A Distributed Approach for Estimation of Information Matrix in Smart Grids and its Application for Anomaly Detection
2020 IEEE International Conference on Communications, Control, and Computing Technologies for Smart Grids (SmartGridComm)(2020)
摘要
Statistical structure learning (SSL)-based approaches have been employed in the recent years to detect different types of anomalies in a variety of cyber-physical systems (CPS). Although these approaches outperform conventional methods in the literature, their computational complexity, need for large number of measurements and centralized computations have limited their applicability to large-scale networks. In this work, we propose a distributed, multi-agent maximum likelihood (ML) approach to detect anomalies in smart grid applications aiming at reducing computational complexity, as well as preserving data privacy among different players in the network. The proposed multi-agent detector breaks the original ML problem into several local (smaller) ML optimization problems coupled by the alternating direction method of multipliers (ADMM). Then, these local ML problems are solved by their corresponding agents, eventually resulting in the construction of the global solution (network's information matrix). The numerical results obtained from two IEEE test (power transmission) systems confirm the accuracy and efficiency of the proposed approach for anomaly detection.
更多查看译文
关键词
statistical structure learning-based approaches,smart grids,distributed approach,anomaly detection,IEEE test systems,information matrix,corresponding agents,local ML problems,alternating direction method,local ML optimization problems,original ML problem,multiagent detector,different players,smart grid applications,multiagent maximum likelihood approach,large-scale networks,centralized computations,computational complexity,cyber-physical systems
AI 理解论文
溯源树
样例
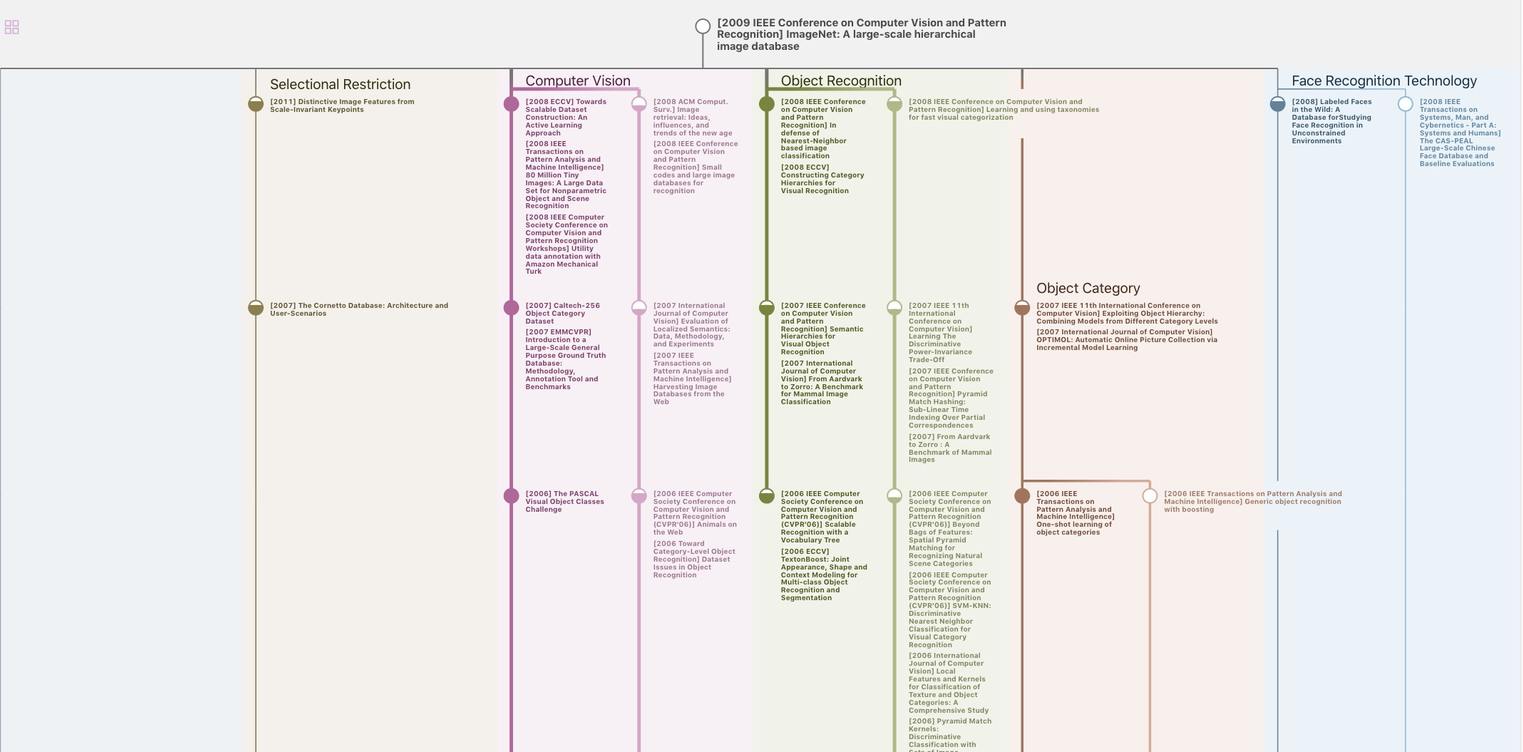
生成溯源树,研究论文发展脉络
Chat Paper
正在生成论文摘要