Explainable Systematic Analysis for Synthetic Aperture Sonar Imagery.
IGARSS(2021)
摘要
In this work, we present an in-depth and systematic analysis using tools such as local interpretable model-agnostic explanations (LIME) (arXiv:1602.04938) and divergence measures to analyze what changes lead to improvement in performance in fine tuned models for synthetic aperture sonar (SAS) data. We examine the sensitivity to factors in the fine tuning process such as class imbalance. Our findings show not only an improvement in seafloor texture classification, but also provide greater insight into what features play critical roles in improving performance as well as a knowledge of the importance of balanced data for fine tuning deep learning models for seafloor classification in SAS imagery.
更多查看译文
关键词
deep learning,transfer learning,SAS,classification,XAI
AI 理解论文
溯源树
样例
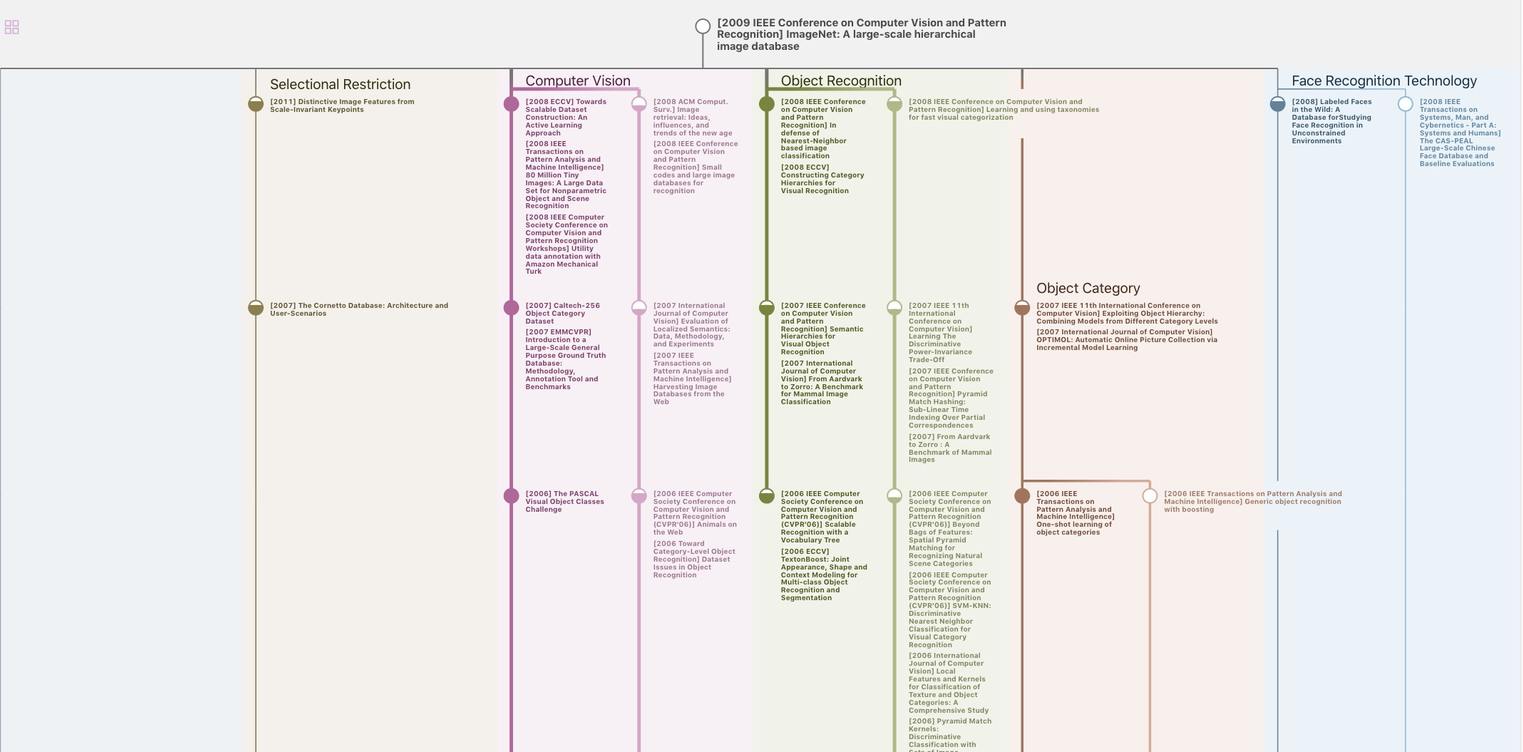
生成溯源树,研究论文发展脉络
Chat Paper
正在生成论文摘要