Accelerating Geostatistical Modeling Using Geostatistics-Informed Machine Learning
COMPUTERS & GEOSCIENCES(2021)
摘要
Ordinary Kriging (OK) is a popular geostatistical algorithm for spatial interpolation and estimation. The computational complexity of OK changes quadratically and cubically for memory and speed, respectively, given the number of data. Therefore, it is computationally intensive and also challenging to process a large set of data, especially in three-dimensional (3D) cases. This paper develops a geostatistics-informed machine learning (GIML) model to improve the efficiency of OK by reducing the number of points required to be estimated using OK. Specifically, only a very few of the unknown points are estimated by OK to get the weights and estimations, which are used as the training dataset. Moreover, the governing equations of OK are used to guide our proposed machine learning to better reproduce the spatial distributions. Our results show that the proposed GIML can reduce the computational time of OK by at least one order of magnitude. The effectiveness of the GIML is evaluated and compared using a 2D case. Furthermore, we demonstrate its efficiency and robustness by considering a different number of training samples on various 3D simulation grids.
更多查看译文
关键词
Artificial intelligence, Geosystems, Groundwater, Estimation, Statistical modeling
AI 理解论文
溯源树
样例
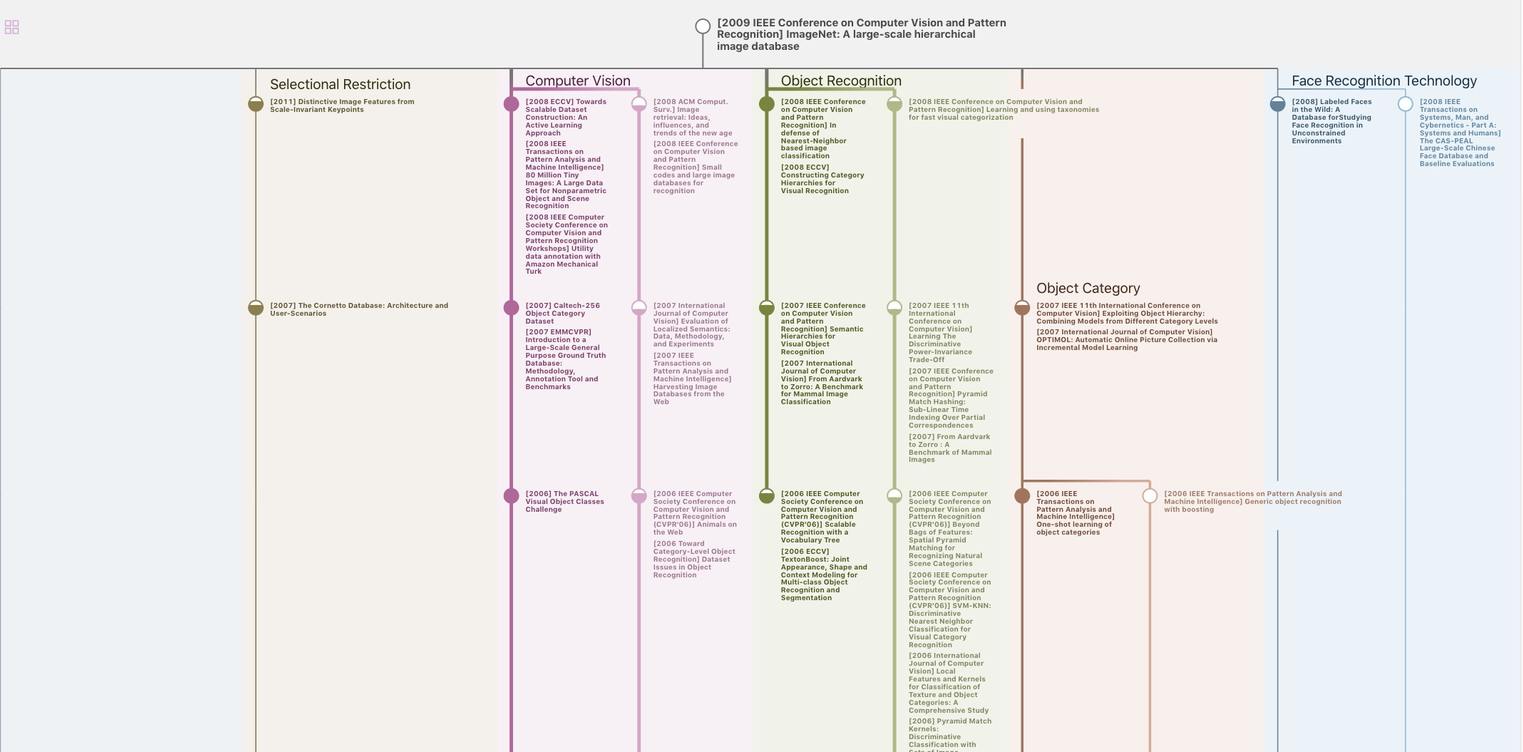
生成溯源树,研究论文发展脉络
Chat Paper
正在生成论文摘要