Variational Embeddings for Community Detection and Node Representation
arxiv(2021)
摘要
In this paper, we study how to simultaneously learn two highly correlated tasks of graph analysis, i.e., community detection and node representation learning. We propose an efficient generative model called VECoDeR for jointly learning Variational Embeddings for Community Detection and node Representation. VECoDeR assumes that every node can be a member of one or more communities. The node embeddings are learned in such a way that connected nodes are not only "closer" to each other but also share similar community assignments. A joint learning framework leverages community-aware node embeddings for better community detection. We demonstrate on several graph datasets that VECoDeR effectively out-performs many competitive baselines on all three tasks i.e. node classification, overlapping community detection and non-overlapping community detection. We also show that VECoDeR is computationally efficient and has quite robust performance with varying hyperparameters.
更多查看译文
关键词
community detection,node representation
AI 理解论文
溯源树
样例
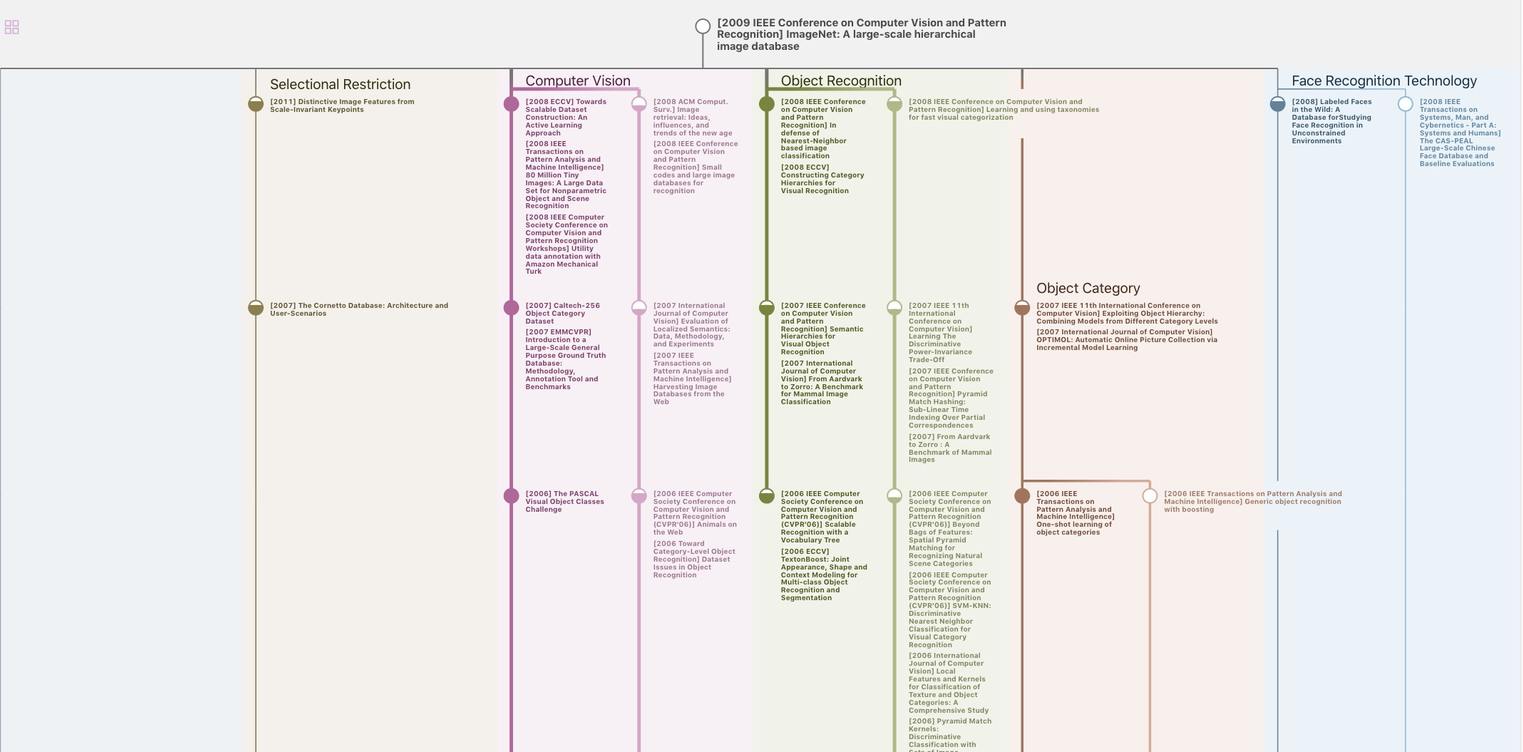
生成溯源树,研究论文发展脉络
Chat Paper
正在生成论文摘要