Mapping And Masking Targets Comparison Using Different Deep Learning Based Speech Enhancement Architectures
2020 INTERNATIONAL JOINT CONFERENCE ON NEURAL NETWORKS (IJCNN)(2020)
摘要
Mapping and Masking targets are both widely used in recent Deep Neural Network (DNN) based supervised speech enhancement. Masking targets are proved to have a positive impact on the intelligibility of the output speech, while mapping targets are found, in other studies, to generate speech with better quality. However, most of the studies are based on comparing the two approaches using the Multilayer Perceptron (MLP) architecture only. With the emergence of new architectures that outperform the MLP, a more generalized comparison is needed between mapping and masking approaches. In this paper, a complete comparison will be conducted between mapping and masking targets using four different DNN based speech enhancement architectures, to work out how the performance of the networks changes with the chosen training target. The results show that there is no perfect training target with respect to all the different speech quality evaluation metrics, and that there is a tradeoff between the denoising process and the intelligibility of the output speech. Furthermore, the generalization ability of the networks was evaluated, and it is concluded that the design of the architecture restricts the choice of the training target, because masking targets result in significant performance degradation for deep convolutional autoencoder architecture.
更多查看译文
关键词
Deep Learning, Speech Enhancement, Training Targets, Time-Frequency Mapping, Time-Frequency Masking
AI 理解论文
溯源树
样例
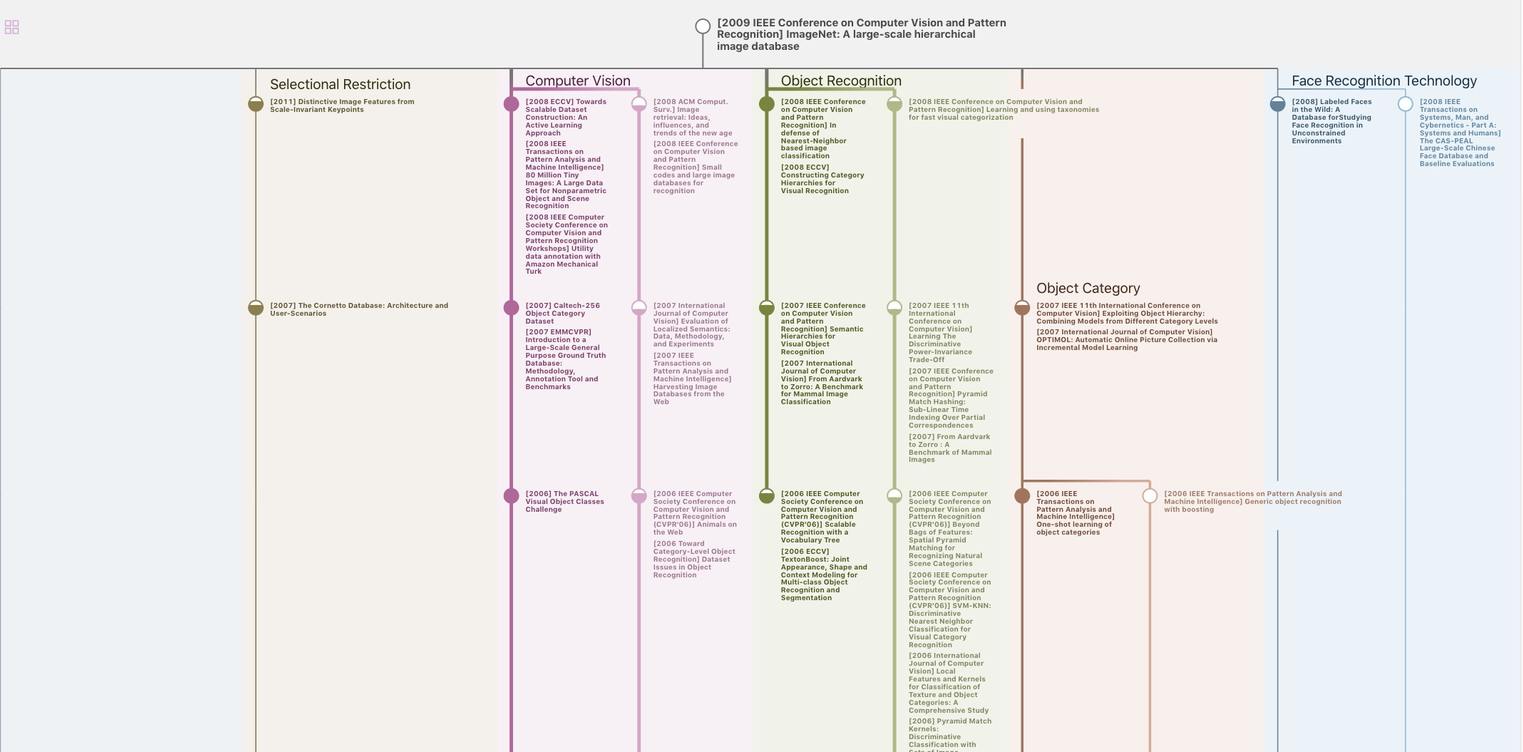
生成溯源树,研究论文发展脉络
Chat Paper
正在生成论文摘要