Group Nonnegative Matrix Factorization With Sparse Regularization In Multi-Set Data
28TH EUROPEAN SIGNAL PROCESSING CONFERENCE (EUSIPCO 2020)(2021)
摘要
Constrained joint analysis of data from multiple sources has received widespread attention for that it allows us to explore potential connections and extract meaningful hidden components. In this paper, we formulate a flexible joint source separation model termed as group nonnegative matrix factorization with sparse regularization (GNMF-SR), which aims to jointly analyze the partially coupled multi-set data. In the GNMF-SR model, common and individual patterns of particular underlying factors can be extracted simultaneously with imposing nonnegative constraint and sparse penalty. Alternating optimization and alternating direction method of multipliers (ADMM) are combined to solve the GNMF-SR model. Using the experiment of simulated fMRI-like data, we demonstrate the ADMM-based GNMF-SR algorithm can achieve the better performance.
更多查看译文
关键词
Alternating direction method of multipliers, coupled, group nonnegative matrix factorization, joint analysis, sparse representation
AI 理解论文
溯源树
样例
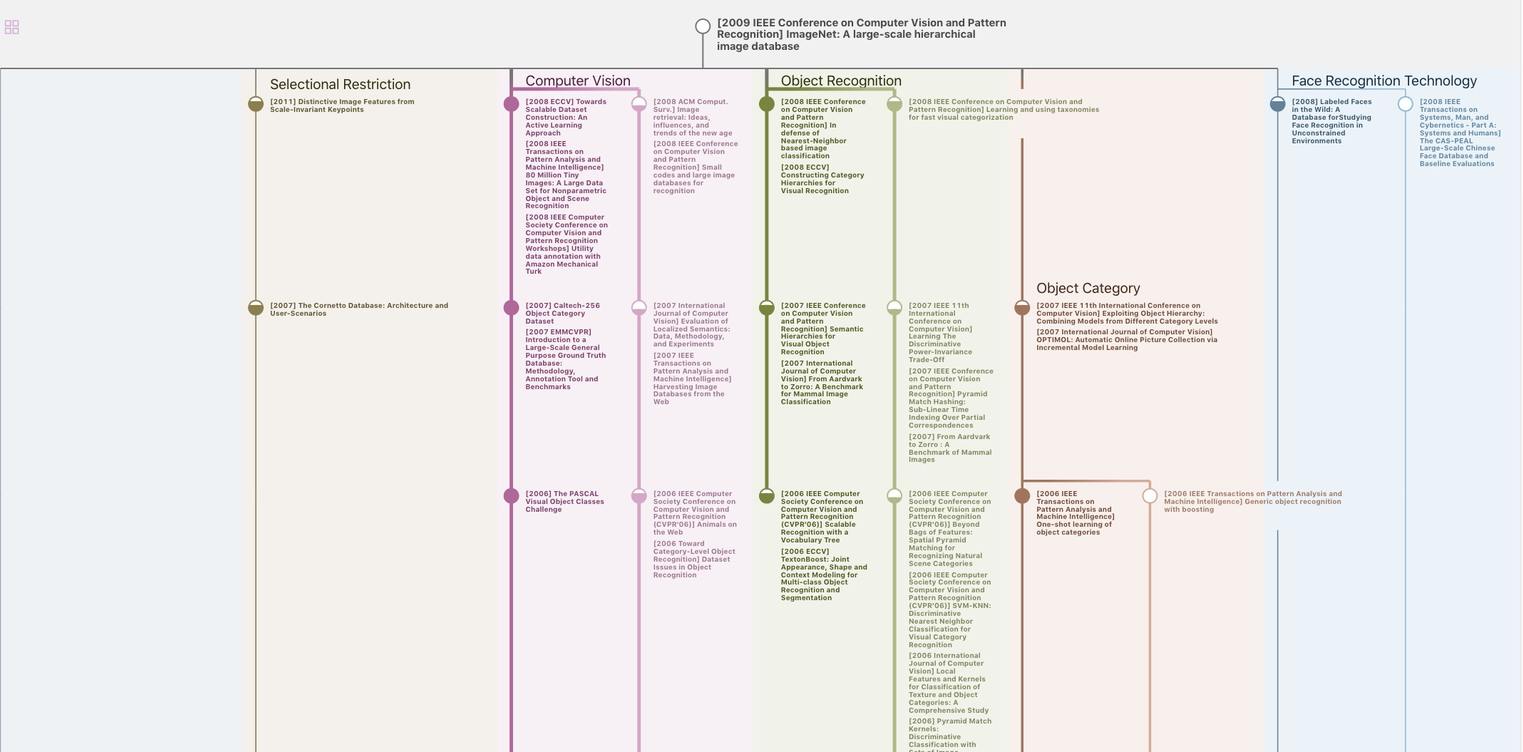
生成溯源树,研究论文发展脉络
Chat Paper
正在生成论文摘要