TrNews: Heterogeneous User-Interest Transfer Learning for News Recommendation
EACL(2021)
摘要
We investigate how to solve the cross-corpus news recommendation for unseen users in the future. This is a problem where traditional content-based recommendation techniques often fail. Luckily, in real-world recommendation services, some publisher (e.g., Daily news) may have accumulated a large corpus with lots of consumers which can be used for a newly deployed publisher (e.g., Political news). To take advantage of the existing corpus, we propose a transfer learning model (dubbed as TrNews) for news recommendation to transfer the knowledge from a source corpus to a target corpus. To tackle the heterogeneity of different user interests and of different word distributions across corpora, we design a translator-based transfer-learning strategy to learn a representation mapping between source and target corpora. The learned translator can be used to generate representations for unseen users in the future. We show through experiments on real-world datasets that TrNews is better than various baselines in terms of four metrics. We also show that our translator is effective among existing transfer strategies.
更多查看译文
关键词
recommendation,trnews,user-interest
AI 理解论文
溯源树
样例
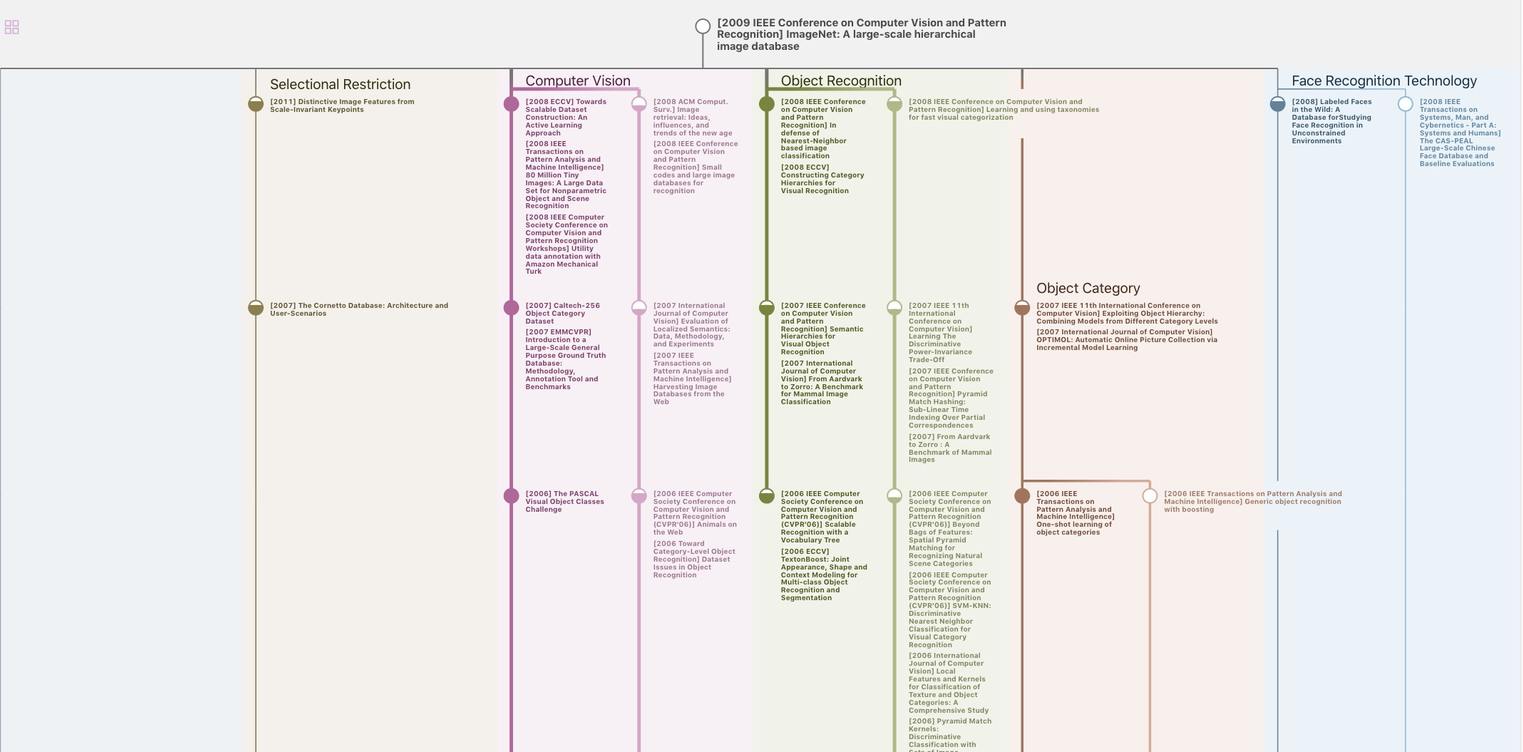
生成溯源树,研究论文发展脉络
Chat Paper
正在生成论文摘要