A Multi-Task Reinforcement Learning Approach For Navigating Unsignalized Intersections
2020 IEEE INTELLIGENT VEHICLES SYMPOSIUM (IV)(2020)
摘要
Navigating through unsignalized intersections is one of the most challenging problems in urban environments for autonomous vehicles. Existing methods need to train specific policy models to deal with different tasks including going straight, turning left and turning right. In this paper we formulate intersection navigation as a multi-task reinforcement learning problem and propose a unified learning framework for all three navigation tasks at the intersections. We propose to represent multiple tasks with a unified four-dimensional vector, which elements mean a common sub-task and three specific target sub-tasks respectively. Meanwhile, we design a vectorized reward function combining with deep Q-networks (DQN) to learn to handle multiple intersection navigation tasks concurrently. We train the agent to navigate through intersections by adjusting the speed of the ego vehicle under given route. Experimental results in both simulation and real-world vehicle test demonstrate that the proposed multi-task DQN algorithm outperforms baselines for all three navigation tasks in several different intersection scenarios.
更多查看译文
关键词
multitask DQN,multitask reinforcement learning approach,navigating unsignalized intersections,autonomous vehicles,unified learning framework,target sub-tasks,deep Q-networks,ego vehicle,reward function,four-dimensional vector,multiple intersection navigation tasks
AI 理解论文
溯源树
样例
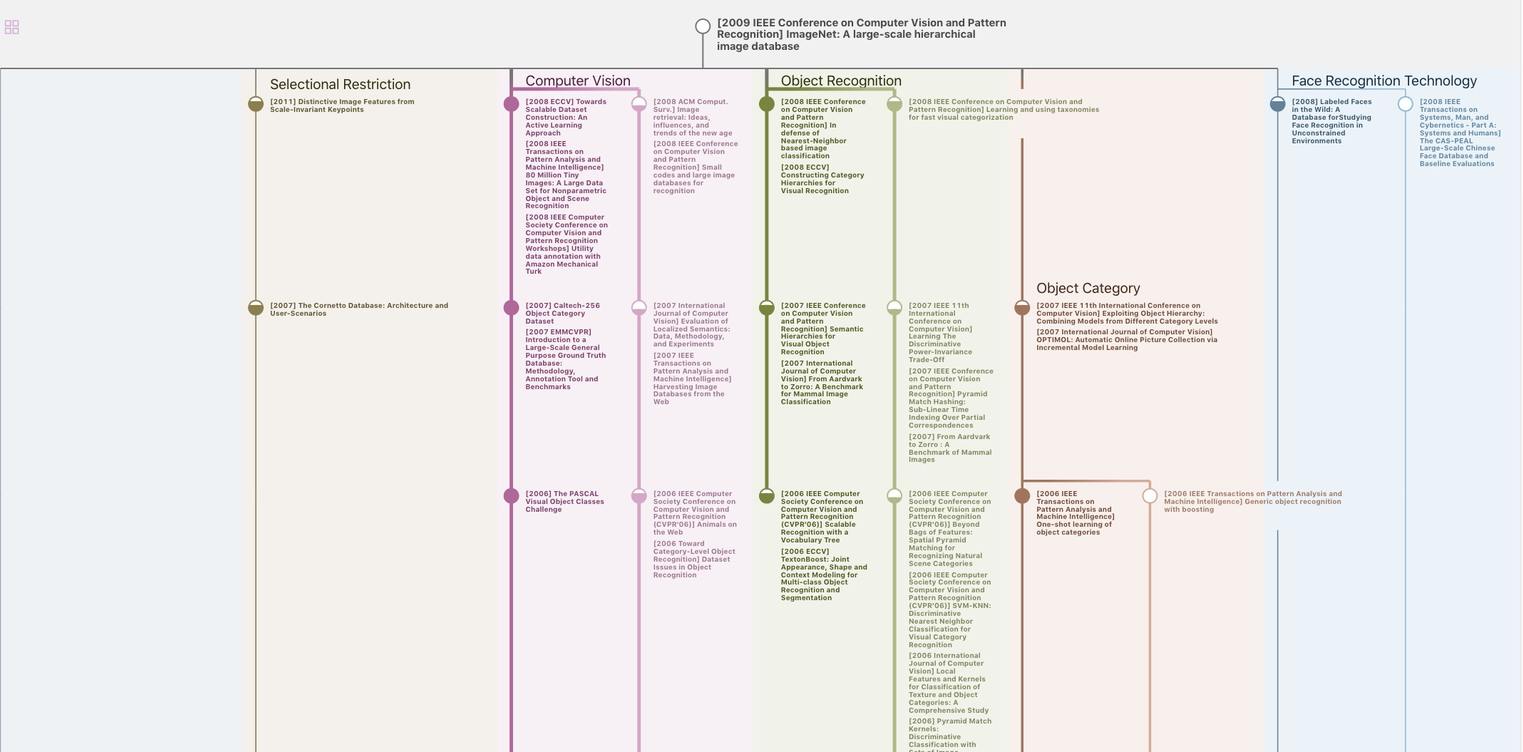
生成溯源树,研究论文发展脉络
Chat Paper
正在生成论文摘要