Efficient Dynamic Analysis of Low-similarity Proteins for Structural Class Prediction
28TH EUROPEAN SIGNAL PROCESSING CONFERENCE (EUSIPCO 2020)(2021)
摘要
Prediction of protein structural classes from amino acid sequences is a challenging problem as it is profitable for analyzing protein function, interactions, and regulation. The majority of existing prediction methods for low-homology sequences utilize numerous amount of features and require an exhausting search for optimal parameter tuning. To address this problem, this work proposes a novel self-tuned architecture for feature extraction by modeling directly the inherent dynamics of the data in higher-dimensional phase space via chaos game representation (CGR) and generalized multidimensional recurrence quantification analysis (GmdRQA). Experimental evaluation on a real benchmark dataset demonstrates the superiority of the herein proposed architecture when compared against the state-of-the-art unidimensional RQA taking under consideration that our method achieves similar performance in a data-driven manner with a smaller computational cost.
更多查看译文
关键词
Protein structure prediction, chaos game representation, multidimensional recurrence quantification analysis, nonlinear time series analysis
AI 理解论文
溯源树
样例
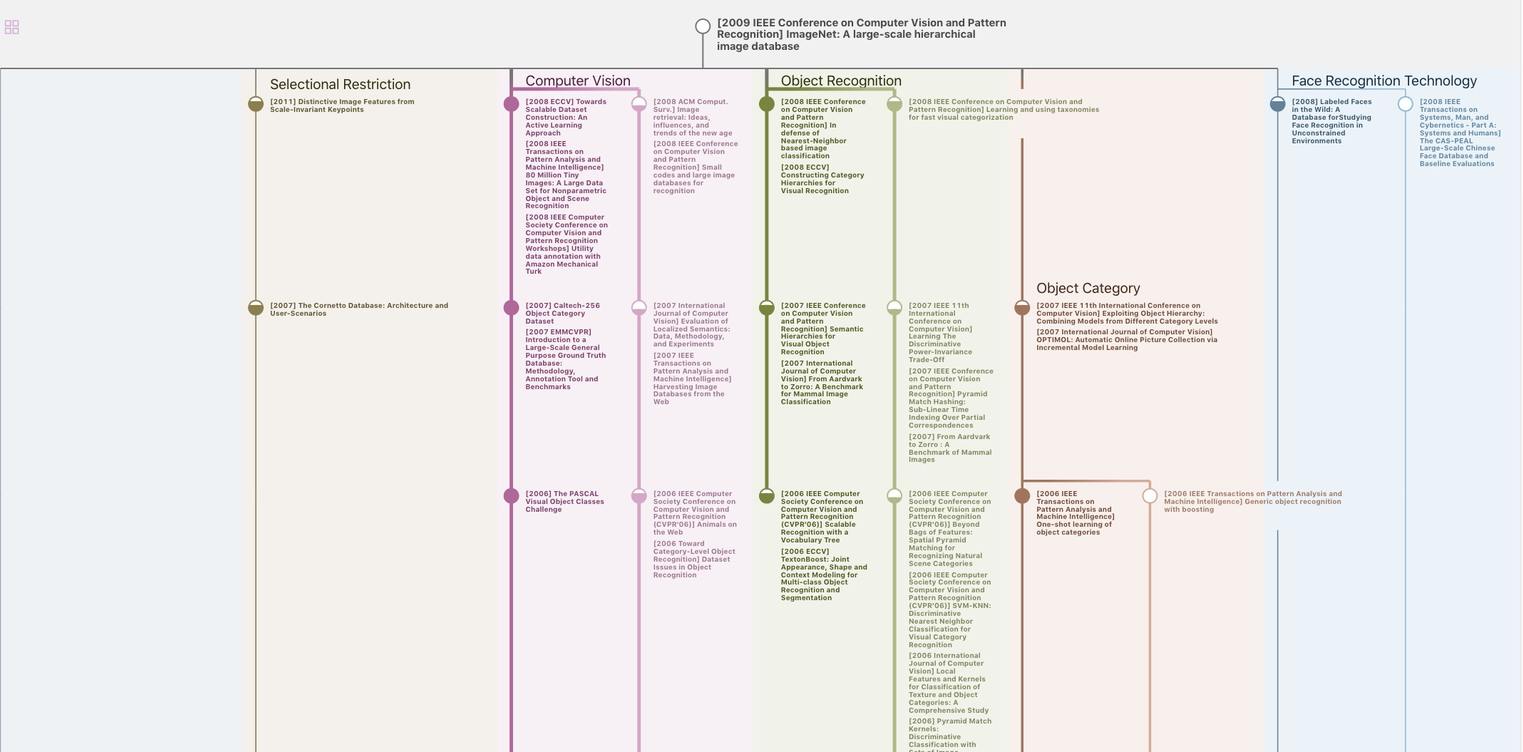
生成溯源树,研究论文发展脉络
Chat Paper
正在生成论文摘要