A Residual U-Net Network With Image Prior For 3d Image Denoising
28TH EUROPEAN SIGNAL PROCESSING CONFERENCE (EUSIPCO 2020)(2021)
摘要
Denoising algorithms via sparse representation are among the state-of-the art for image restoration. On previous work, we proposed SPADE - a sparse- and prior-based method for 3D-image denoising. In this work, we extend this idea to learning approaches and propose a novel residual-U-Net prior-based (ResPrU-Net) method that exploits a prior image. The proposed ResPrU-Net architecture has two inputs, the noisy image and the prior image, and a residual connection that connects the prior image to the output of the network. We compare ResPrU-Net to U-Net and SPADE on human knee data acquired on a spectral computerized tomography scanner. The prior image is built from the noisy image by combining information from neighbor slices and it is the same for both SPADE and ResPrU-Net. For deep learning approaches, we use four knee samples and data augmentation for training, one knee for validation and two for test. Results show that for high noise, U-Net leads to worst results, with images that are excessively blurred. Prior-based methods, SPADE and ResPrU-Net, outperformed U-Net, leading to restored images that present similar image quality than the target. ResPrU-Net provides slightly better results than SPADE. For low noise, methods present similar results.
更多查看译文
关键词
Image denoising, deep learning, U-Net
AI 理解论文
溯源树
样例
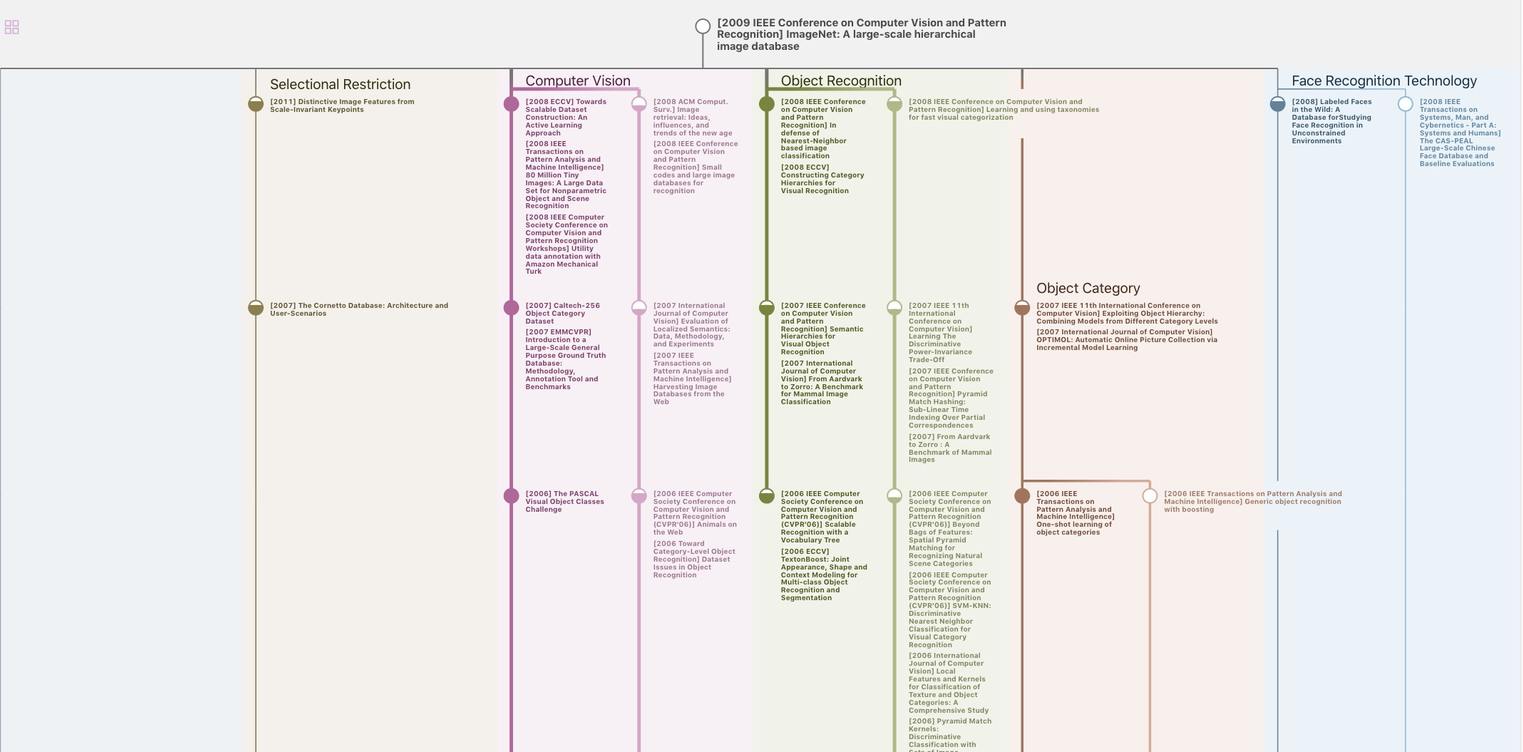
生成溯源树,研究论文发展脉络
Chat Paper
正在生成论文摘要