Faulty Requirements Made Valuable: On the Role of Data Quality in Deep Learning
2020 IEEE Seventh International Workshop on Artificial Intelligence for Requirements Engineering (AIRE)(2020)
摘要
Large collections of data help evolve deep learning into the state-of-the-art in solving many artificial intelligence problems. However, the requirements engineering (RE) community has yet to adapt to such sweeping changes caused exclusively by data. One reason is that the traditional requirements quality like unambiguity becomes less applicable to data, and so do requirements fault detection techniques like inspections. In this paper, we view deep learning as a class of machines whose effects must be evaluated with direct consideration of inherent data quality attributes: accuracy, consistency, currentness, etc. We substantiate this view by altering stationarity of the multivariate time-series data, and by further analyzing how the stationarity changes affect the behavior of a recurrent neural network in the context of predicting combined sewer overflow. Our work sheds light on the active role RE plays in deep learning.
更多查看译文
关键词
data quality,stationarity,recurrent neural network,metamorphic testing,smart sewer systems
AI 理解论文
溯源树
样例
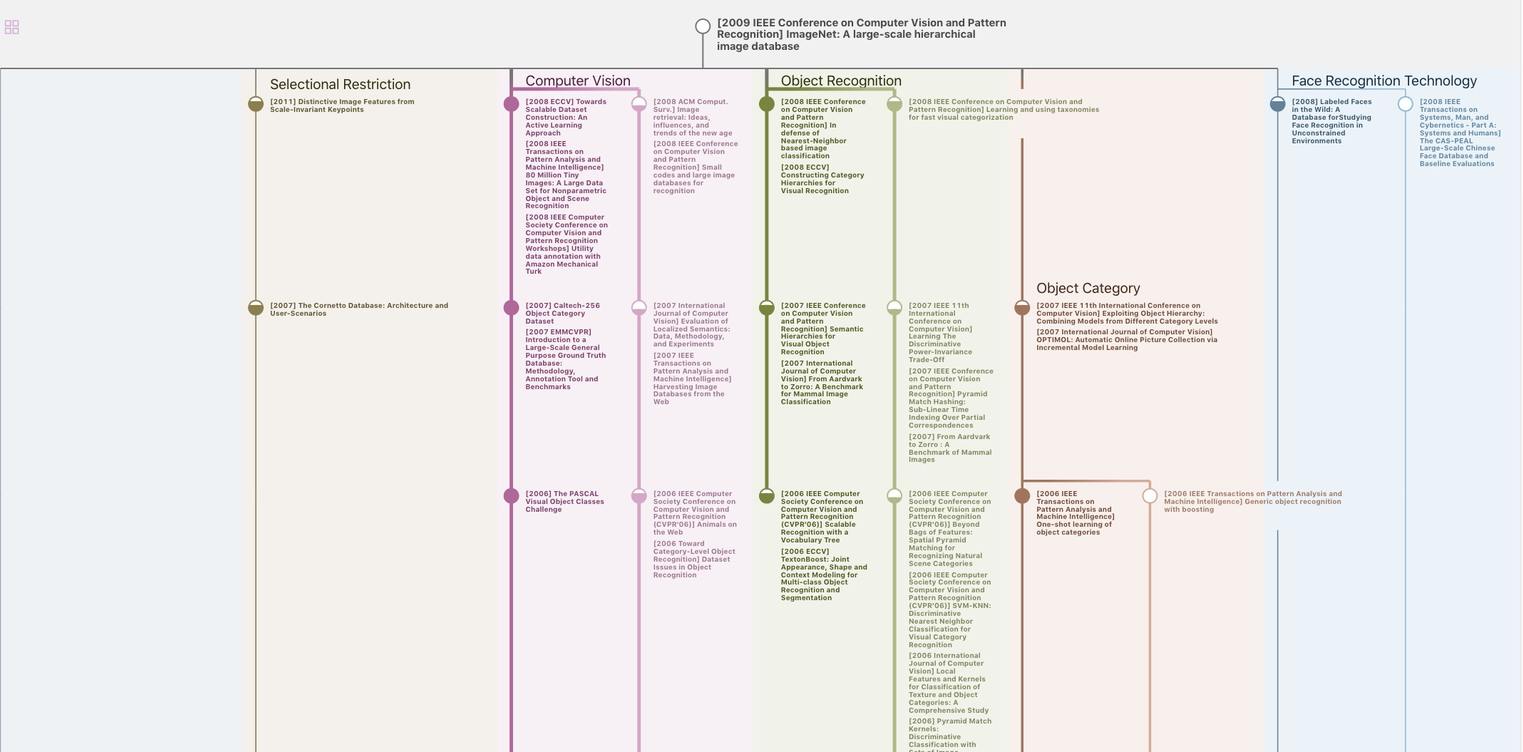
生成溯源树,研究论文发展脉络
Chat Paper
正在生成论文摘要