DeepWind: An Accurate Wind Turbine Condition Monitoring Framework via Deep Learning on Embedded Platforms
2020 25TH IEEE INTERNATIONAL CONFERENCE ON EMERGING TECHNOLOGIES AND FACTORY AUTOMATION (ETFA)(2020)
摘要
Condition monitoring of critical components in wind turbines is of utmost importance to enable low cost and preventive maintenance and to minimize downtime. In this paper we propose DEEPWIND, an end-to-end condition monitoring and fault detection framework that can be implemented on resource-constrained embedded platforms. DEEPWIND exploits multi-channel convolutional neural networks to automatically extract features from sensor data without any need of human feature engineering, and utilizes the features to classify faults occurring in rotor blades of wind turbines. Experiments on a real-world dataset provided by Weidmüller Monitoring Systems GmbH reveal an average F1-score of 0.94 and thus underline the suitability of the approach for fault detection in wind turbines.
更多查看译文
关键词
Wind turbine, Condition monitoring, Deep learning, Embedded systems, Fault, Anomaly detection, Precision scaling, Approximate computing
AI 理解论文
溯源树
样例
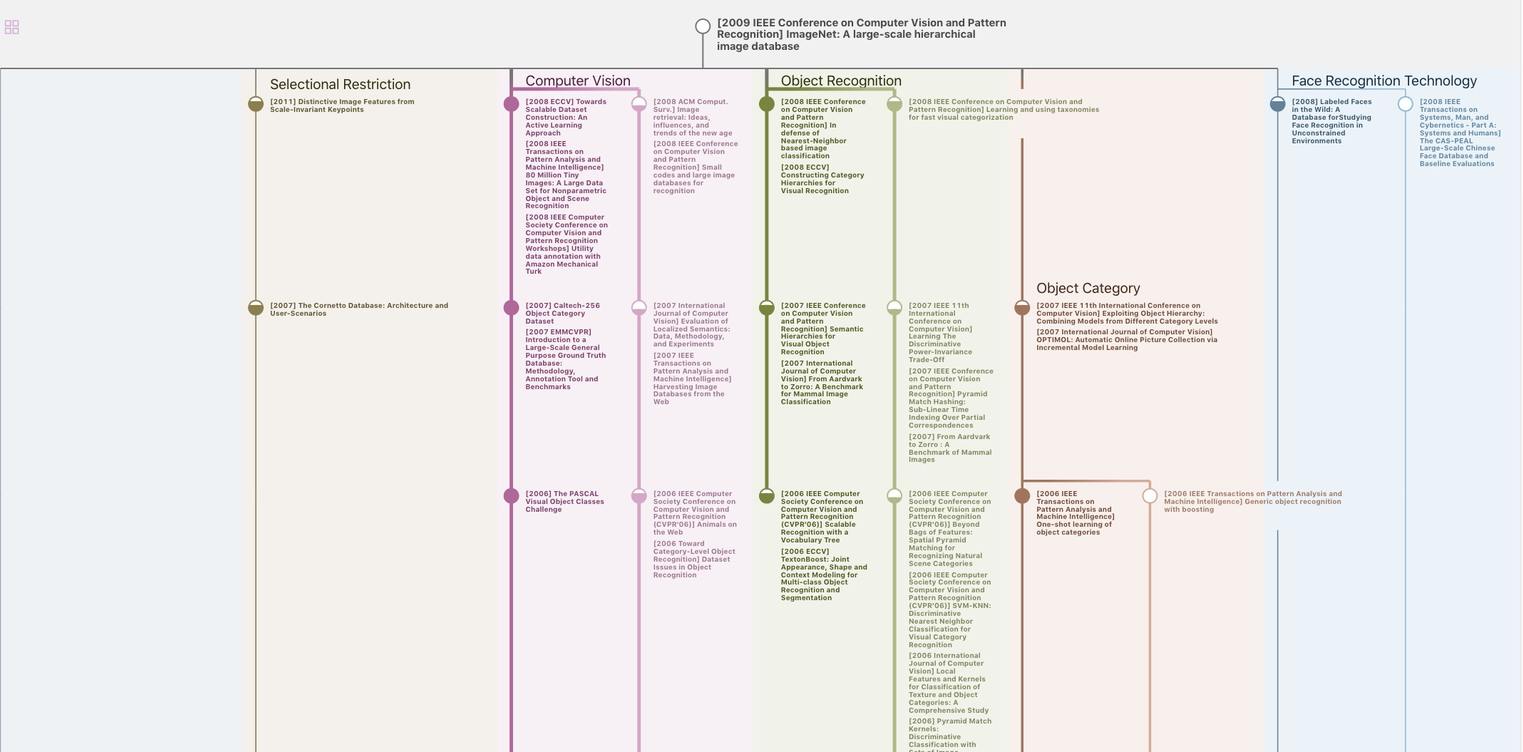
生成溯源树,研究论文发展脉络
Chat Paper
正在生成论文摘要