A Cluster-Based Under-Sampling Algorithm for Class-Imbalanced Data.
HAIS(2020)
摘要
The resampling methods are among the most popular strategies to face the class imbalance problem. The objective of these methods is to compensate the imbalanced class distribution by over-sampling the minority class and/or under-sampling the majority class. In this paper, a new under-sampling method based on the DBSCAN clustering algorithm is introduced. The main idea is to remove the majority class instances that are identified as noise by DBSCAN. The proposed method is empirically compared to well-known state-of-the-art under-sampling algorithms over 25 benchmarking databases and the experimental results demonstrate the effectiveness of the new method in terms of sensitivity, specificity, and geometric mean of individual accuracies.
更多查看译文
关键词
cluster-based,under-sampling,class-imbalanced
AI 理解论文
溯源树
样例
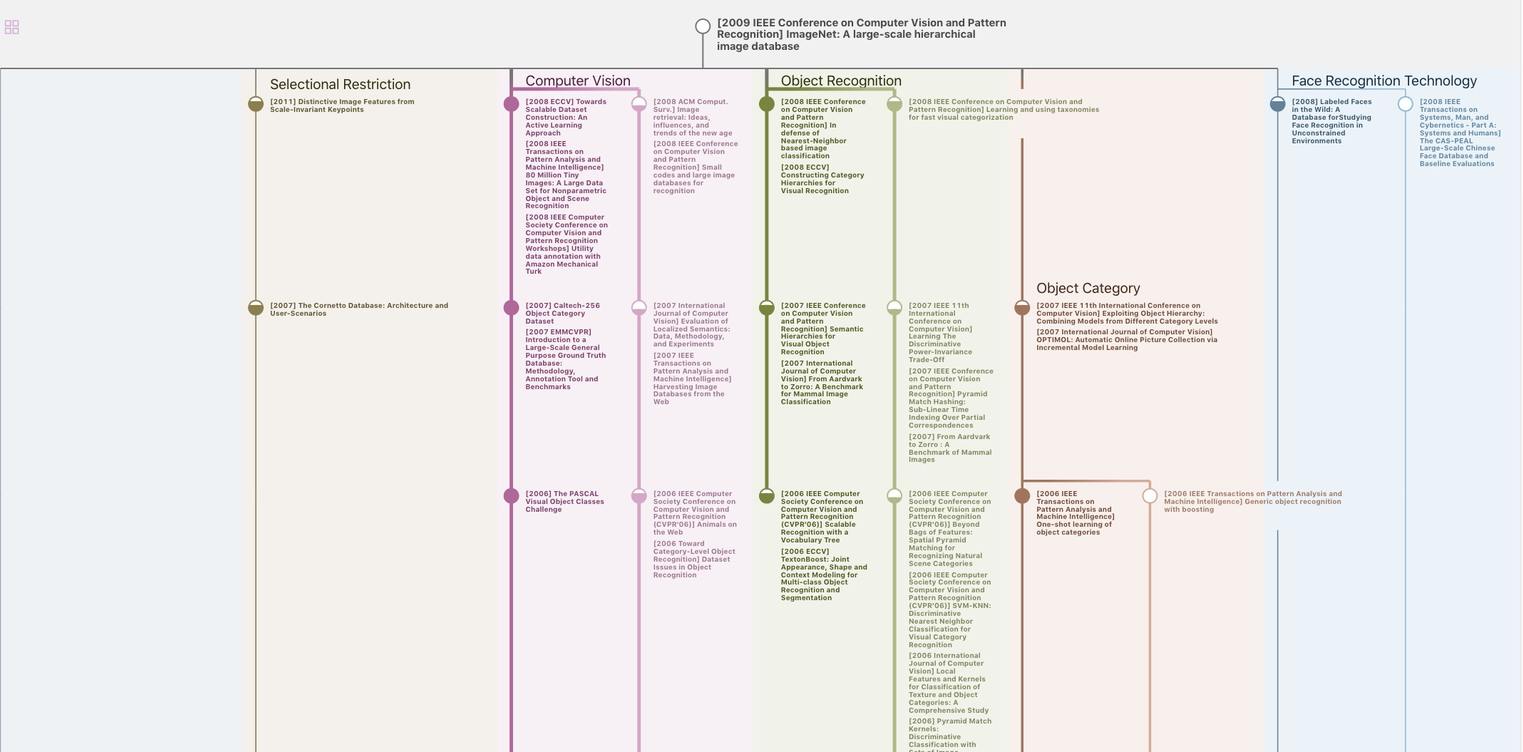
生成溯源树,研究论文发展脉络
Chat Paper
正在生成论文摘要