Single-Image Real-Time Rain Removal Based On Depth-Guided Non-Local Features
IEEE TRANSACTIONS ON IMAGE PROCESSING(2021)
摘要
Rain is a common weather phenomenon that affects environmental monitoring and surveillance systems. According to an established rain model (Garg and Nayar, 2007), the scene visibility in the rain varies with the depth from the camera, where objects faraway are visually blocked more by the fog than by the rain streaks. However, existing datasets and methods for rain removal ignore these physical properties, thus limiting the rain removal efficiency on real photos. In this work, we analyze the visual effects of rain subject to scene depth and formulate a rain imaging model that collectively considers rain streaks and fog. Also, we prepare a dataset called RainCityscapes on real outdoor photos. Furthermore, we design a novel real-time end-to-end deep neural network, for which we train to learn the depth-guided non-local features and to regress a residual map to produce a rain-free output image. We performed various experiments to visually and quantitatively compare our method with several state-of-the-art methods to show its superiority over others.
更多查看译文
关键词
Rain removal, rain formulation, depth-guided, non-local features
AI 理解论文
溯源树
样例
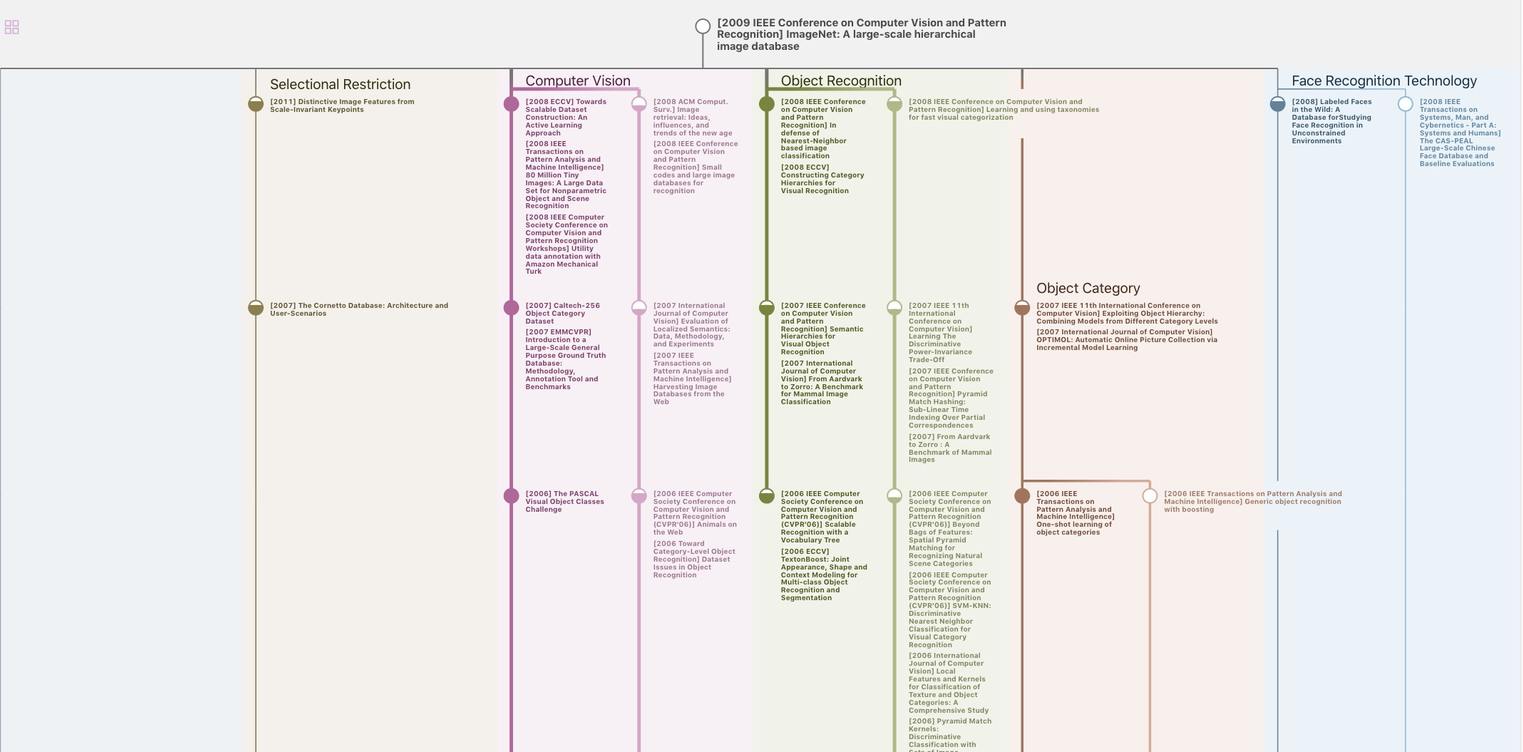
生成溯源树,研究论文发展脉络
Chat Paper
正在生成论文摘要