Learning hydrodynamic equations for active matter from particle simulations and experiments.
Proceedings of the National Academy of Sciences of the United States of America(2023)
摘要
Recent advances in high-resolution imaging techniques and particle-based simulation methods have enabled the precise microscopic characterization of collective dynamics in various biological and engineered active matter systems. In parallel, data-driven algorithms for learning interpretable continuum models have shown promising potential for the recovery of underlying partial differential equations (PDEs) from continuum simulation data. By contrast, learning macroscopic hydrodynamic equations for active matter directly from experiments or particle simulations remains a major challenge, especially when continuum models are not known a priori or analytic coarse graining fails, as often is the case for nondilute and heterogeneous systems. Here, we present a framework that leverages spectral basis representations and sparse regression algorithms to discover PDE models from microscopic simulation and experimental data, while incorporating the relevant physical symmetries. We illustrate the practical potential through a range of applications, from a chiral active particle model mimicking nonidentical swimming cells to recent microroller experiments and schooling fish. In all these cases, our scheme learns hydrodynamic equations that reproduce the self-organized collective dynamics observed in the simulations and experiments. This inference framework makes it possible to measure a large number of hydrodynamic parameters in parallel and directly from video data.
更多查看译文
关键词
active matter,coarse-graining,hydrodynamic equations,sparse regression
AI 理解论文
溯源树
样例
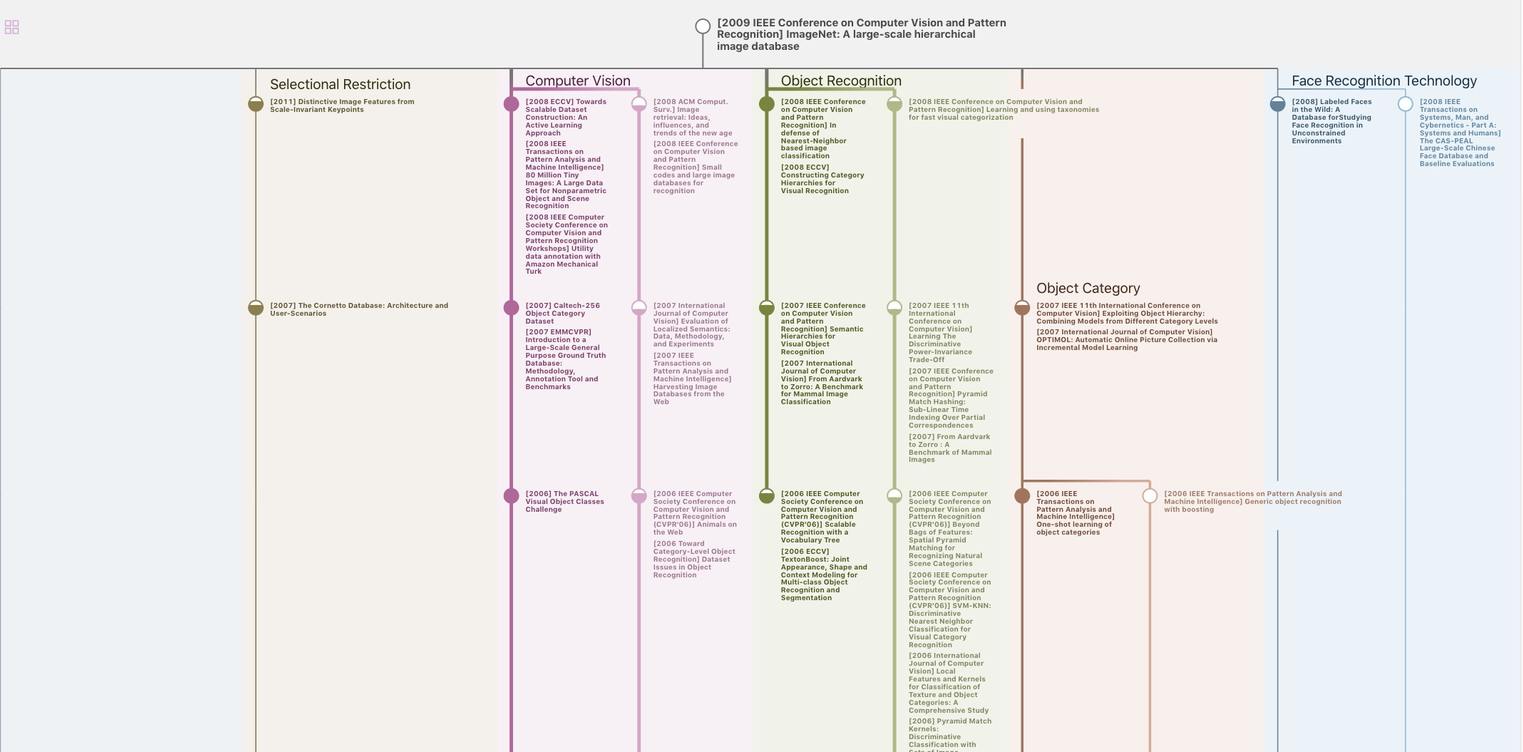
生成溯源树,研究论文发展脉络
Chat Paper
正在生成论文摘要