Low-Rank Temporal Attention-Augmented Bilinear Network for financial time-series forecasting.
SSCI(2020)
摘要
Financial market analysis, especially the prediction of movements of stock prices, is a challenging problem. The nature of financial time-series data, being non-stationary and nonlinear, is the main cause of these challenges. Deep learning models have led to significant performance improvements in many problems coming from different domains, including prediction problems of financial time-series data. Although the prediction performance is the main goal of such models, dealing with ultra high-frequency data sets restrictions in terms of the number of model parameters and its inference speed. The Temporal Attention-Augmented Bilinear network was recently proposed as an efficient and high-performing model for Limit Order Book time-series forecasting. In this paper, we propose a low-rank tensor approximation of the model to further reduce the number of trainable parameters and increase its speed.
更多查看译文
关键词
Deep learning, Low-rank tensor decomposition, Limit Order Book data, Financial lime-series analysis
AI 理解论文
溯源树
样例
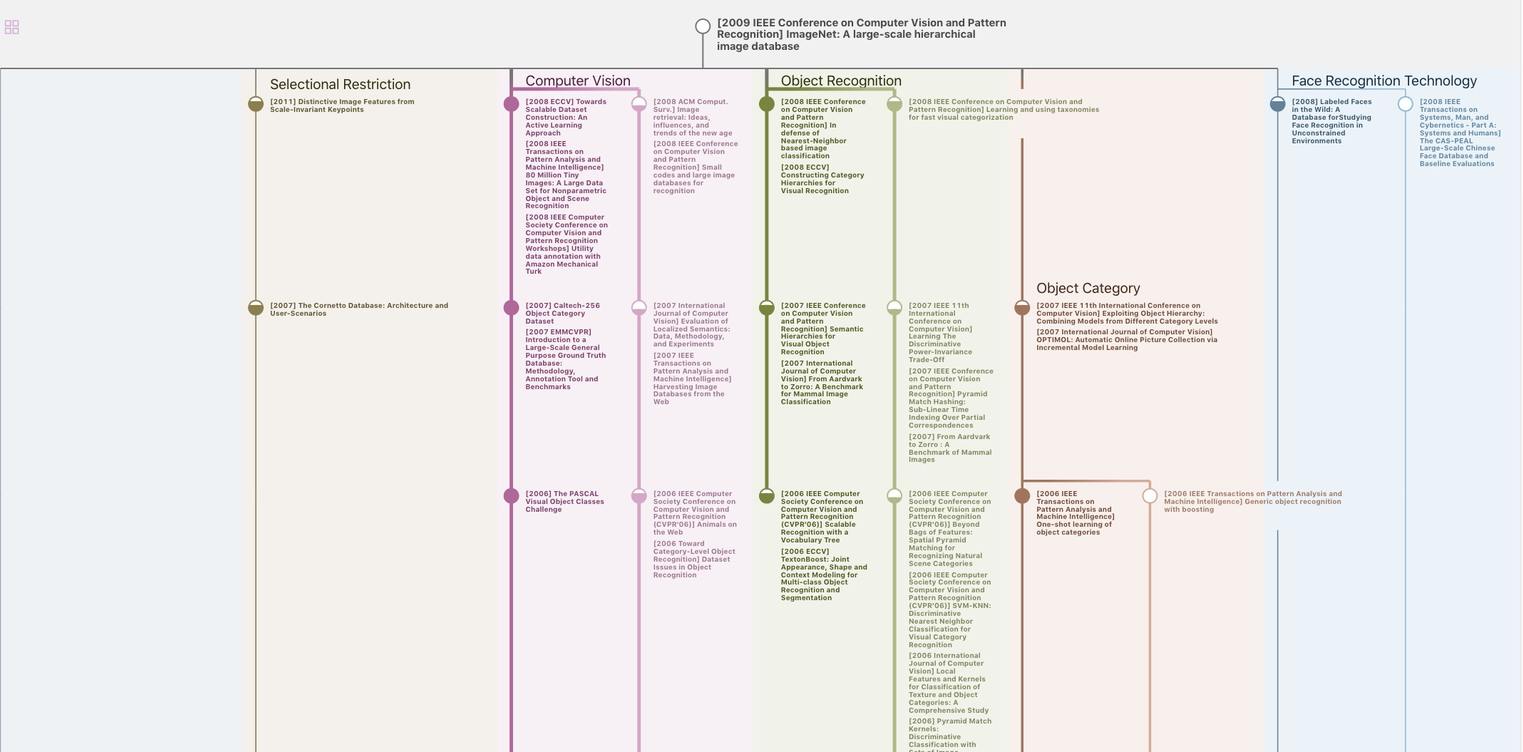
生成溯源树,研究论文发展脉络
Chat Paper
正在生成论文摘要