Predicting Human Errors from Gaze and Cursor Movements
2020 INTERNATIONAL JOINT CONFERENCE ON NEURAL NETWORKS (IJCNN)(2020)
摘要
Intelligent interfaces are increasingly integrated into diverse technological areas. In complex high-risk environments, where humans represent a crucial part of the system and their attention is often divided between simultaneous activities, imminent human errors may have serious consequences. Enhancing interfaces with predictive capabilities promotes the safe and reliable operation of such systems. In this work, we employ a data-driven approach to predict human errors in a special divided attention task involving timing constraints and requiring focused concentration and frequent shifts of attention. We performed a longitudinal study with 10 subjects, and constructed time series from the experimental data using gaze movement and mouse cursor motion features in order to classify successful and failed actions. We evaluate classical machine learning algorithms, compare them with a more traditional temporal modeling approach and a deep learning based LSTM model. Employing a leave-one- subject-out cross-validation procedure we achieve a classification accuracy of up to 86%, with LSTM presenting the highest performance. Furthermore, we investigate the trade-off between evaluation metrics and anticipation window, i.e. the time remaining until the correct action can still be performed. We conclude that prediction is feasible and accuracy and F1-score increases, despite the training dataset becoming greatly imbalanced. Investigating the anticipation window allows to understand how far in advance human errors need to be predicted in order to initiate preventive measures. Our efforts have implications for the design of predictive interfaces involving decision making under time pressure in dynamic divided attention environments.
更多查看译文
关键词
human error, time series, LSTM, gaze tracking, anticipation window, predictive interface
AI 理解论文
溯源树
样例
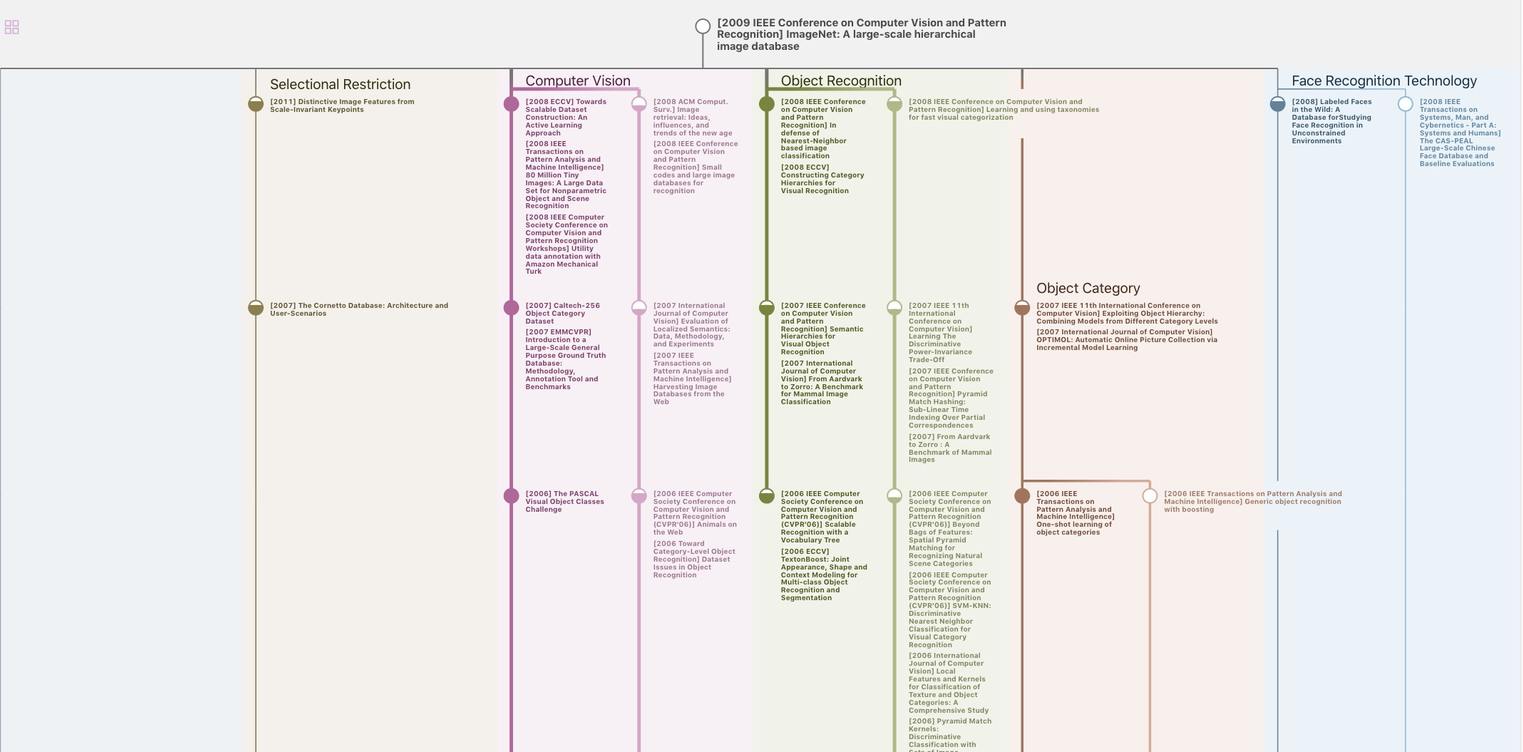
生成溯源树,研究论文发展脉络
Chat Paper
正在生成论文摘要