Variational Auto-Encoder-Based Detection Of Electricity Stealth Cyber-Attacks In Ami Networks
28TH EUROPEAN SIGNAL PROCESSING CONFERENCE (EUSIPCO 2020)(2021)
摘要
Current efforts to detect electricity theft cyber-attacks in advanced metering infrastructures (AMIs) are hindered by the lack of malicious electricity theft datasets. Therefore, anomaly detectors trained with the energy consumption profiles of honest customers appear as a plausible solution to overcome the lack of malicious datasets. Taking into account this constraint, this paper examines the performance of two structures of variational auto-encoders (VAEs); fully-connected (FC) VAE and long-short-term-memory (LSTM) VAE in detecting electricity thefts. The proposed structures are promising and exhibit an improvement of 11 - 15% in detection rate, 9 - 22% in false alarm rate, and 27 - 37% in the highest difference compared to existing state-of-the-art anomaly detectors that are shallow and static, such as single-class support vector machine (SVM) and auto-regressive integrated moving average (ARIMA) models.
更多查看译文
关键词
electricity theft, auto-encoders, deep learning
AI 理解论文
溯源树
样例
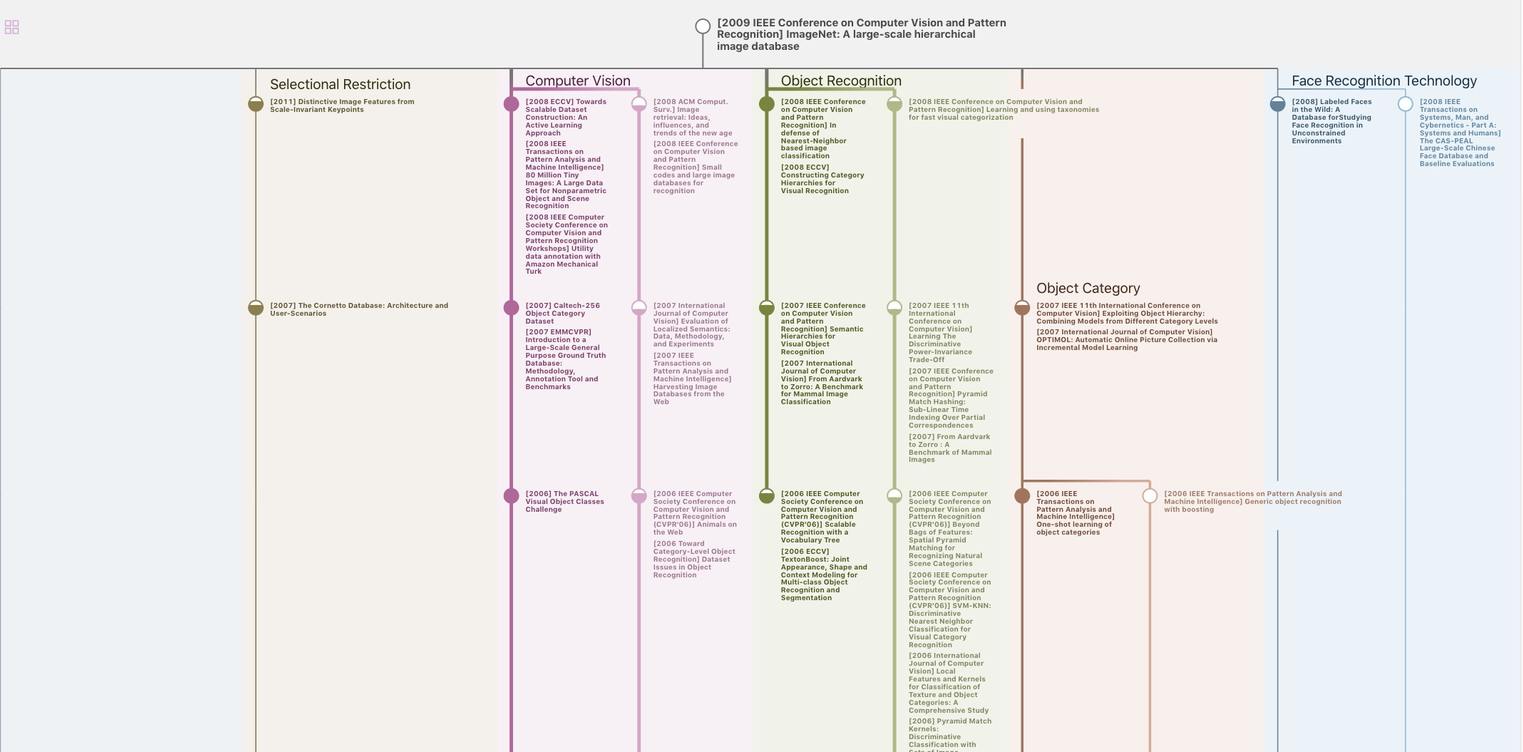
生成溯源树,研究论文发展脉络
Chat Paper
正在生成论文摘要