Spatiotemporal blocking of the bouncy particle sampler for efficient inference in state-space models
arXiv (Cornell University)(2021)
摘要
We propose a novel blocked version of the continuous-time bouncy particle sampler of Bouchard-Côté et al. (J Am Stat Assoc 113(522):855–867, 2018) which is applicable to any differentiable probability density. This alternative implementation is motivated by blocked Gibbs sampling for state-space models (Singh et al. in Biometrika 104(4):953–969, 2017) and leads to significant improvement in terms of effective sample size per second, and furthermore, allows for significant parallelization of the resulting algorithm. The new algorithms are particularly efficient for latent state inference in high-dimensional state-space models, where blocking in both space and time is necessary to avoid degeneracy of MCMC. The efficiency of our blocked bouncy particle sampler, in comparison with both the standard implementation of the bouncy particle sampler and the particle Gibbs algorithm of Andrieu et al. (J R Stat Soc Ser B Stat Methodol 72(3):269–342, 2010), is illustrated numerically for both simulated data and a challenging real-world financial dataset.
更多查看译文
关键词
Markov chain Monte Carlo, Piecewise-deterministic Markov process, Bouncy particle sampler, Particle Gibbs, State-space model
AI 理解论文
溯源树
样例
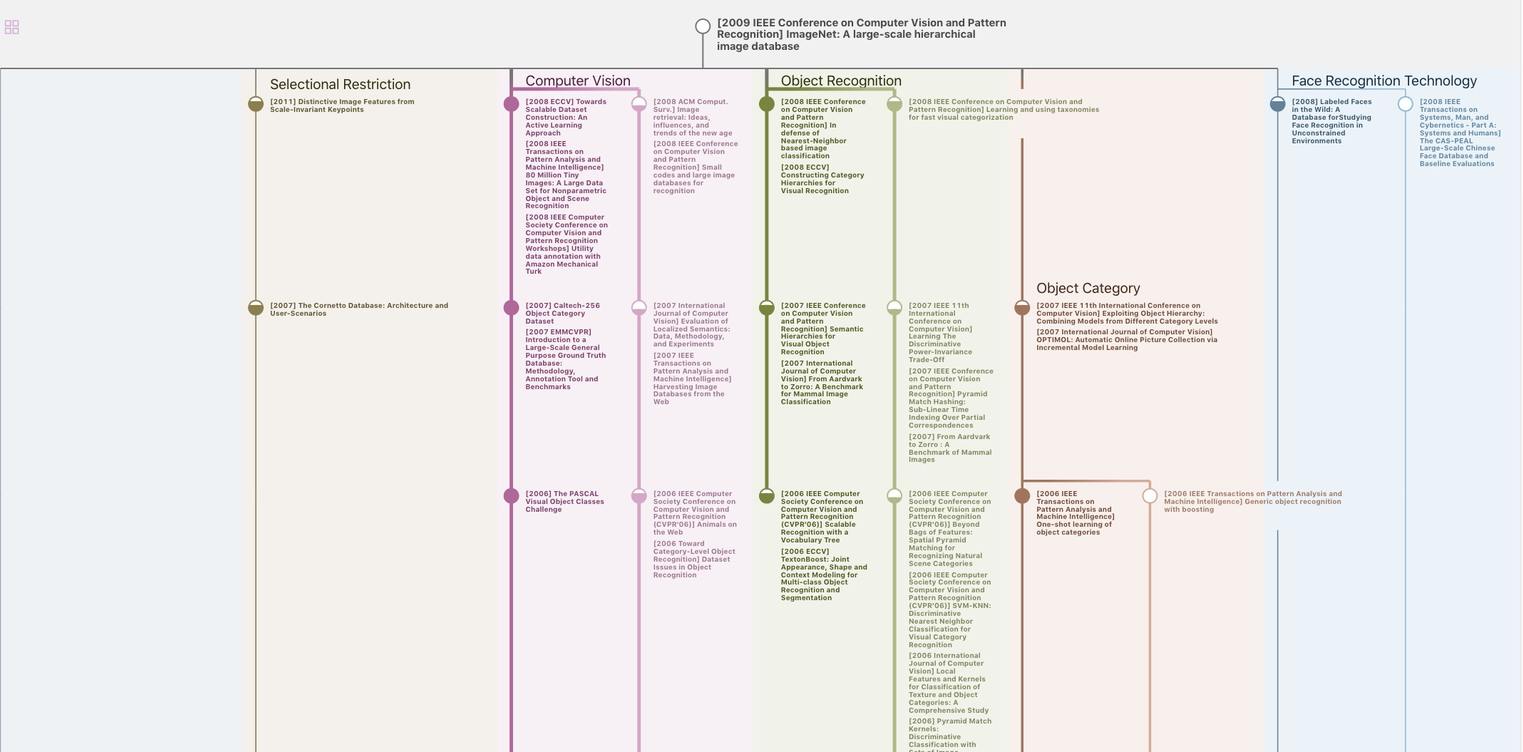
生成溯源树,研究论文发展脉络
Chat Paper
正在生成论文摘要