A Graph Signal Processing Framework For The Classification Of Temporal Brain Data
28TH EUROPEAN SIGNAL PROCESSING CONFERENCE (EUSIPCO 2020)(2021)
摘要
Graph Signal Processing (GSP) addresses the analysis of data living on an irregular domain which can be modeled with a graph. This capability is of great interest for the study of brain connectomes. In this case, data lying on the nodes of the graph are considered as signals (e.g., fMRI time-series) that have a strong dependency on the graph topology (e.g., brain structural connectivity). In this paper, we adopt GSP tools to build features related to the frequency content of the signals. To make these features highly discriminative, we apply an extension of the Fukunaga-Koontz transform. We then use these new features to train a decision tree for the prediction of autism spectrum disorder. Interestingly, our framework outperforms state-of-the-art methods on the publicly available ABIDE dataset.
更多查看译文
关键词
Graph signal processing, machine learning, explainability, decision trees, functional MRI, autism spectrum disorder
AI 理解论文
溯源树
样例
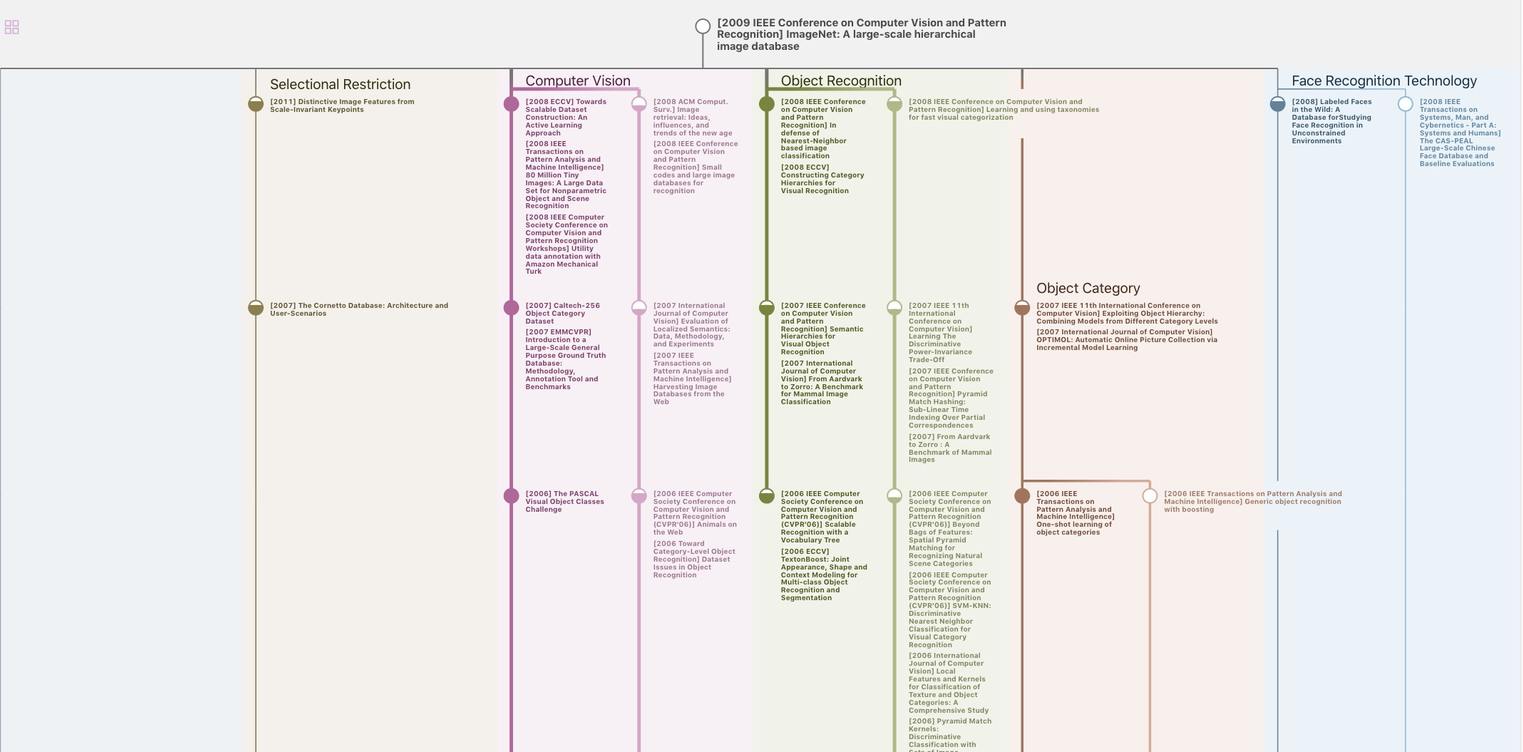
生成溯源树,研究论文发展脉络
Chat Paper
正在生成论文摘要