Dagnet: Exploring The Structure Of Objects For Saliency Detection
2020 INTERNATIONAL JOINT CONFERENCE ON NEURAL NETWORKS (IJCNN)(2020)
摘要
Fully Convolutional Neural Networks (FCNs) greatly promote the development of saliency detection. However, most of the FCN-based models have suffered from the structure of salient objects challenges. The extracted multi-scale features by previous models could help locate the objects with various scales, but they cannot contribute to effectively locating the objects with complex shapes, especially the salient regions that might intertwine with non-salient regions. Moreover, the style of decoder in previous models cannot adequately filter out the disturbance in low-level features, which is sub-optimal to sharpen the boundary of salient objects. In this paper, we propose DAGNet that explores the structure of salient objects from multi-level features to precisely detect salient objects. Firstly, the new dense multi-scale context extraction modules (DMCEMs) are implemented to transmit the rich structural information flow of salient objects from shallower layers to deeper layers, by which our model can locate the objects with complex shapes. Secondly, attention-based deeply refining modules (ADRMs) are designed in an effective attention-based style to effectively restore the boundary of objects stage-by-stage. In the style, the semantic information of high-level features is utilized to guide the shallow layer to filter out disturbance and refine the high-level features. Considering the salient objects surrounded by a cluttered scene, we propose a global context extraction module (GCEM) that can sufficiently understand the cluttered scene of an image from a global view. Comprehensive experiments indicate that our model is superior to 13 state-of-the-art models on 5 benchmark datasets under different evaluation metrics.
更多查看译文
关键词
Fully convolutional neural network, Saliency detection, Structure of salient objects, Deep learning
AI 理解论文
溯源树
样例
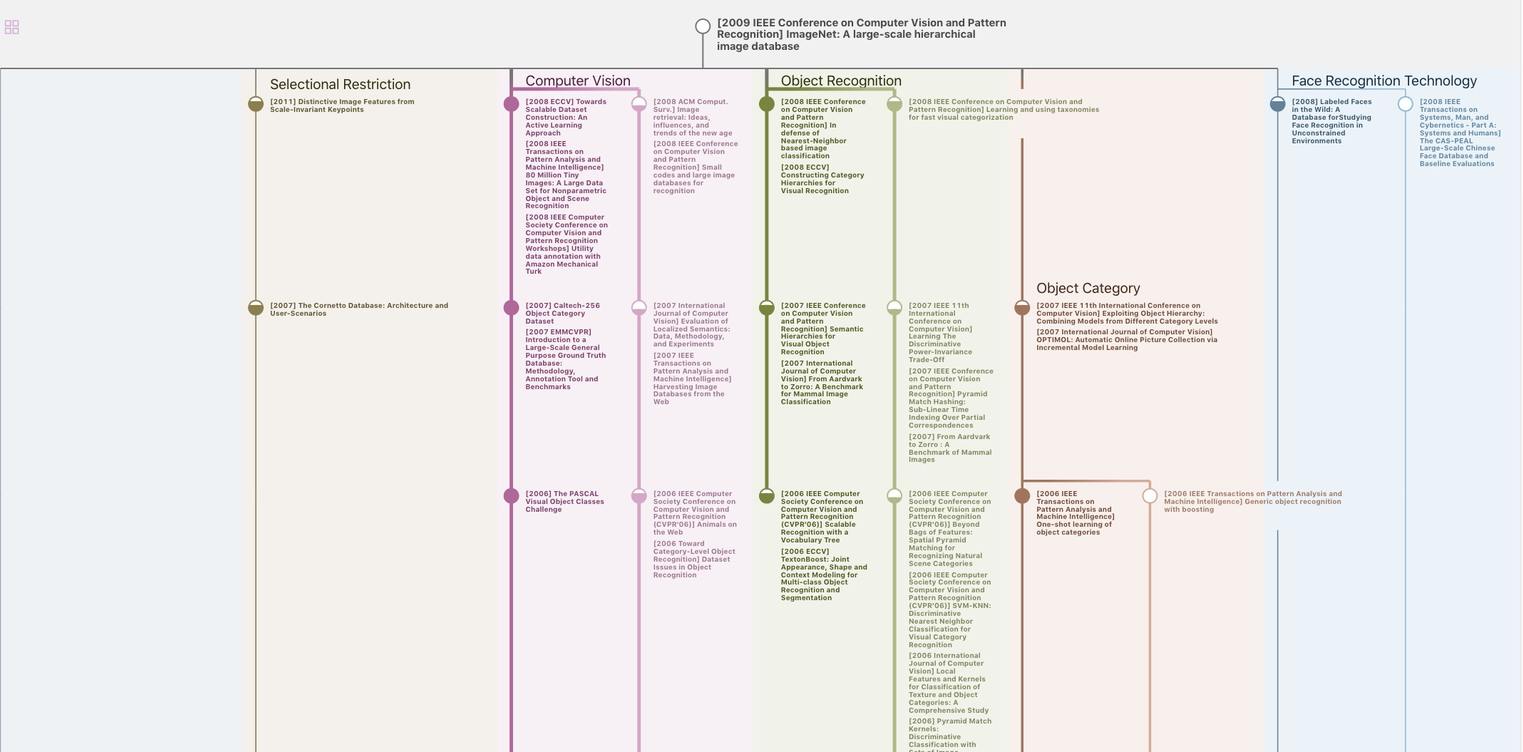
生成溯源树,研究论文发展脉络
Chat Paper
正在生成论文摘要