Composition of Saliency Metrics for Pruning with a Myopic Oracle.
SSCI(2020)
摘要
The cost of Convolutional Neural Network (CNN) inference can be reduced by pruning weights from a trained network, eliminating computations while preserving the predictive accuracy up to some threshold. While many heuristic saliency metrics have been proposed to guide this process, the quality of pruning decisions made by any one metric is highly context-sensitive. Some metrics make excellent pruning decisions for one network, while making poor decisions for other networks. Traditionally, a single heuristic saliency metric is used for the entire pruning process. We show how to compose a set of these saliency metrics to form a much more robust (albeit still heuristic) saliency. The key idea is to exploit the cases where the different base metrics do well, and avoid the cases where they do poorly by switching to a different metric. With an experimental evaluation of channel pruning on several popular CNNs on the CIFAR-10 and CIFAR-100 datasets, we show that the composite saliency metrics derived by our method consistently outperform all of the individual constituent metrics.
更多查看译文
关键词
Machine Learning, Convolutional Neural Networks, Pruning
AI 理解论文
溯源树
样例
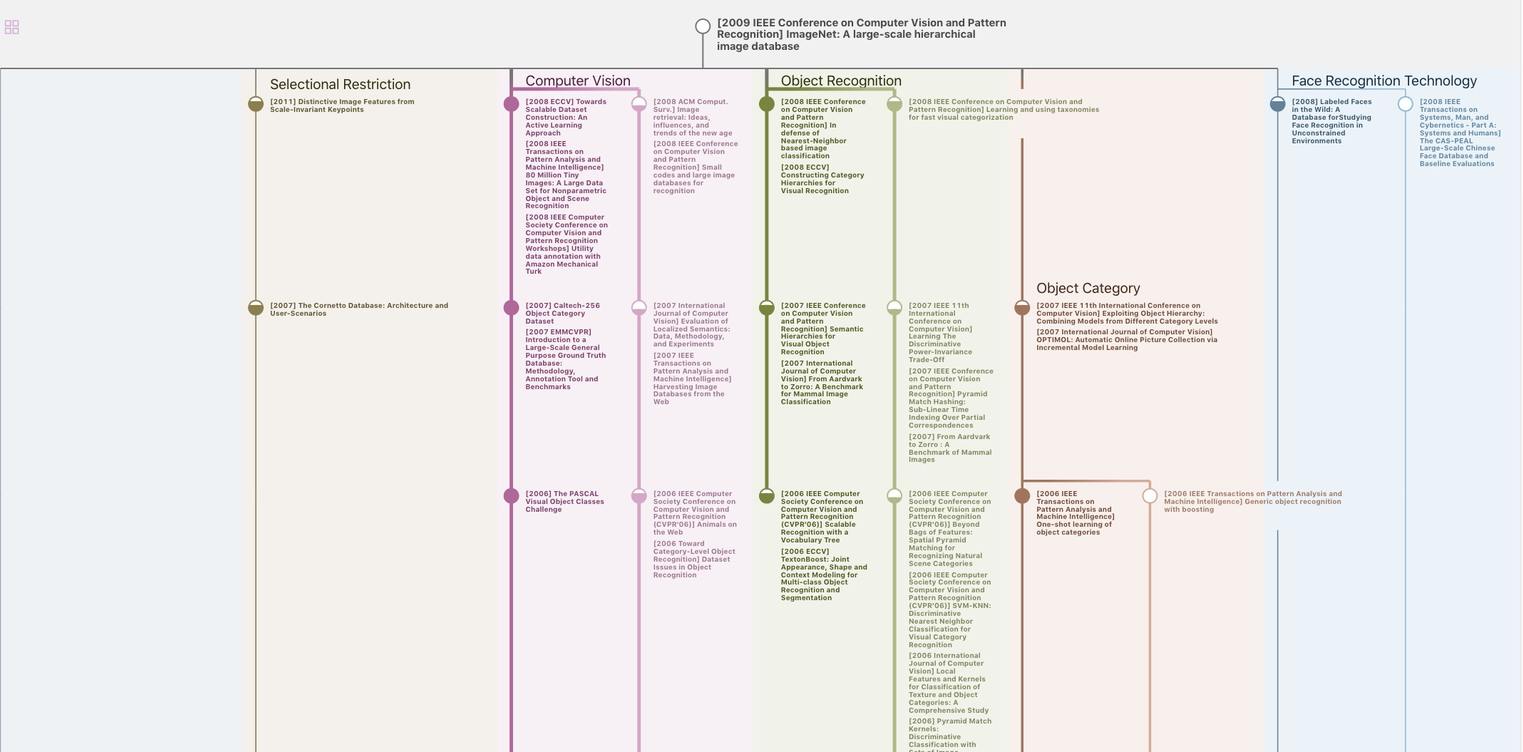
生成溯源树,研究论文发展脉络
Chat Paper
正在生成论文摘要