Image Deblurring based on Lightweight Multi-Information Fusion Network.
ICIP(2021)
摘要
Recently, deep learning based image deblurring has been well developed. However, exploiting the detailed image features in a deep learning framework always requires a mass of parameters, which inevitably makes the network suffer from high computational burden. To solve this problem, we propose a lightweight multiinformation fusion network (LMFN) for image deblurring. The proposed LMFN is designed as an encoder-decoder architecture. In the encoding stage, the image feature is reduced to various smallscale spaces for multi-scale information extraction and fusion without a large amount of information loss. Then, a distillation network is used in the decoding stage, which allows the network benefit the most from residual learning while remaining sufficiently lightweight. Meanwhile, an information fusion strategy between distillation modules and feature channels is also carried out by attention mechanism. Through fusing different information in the proposed approach, our network can achieve state-of-the-art image deblurring result with smaller number of parameters and outperforms existing methods in model complexity.
更多查看译文
关键词
Image deblurring,multi-information fusion network,lightweight distillation block,fusion mechanism
AI 理解论文
溯源树
样例
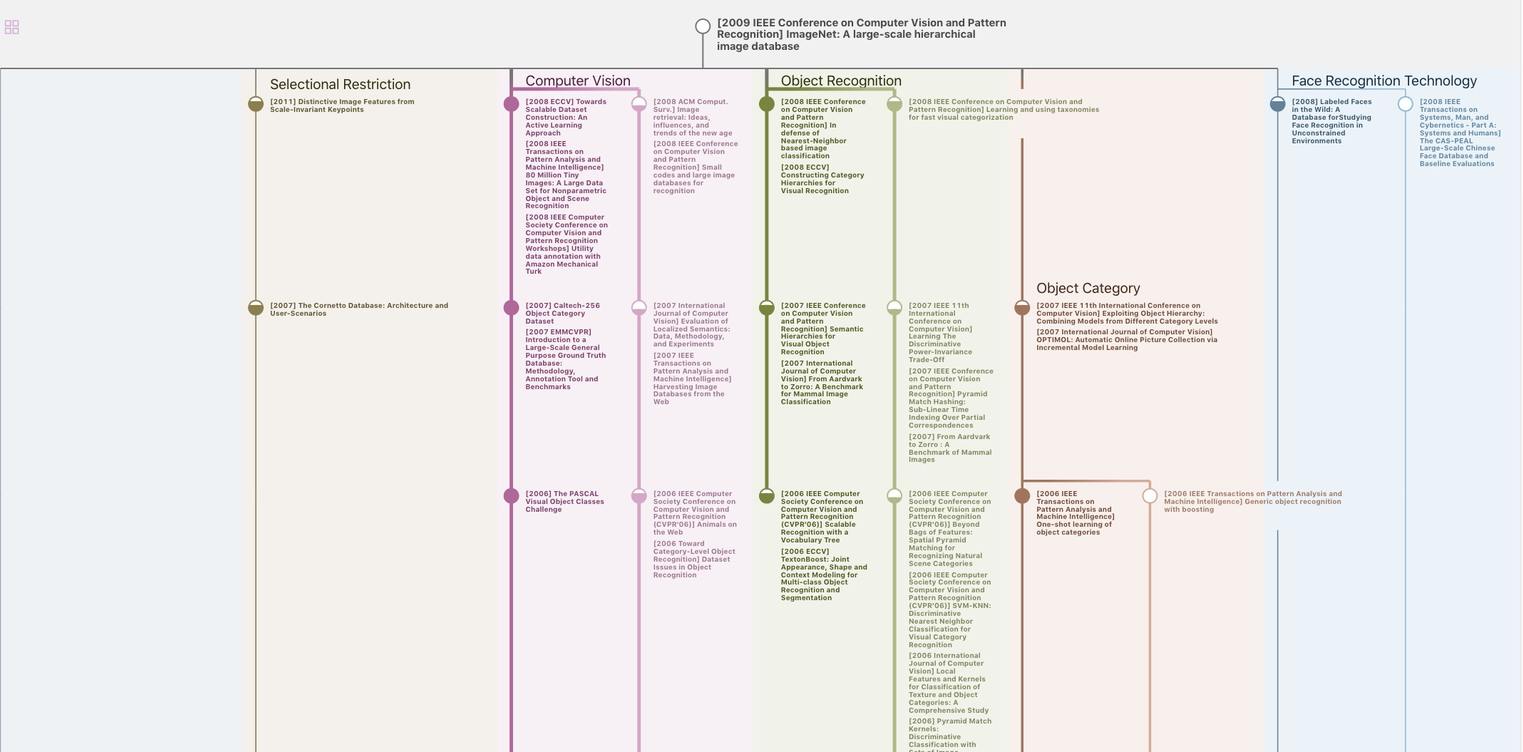
生成溯源树,研究论文发展脉络
Chat Paper
正在生成论文摘要