Fast 3D Indoor Scene Synthesis by Learning Spatial Relation Priors of Objects
IEEE Transactions on Visualization and Computer Graphics(2022)
摘要
We present a framework for fast synthesizing indoor scenes, given a room geometry and a list of objects with learnt priors. Unlike existing data-driven solutions, which often learn priors by co-occurrence analysis and statistical model fitting, our method measures the strengths of spatial relations by tests for complete spatial randomness (CSR), and learns discrete priors based on samples with the ability to accurately represent exact layout patterns. With the learnt priors, our method achieves both acceleration and plausibility by partitioning the input objects into disjoint groups, followed by layout optimization using position-based dynamics (PBD) based on the Hausdorff metric. Experiments show that our framework is capable of measuring more reasonable relations among objects and simultaneously generating varied arrangements in seconds compared with the state-of-the-art works.
更多查看译文
关键词
3D indoor scene synthesis,furniture objects arrangement,complete spatial randomness
AI 理解论文
溯源树
样例
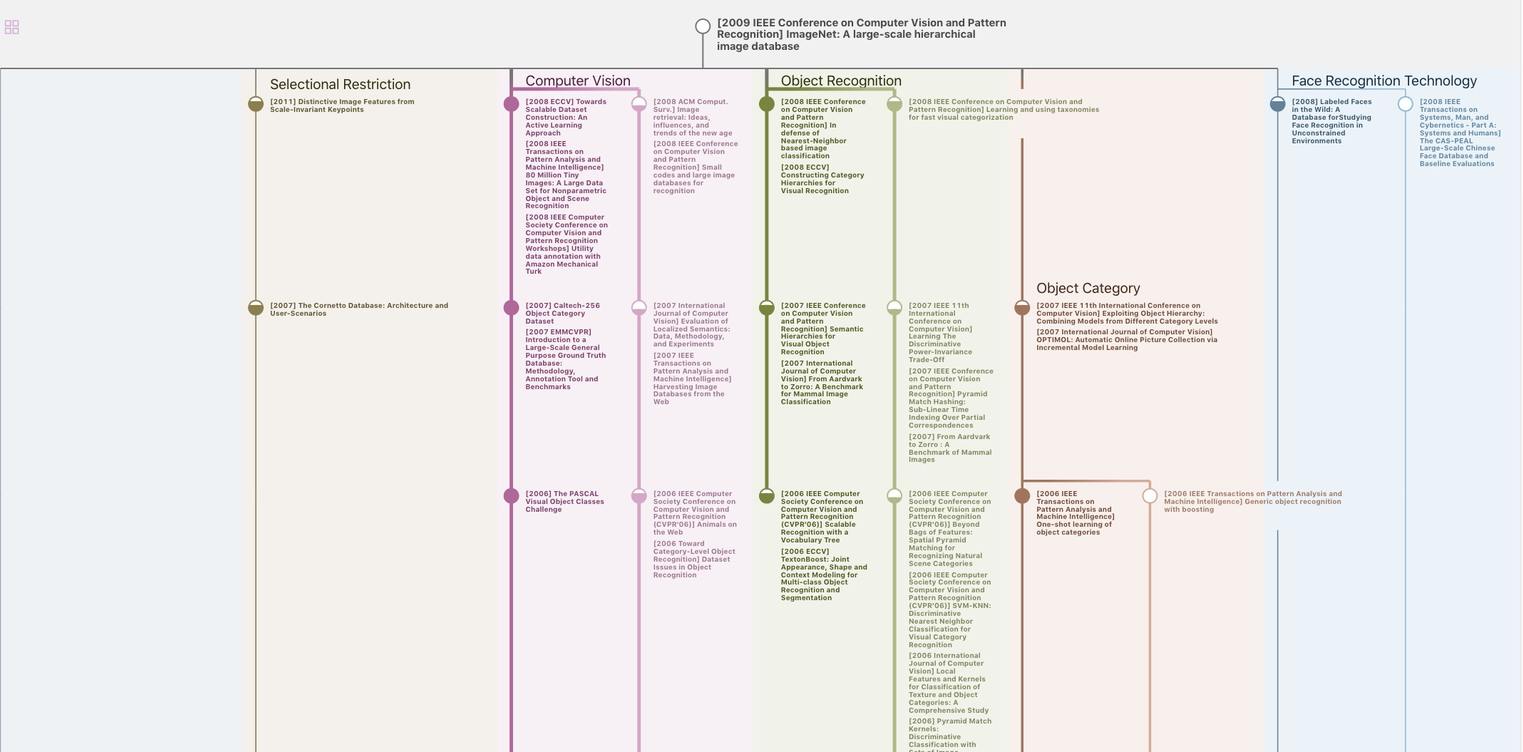
生成溯源树,研究论文发展脉络
Chat Paper
正在生成论文摘要