Learning Word Representation For The Cyber Security Vulnerability Domain
2020 INTERNATIONAL JOINT CONFERENCE ON NEURAL NETWORKS (IJCNN)(2020)
摘要
There have been ever-increasing amounts of security vulnerabilities discovered and reported in recent years. Much of the information related to these vulnerabilities is currently available to the public, in the form of rich, textual data (e.g. vulnerability reports). Many of the state-of-the-art techniques used today to process such textual data rely on so-called word embeddings. As of today, several pre-trained embeddings have been created, many of which rely on general-purpose training datasets such as Google News and Wikipedia. More recently, other domain-specific word embeddings have been created (e.g. in the context of software development) to cope with terminology and ambiguity limitations of existing general-purpose embeddings. The availability of word embeddings for specialised domains is critical for the effectiveness of domain -specific tasks that rely on this technique. In this paper, we propose a word embedding for the cyber security vulnerability domain. We train our embedding model on multiple, rich and heterogeneous security vulnerability information sources publicly available on the web. The benefits of such specialised word embedding are demonstrated through a qualitative comparison of word similarity and the exemplary task of matching security professionals to vulnerability discovery tasks posted to bug bounty programs. We also introduce a new dataset of words pairs similarity with a human judgement that can be used as a benchmark. Our experimental results show that, in the context of cyber security, our domain -specific word embedding outperforms existing pre -trained embeddings built on general-purpose and software engineering datasets.
更多查看译文
关键词
Cyber security vulnerability, word embedding, representation learning, crowdsourcing, vulnerability discovery
AI 理解论文
溯源树
样例
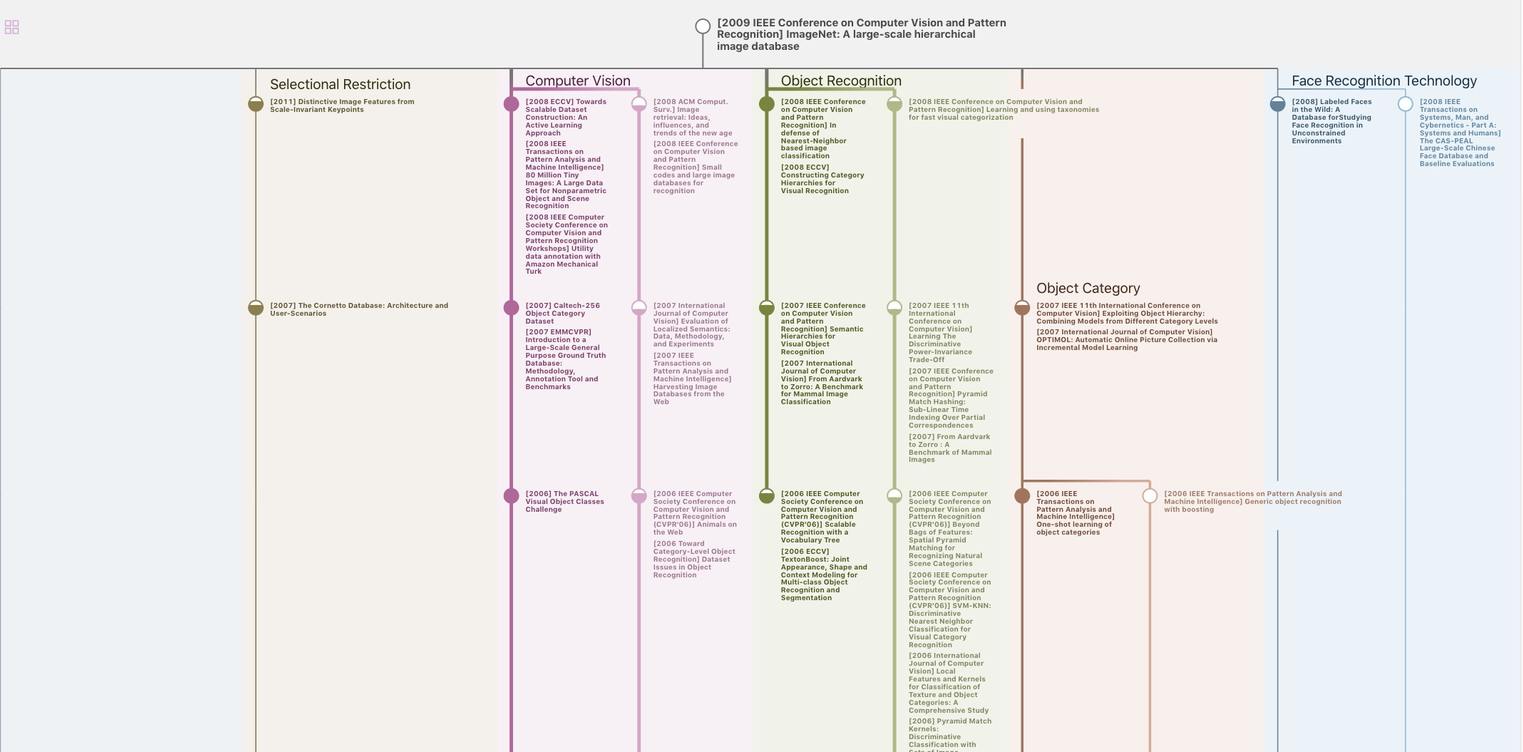
生成溯源树,研究论文发展脉络
Chat Paper
正在生成论文摘要