Explaining the black-box smoothly—A counterfactual approach
Medical Image Analysis(2023)
摘要
We propose a BlackBox Counterfactual Explainer, designed to explain image classification models for medical applications. Classical approaches (e.g., , saliency maps) that assess feature importance do not explain how imaging features in important anatomical regions are relevant to the classification decision. Such reasoning is crucial for transparent decision-making in healthcare applications. Our framework explains the decision for a target class by gradually exaggerating the semantic effect of the class in a query image. We adopted a Generative Adversarial Network (GAN) to generate a progressive set of perturbations to a query image, such that the classification decision changes from its original class to its negation. Our proposed loss function preserves essential details (e.g., support devices) in the generated images.
更多查看译文
关键词
Explainable AI,Interpretable machine learning,Counterfactual reasoning,Chest X-ray diagnosis
AI 理解论文
溯源树
样例
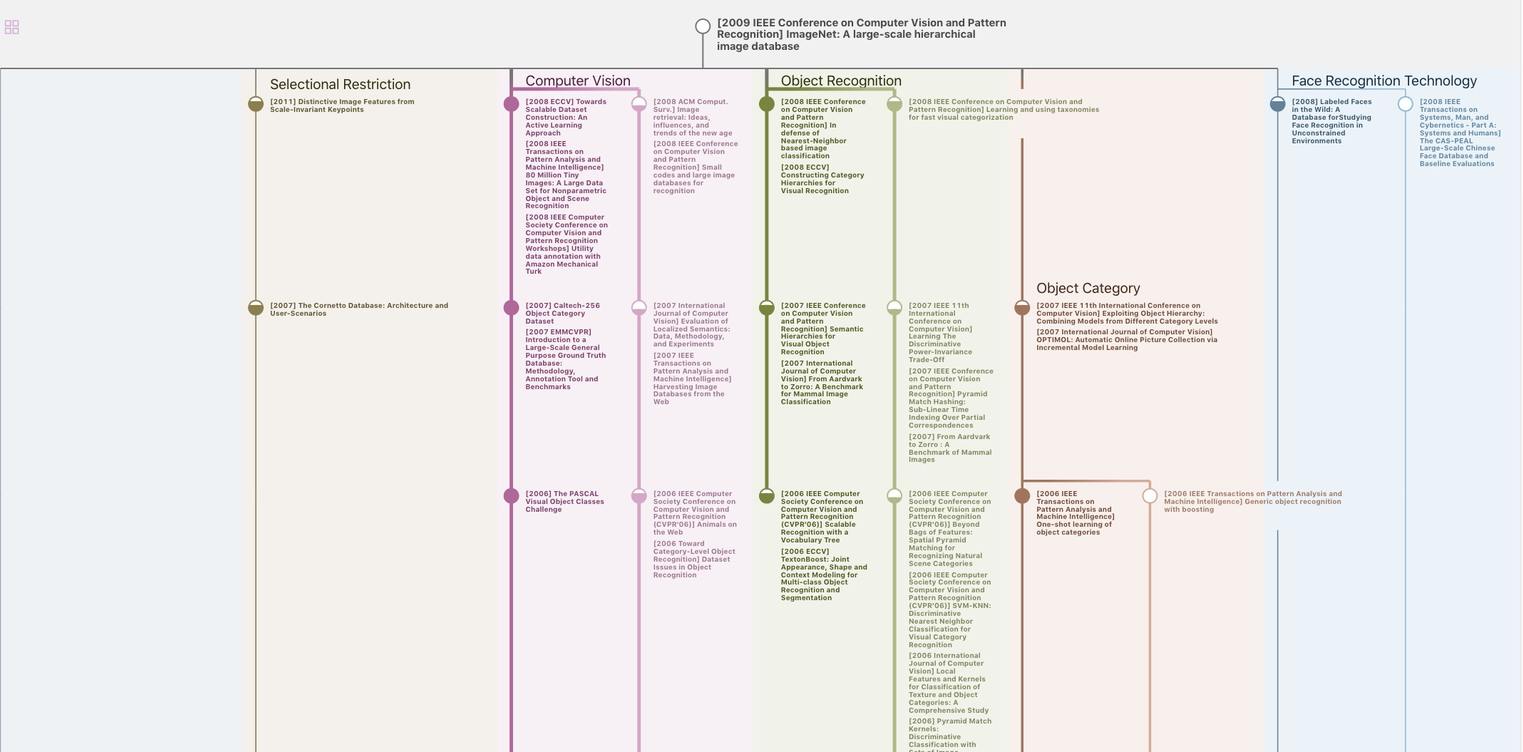
生成溯源树,研究论文发展脉络
Chat Paper
正在生成论文摘要