Interpreting Remaining Useful Life estimations combining Explainable Artificial Intelligence and domain knowledge in industrial machinery
2020 IEEE International Conference on Fuzzy Systems (FUZZ-IEEE)(2020)
摘要
This paper presents the implementation and explanations of a remaining life estimator model based on machine learning, applied to industrial data. Concretely, the model has been applied to a bushings testbed, where fatigue life tests are performed to find more suitable bushing characteristics. Different regressors have been compared Environmental and Operational Condition and setting variables as input data to prognosticate the remaining life on each observation during fatigue tests, where final model is a Random Forest was chosen given its accuracy and explainability potential. The model creation, optimisation and interpretation has been guided combining eXplainable Artificial Intelligence with domain knowledge. Precisely, ELI5 and LIME explainable techniques have been used to perform local and global explanations. These were used to understand the relevance of predictor variables in individual and overall remaining life estimations. The achieved results have been process knowledge gain and expert knowledge validation, assertion of huge potential of data-driven models in industrial processes and highlight the need of collaboration between expert knowledge technicians and eXplainable Artificial Intelligence techniques to understand advanced machine learning models.
更多查看译文
关键词
Explainable Artificial Intelligence,interpret,Machine Learning,data-driven model,Remaining Useful Life,prognosis,industrial process,domain knowledge
AI 理解论文
溯源树
样例
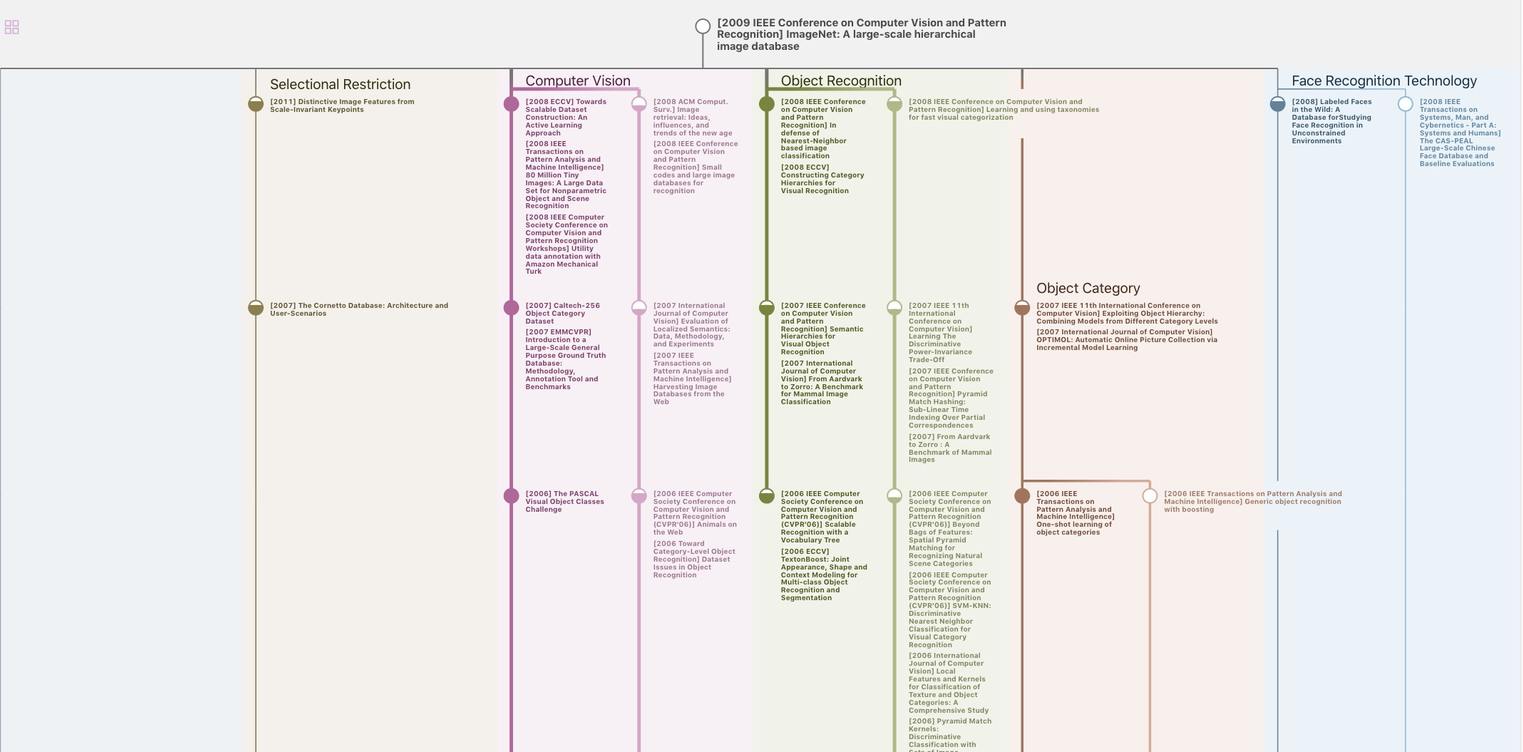
生成溯源树,研究论文发展脉络
Chat Paper
正在生成论文摘要