Machine-Learning-Guided Prediction Models Of Critical Temperature Of Cuprates
JOURNAL OF PHYSICAL CHEMISTRY LETTERS(2021)
摘要
Cuprates have been at the center of long debate regarding their superconducting mechanism; therefore, predicting the critical temperatures of cuprates remains elusive. Herein, using machine learning and firstprinciples calculations, we predict the maximum superconducting transition temperature (T-c,T-max) of hole-doped cuprates and suggest the functional form for T-c,T- max with the root-mean-square-error of 3.705 K and R-2 of 0.969. We have found that the Bader charge of apical oxygen, the bond strength between apical atoms, and the number of superconducting layers are essential to estimate T-c,T-max. Furthermore, we predict the T-c,T-max of hypothetical cuprates generated by replacing apical cations with other elements. Among the hypothetical structures, the cuprates with Ga show the highest predicted T-c,T-max values, which are 71, 117, and 131 K for one, two, and three CuO2 layers, respectively. These findings suggest that machine learning could guide the design of new high-T-c superconductors in the future.
更多查看译文
AI 理解论文
溯源树
样例
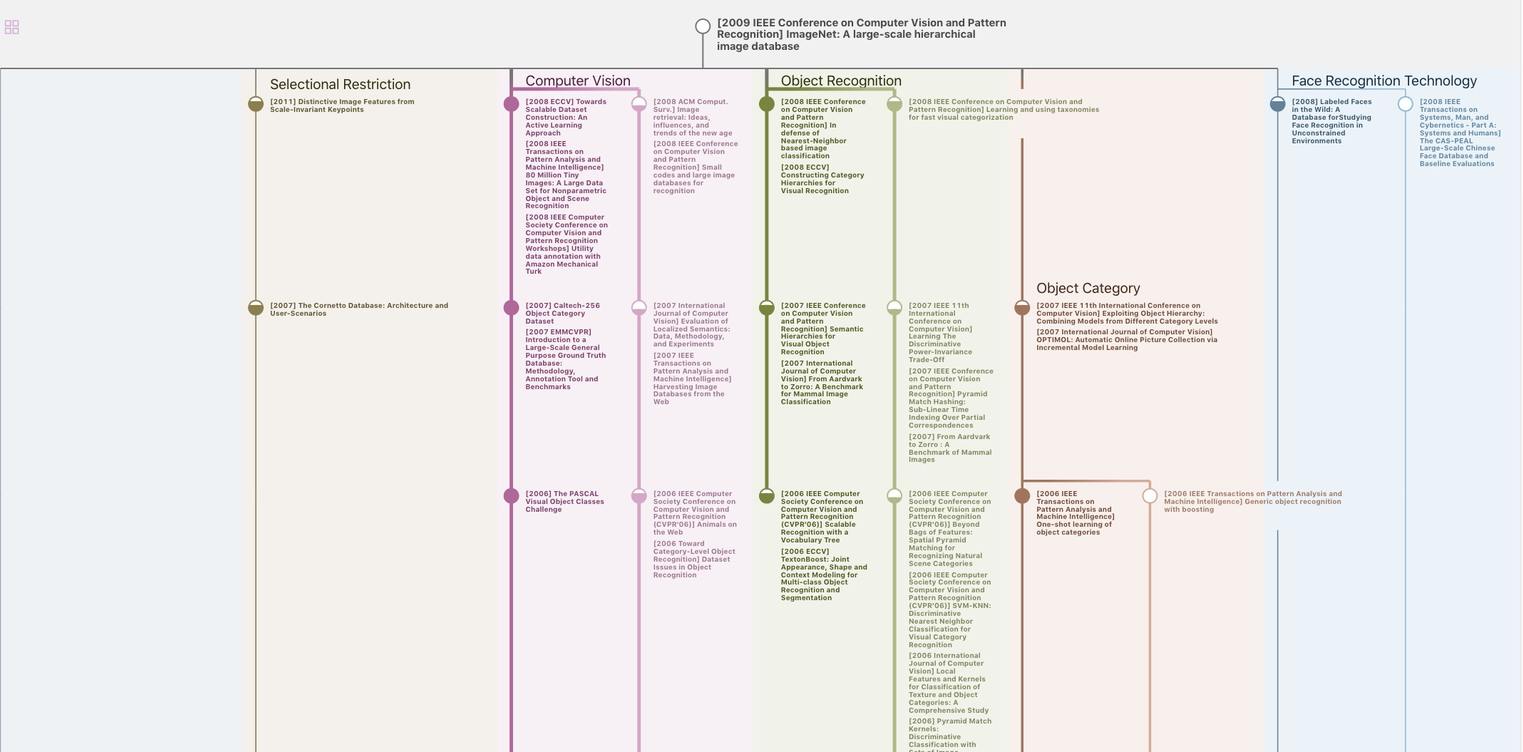
生成溯源树,研究论文发展脉络
Chat Paper
正在生成论文摘要