Online Meta-Forest For Regression Data Streams
2020 INTERNATIONAL JOINT CONFERENCE ON NEURAL NETWORKS (IJCNN)(2020)
摘要
Stream learning is essential when there is limited memory, time and computational power. However, existing streaming methods are mostly designed for classification with only a few exceptions for regression problems. Although being fast, the performance of these online regression methods is inadequate due to their dependence on merely linear models. Besides, only a few stream methods are based on meta-learning that aims at facilitating the dynamic choice of the right model. Nevertheless, these approaches are restricted to recommend learners on a window and not on the instance level. In this paper, we present a novel approach, named Online Meta-Forest, that incrementally induces an ensemble of meta-learners that selects the best set of predictors for each test example. Each meta-learner has the ability to find a non-linear mapping of the input space to the set of induced models. We conduct a series of experiments demonstrating that Online Meta-Forest outperforms related methods on 16 out of 25 evaluated benchmark and domain datasets in transportation.
更多查看译文
关键词
Learning from Data Streams, Adaptive Learning, Meta-Learning, Regression Streams, Data Streams, Online Bagging, Ensemble Learning
AI 理解论文
溯源树
样例
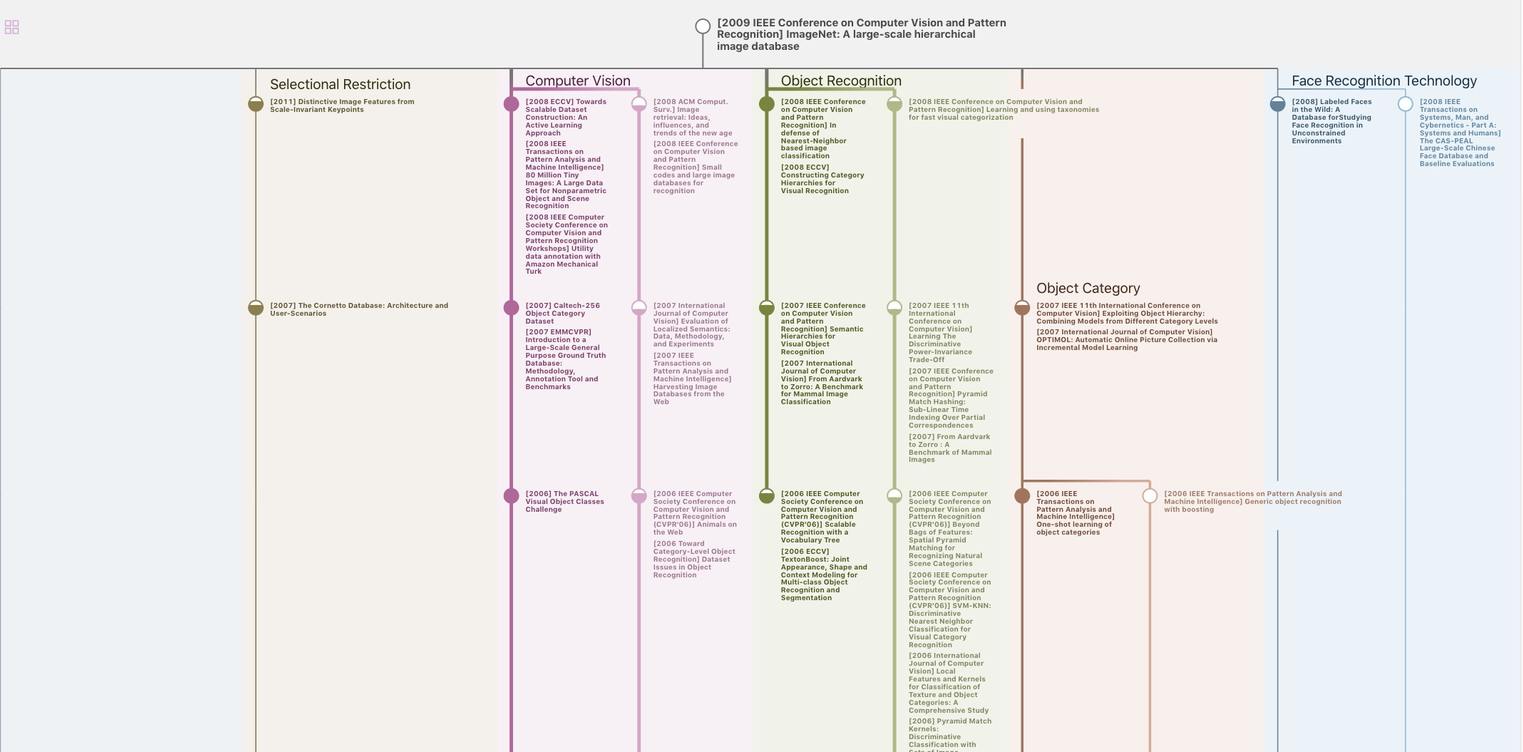
生成溯源树,研究论文发展脉络
Chat Paper
正在生成论文摘要