Polymorphic Adversarial DDoS attack on IDS using GAN.
ISNCC(2020)
摘要
Intrusion Detection systems are important tools in preventing malicious traffic from penetrating into networks and systems. Recently, Intrusion Detection Systems are rapidly enhancing their detection capabilities using machine learning algorithms. However, these algorithms are vulnerable to new unknown types of attacks that can evade machine learning IDS. In particular, they may be vulnerable to attacks based on Generative Adversarial Networks (GAN). GANs have been widely used in domains such as image processing, natural language processing to generate adversarial data of different types such as graphics, videos, texts, etc. We propose a model using GAN to generate adversarial DDoS attacks that can change the attack profile and can be undetected. Our simulation results indicate that by continuous changing of attack profile, defensive systems that use incremental learning will still be vulnerable to new attacks.
更多查看译文
关键词
Adversarial Attacks,Generative Adversarial Networks (GAN),IDS,DDoS attacks,Machine Learning
AI 理解论文
溯源树
样例
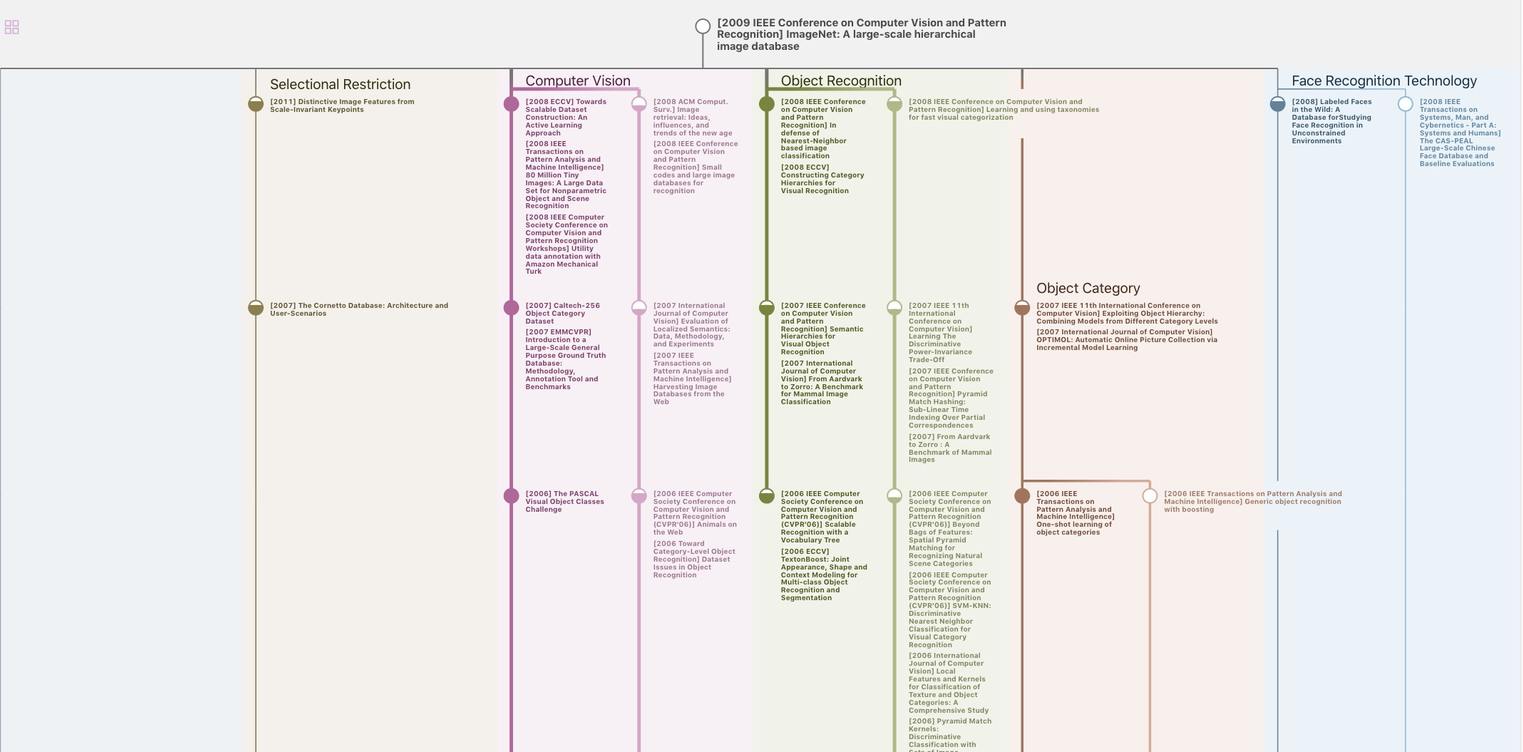
生成溯源树,研究论文发展脉络
Chat Paper
正在生成论文摘要