Data Fusion With Model-Based Machine Learning For Weighted Least Squares Based Positioning
2020 14th International Conference on Signal Processing and Communication Systems (ICSPCS)(2020)
摘要
This work presents data fusion techniques with Machine Learning based algorithms for hybrid cooperative positioning. More precisely, model-based Machine Learning methods built on Bayesian inference in Bayesian Networks using a Belief Propagation algorithm. The linear systems consist of Gaussian Factor Graphs (FG) for knowing the probability of target position given the distance measurements to anchor nodes that know its position. The models are based on Least Squares (LS) and Weighted LS (WLS) algorithms with anchor based positioning. It is assumed that the nodes are able to estimate range with wireless technologies, such as IEEE 802.15.4-UWB, and different ranging techniques such as those based on Time of Arrival (two-way). One of the main objectives of this work is to study techniques based on Machine Learning to improve positioning both in good and challenging scenarios. Simulation results show that the presented FG with WLS algorithm achieves better results than the LS and WLS algorithms in various scenarios with good and poor conditions for positioning.
更多查看译文
关键词
Data Fusion,Model-based Machine Learning,Factor Graph,Belief Propagation,Hybrid Cooperative Positioning,Weighted Least Squares
AI 理解论文
溯源树
样例
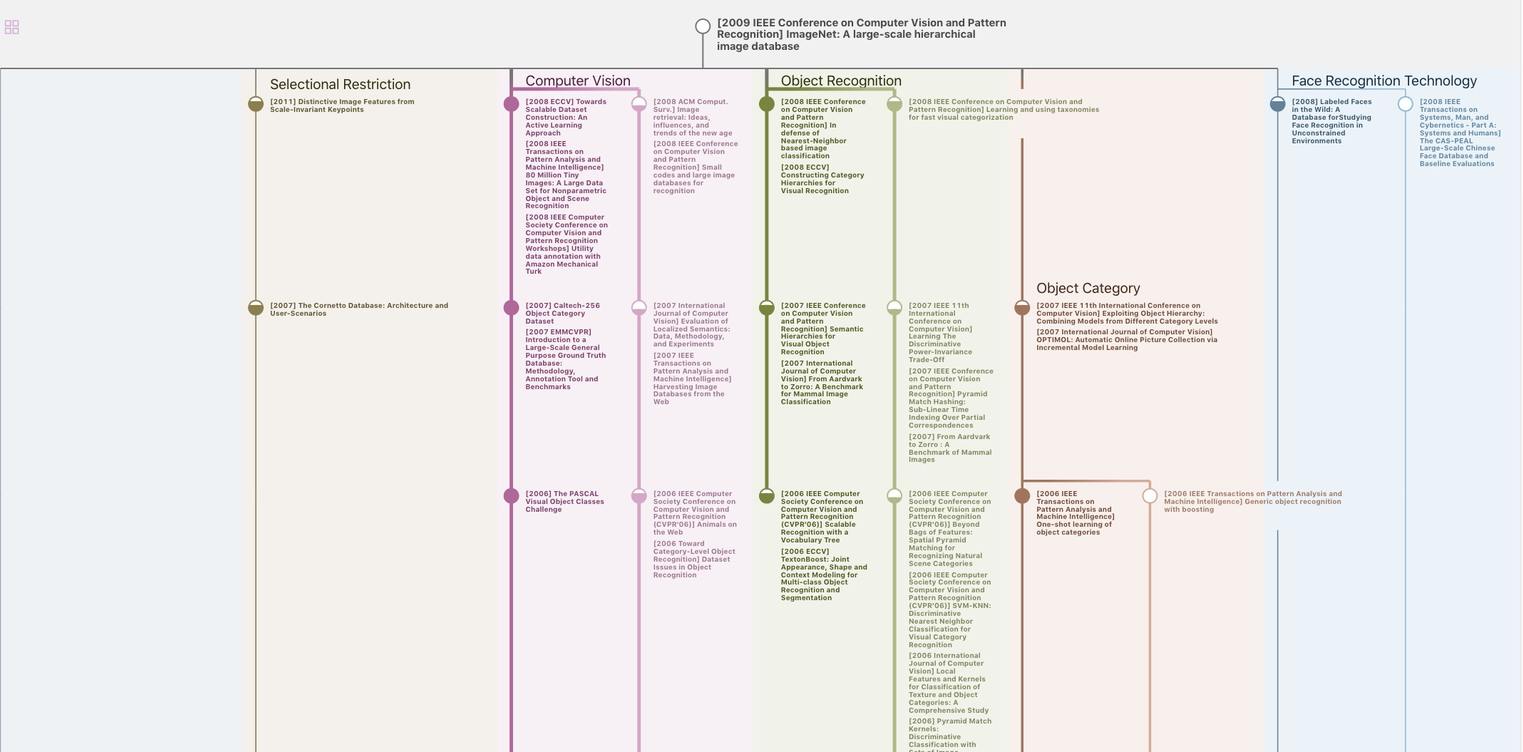
生成溯源树,研究论文发展脉络
Chat Paper
正在生成论文摘要