Wavenet Based Architectures For Denoising Periodic Discontinuous Signals And Application To Friction Signals
28TH EUROPEAN SIGNAL PROCESSING CONFERENCE (EUSIPCO 2020)(2021)
摘要
In this paper, we introduce a deep learning model based on Wavenet to denoise periodic signals containing some strong discontinuities, where the dataset used for training contains only synthetic data. We introduce a new cost function using a total variation term. The synthetic data which contain strong discontinuities, are generated as the sum of a sine wave, a square signal and a white gaussian noise. This simple model is very time-efficient to compute and allows us to perform data generation for each training of the architecture instead of physically storing the dataset. We specifically apply this model to real friction signals obtained through a rotating tribological system. We also compared our method with an improved TV denoising algorithm.
更多查看译文
关键词
Denoising, Wavenet, Total variation, Synthetic data, Friction signals
AI 理解论文
溯源树
样例
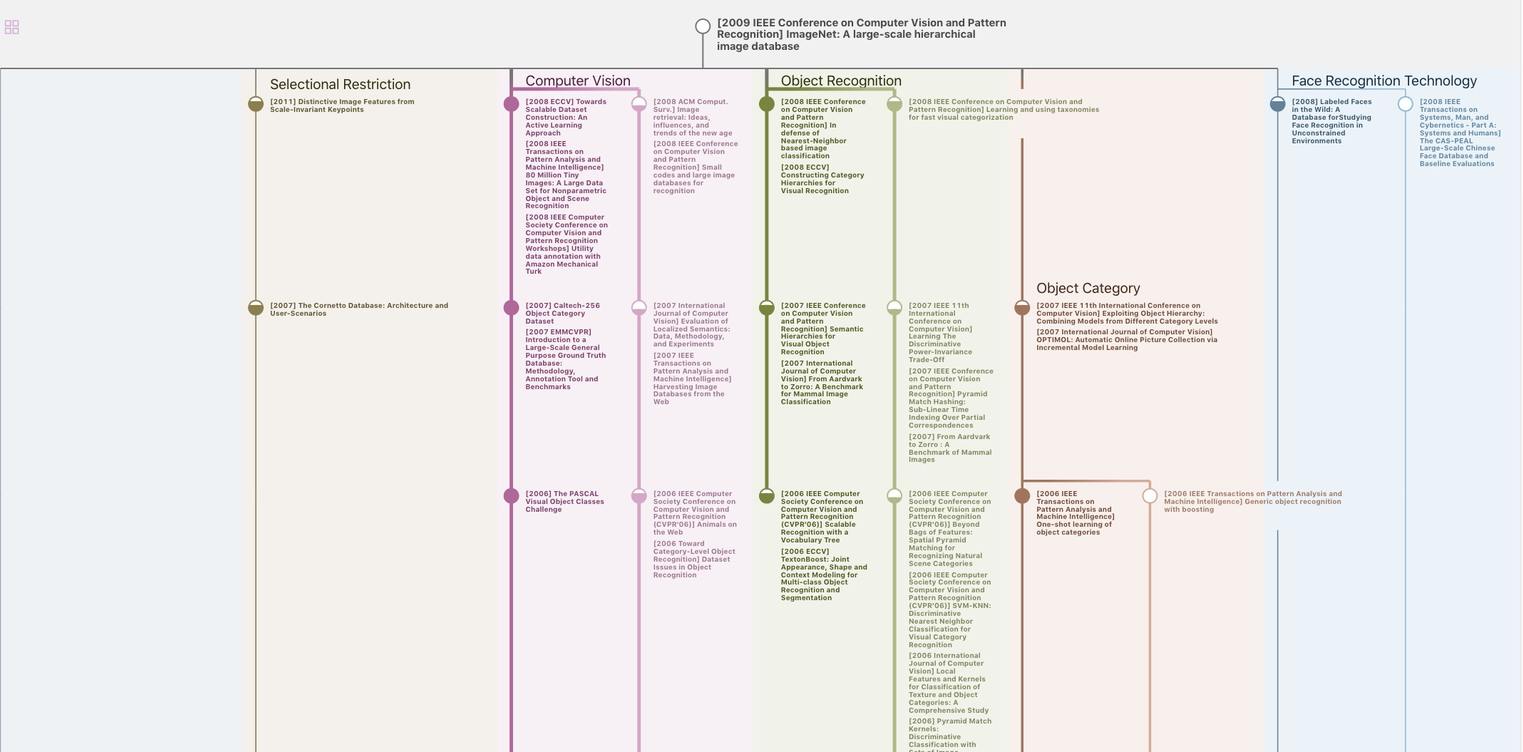
生成溯源树,研究论文发展脉络
Chat Paper
正在生成论文摘要