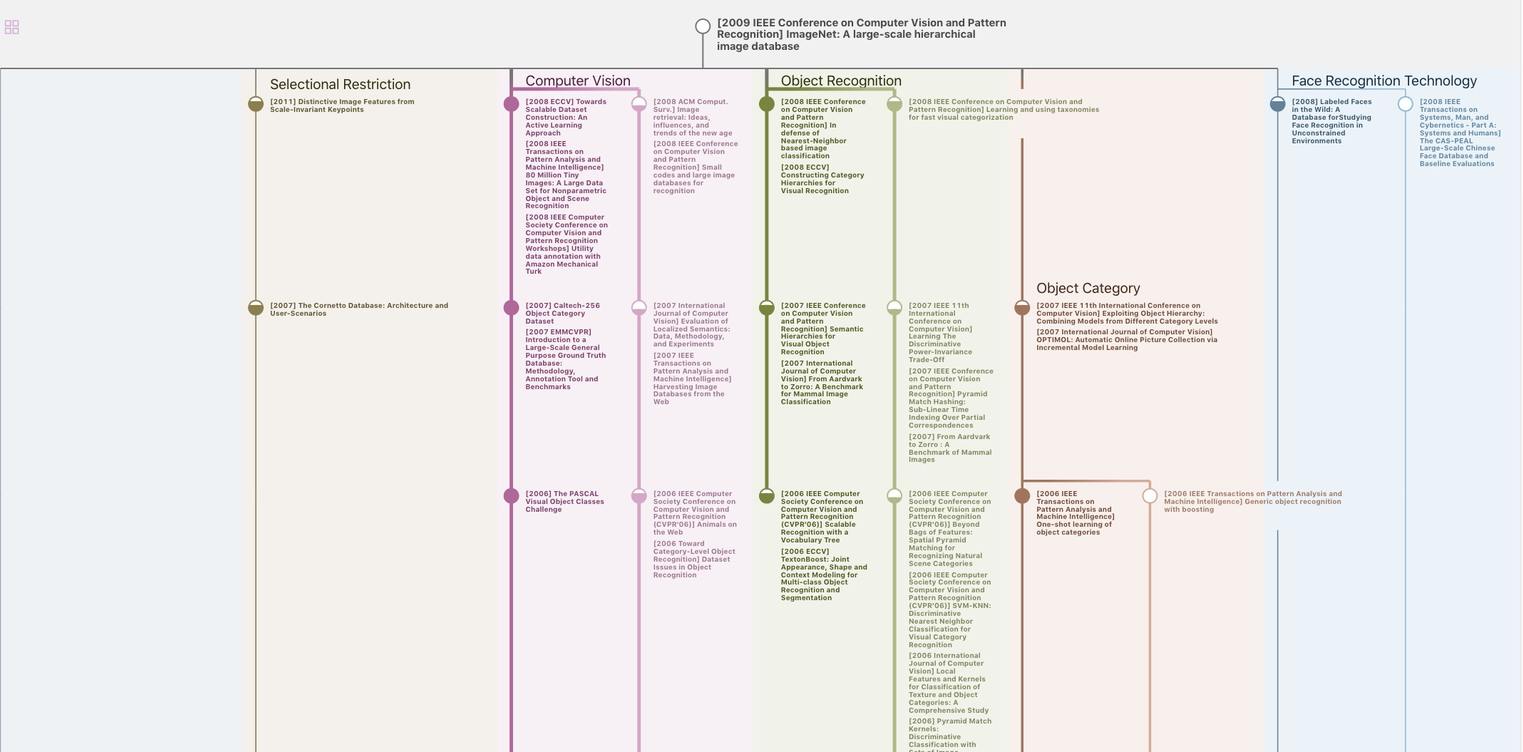
生成溯源树,研究论文发展脉络
Chat Paper
正在生成论文摘要