Convolutional Neural Network For Material Decomposition In Spectral Ct Scans
28TH EUROPEAN SIGNAL PROCESSING CONFERENCE (EUSIPCO 2020)(2021)
摘要
Spectral computed tomography acquires energy-resolved data that allows recovery of densities of constituents of an object. This can be achieved by decomposing the measured spectral projection into material projections, and passing these decomposed projections through a tomographic reconstruction algorithm, to get the volumetric mass density of each material. Material decomposition is a nonlinear inverse problem that has been traditionally solved using model-based material decomposition algorithms. However, the forward model is difficult to estimate in real prototypes. Moreover, the traditional regularizers used to stabilized inversions are not fully relevant in the projection domain.In this study, we propose a deep-learning method for material decomposition in the projection domain. We validate our methodology with numerical phantoms of human knees that are created from synchrotron CT scans. We consider four different scans for training, and one for validation. The measurements are corrupted by Poisson noise, assuming that at most 105 photons hit the detector. Compared to a regularized Gauss-Newton algorithm, the proposed deep-learning approach provides a compromise between noise and resolution, which reduces the computation time by a factor of 100.
更多查看译文
关键词
Spectral computed tomography, Convolutional neural network, Material decomposition, Knee phantom
AI 理解论文
溯源树
样例
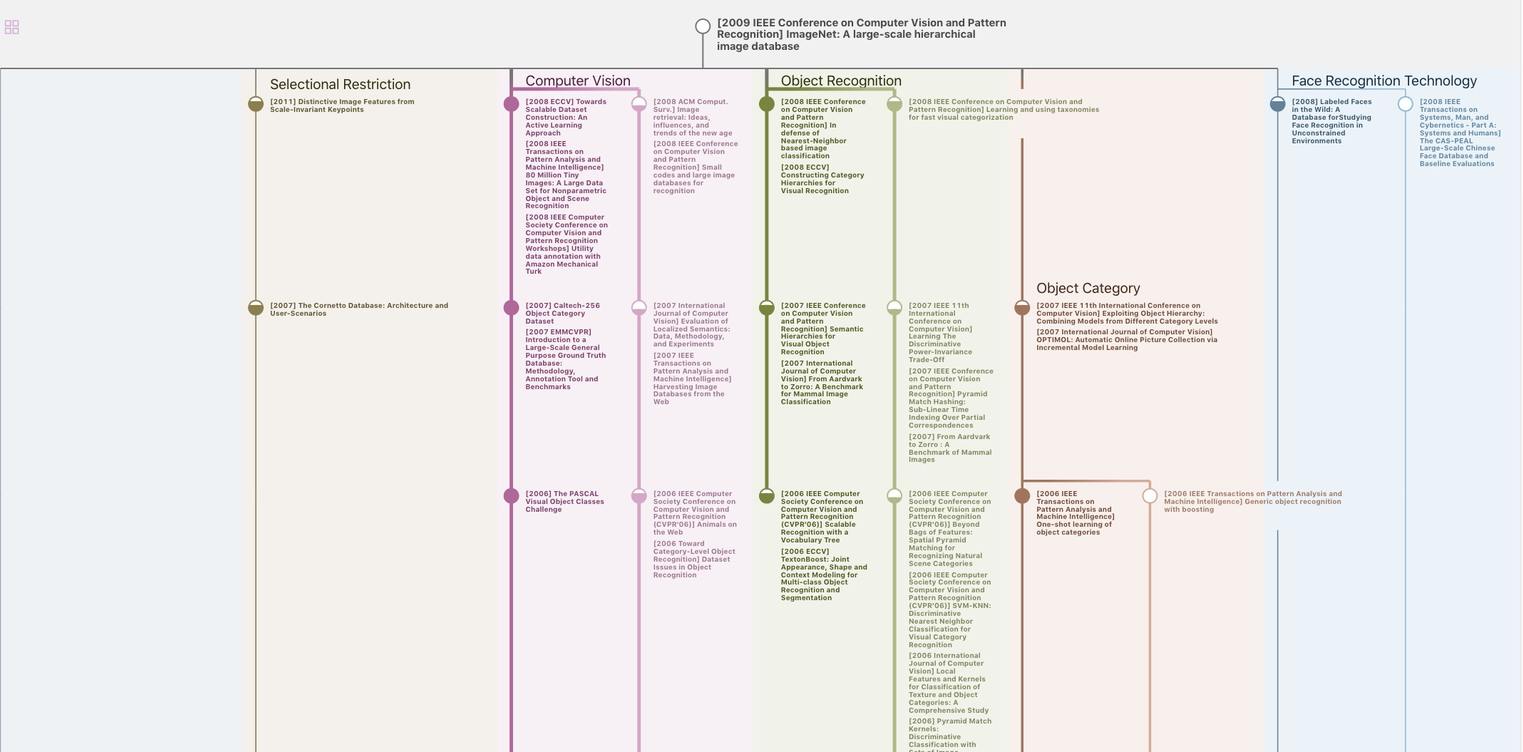
生成溯源树,研究论文发展脉络
Chat Paper
正在生成论文摘要