Risk-Controlled Selective Prediction For Regression Deep Neural Network Models
2020 INTERNATIONAL JOINT CONFERENCE ON NEURAL NETWORKS (IJCNN)(2020)
摘要
Regression deep neural network (DNN) models have been successfully utilized in numerous fields. In real-world applications, large regression errors on individual samples may result in severe consequences. Selective techniques, also known as reject options, have been used to reject predictions with high uncertainty. However, they have yet been mainly considered in classification neural networks (NNs), in comparison to the limited work in regression NNs. In this paper, we considered the selective regression problem from a risk-coverage point of view, and proposed a method to construct a selective regression model given a trained regression DNN model and a desired regression error risk. Then, we proposed to utilize blending variance to quantify uncertainty in regression NNs. We evaluated both the proposed uncertainty function and selective regression models for two real-world applications, the tropical cyclone (TC) intensity estimation problem and the apparent age estimation problem. Our proposed methods achieved promising results. For example, for the TC intensity estimation problem, our selective regression model guaranteed a risk bound (in terms of the root mean squared error (RMSE)) of 9.5 knots for 75% test coverage with a guided confidence level of 0.05, whereas the RMSE value achieved by the state-of-the-art model without selection was 10.5 knots.
更多查看译文
关键词
selective prediction, regression, deep neural networks, blending variance
AI 理解论文
溯源树
样例
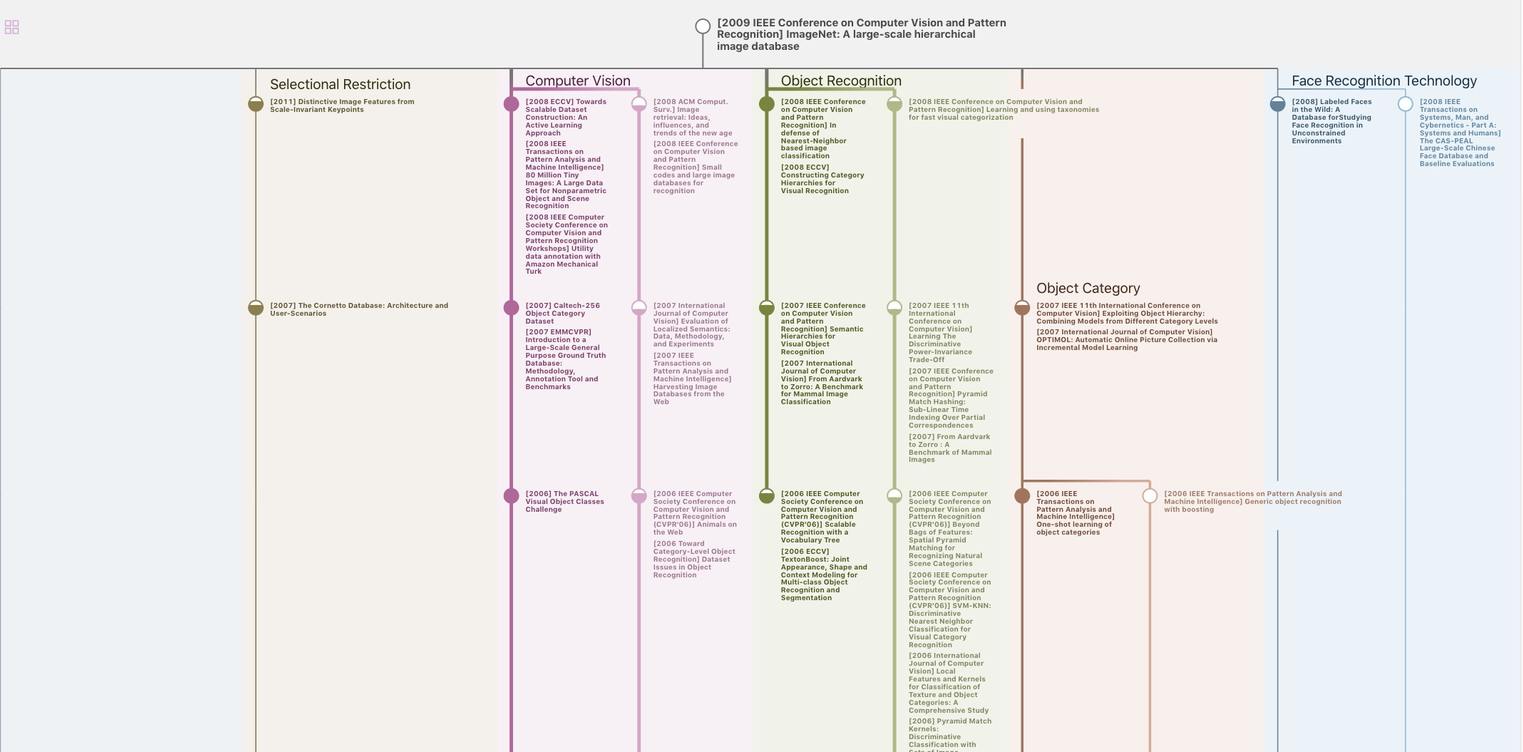
生成溯源树,研究论文发展脉络
Chat Paper
正在生成论文摘要