A New Hybrid Possibilistic-Probabilistic Decision-Making Scheme For Classification
ENTROPY(2021)
摘要
Uncertainty is at the heart of decision-making processes in most real-world applications. Uncertainty can be broadly categorized into two types: aleatory and epistemic. Aleatory uncertainty describes the variability in the physical system where sensors provide information (hard) of a probabilistic type. Epistemic uncertainty appears when the information is incomplete or vague such as judgments or human expert appreciations in linguistic form. Linguistic information (soft) typically introduces a possibilistic type of uncertainty. This paper is concerned with the problem of classification where the available information, concerning the observed features, may be of a probabilistic nature for some features, and of a possibilistic nature for some others. In this configuration, most encountered studies transform one of the two information types into the other form, and then apply either classical Bayesian-based or possibilistic-based decision-making criteria. In this paper, a new hybrid decision-making scheme is proposed for classification when hard and soft information sources are present. A new Possibilistic Maximum Likelihood (PML) criterion is introduced to improve classification rates compared to a classical approach using only information from hard sources. The proposed PML allows to jointly exploit both probabilistic and possibilistic sources within the same probabilistic decision-making framework, without imposing to convert the possibilistic sources into probabilistic ones, and vice versa.
更多查看译文
关键词
possibility theory, possibilistic decision rule, possibilistic maximum likelihood, pattern classification, uncertainty, Bayesian decision, maximum a posteriori, image processing
AI 理解论文
溯源树
样例
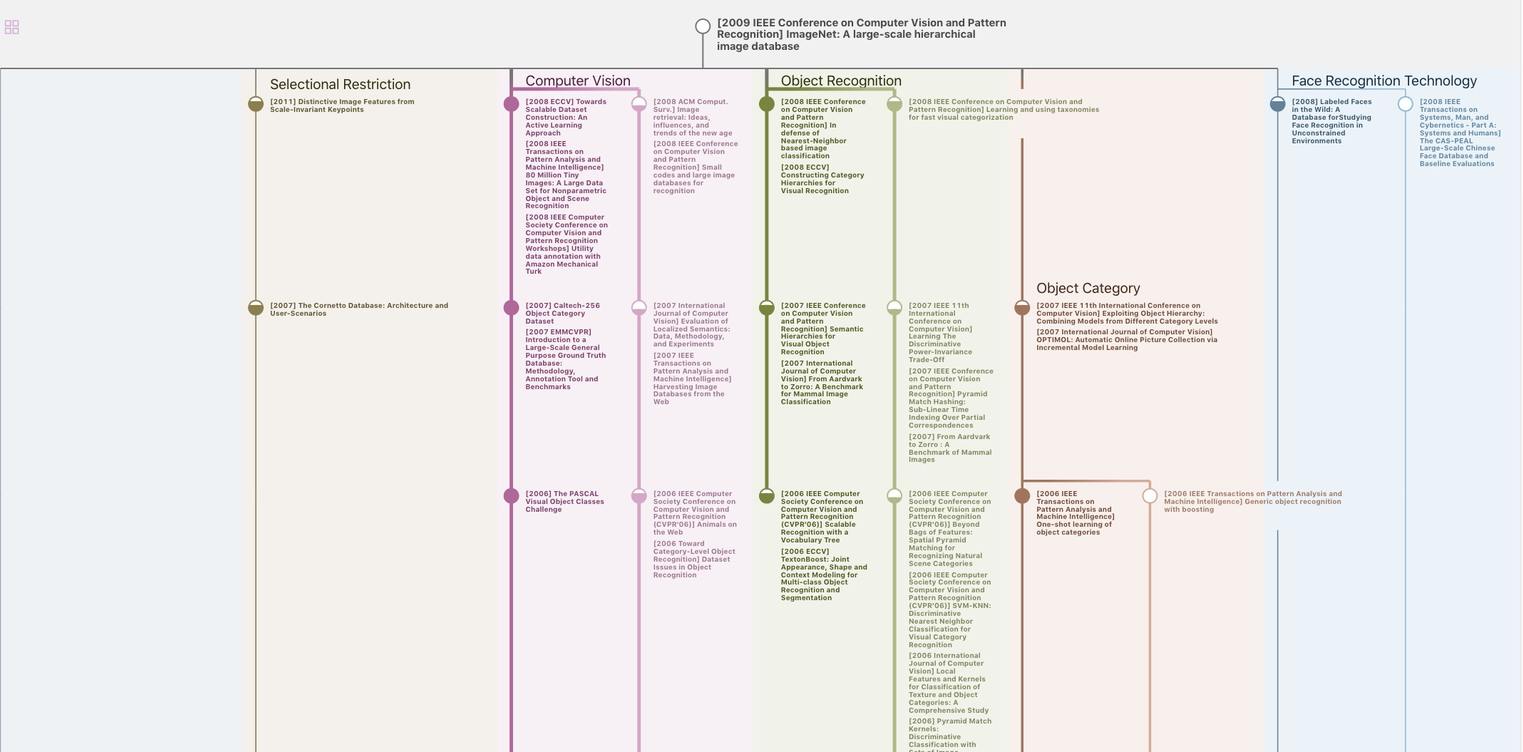
生成溯源树,研究论文发展脉络
Chat Paper
正在生成论文摘要