Improved Policy Extraction via Online Q-Value Distillation.
IJCNN(2020)
摘要
Deep neural networks are capable of solving complex control tasks in challenging environments, but their learned policies are hard to interpret. Not being able to explain or verify them limits their practical applicability. By contrast, decision trees lend themselves well to explanation and verification, but are not easy to train, especially in an online fashion. In this work we introduce Q-BSP trees and propose an Ordered Sequential Monte Carlo training algorithm that efficiently distills the Q-function from fully trained deep Q-networks into a tree structure. Q-BSP forests are used to generate the partitioning rules that transparently reconstruct an accurate value function. We explain our approach and provide results that convincingly beat earlier online policy distillation methods with respect to their own performance benchmarks.
更多查看译文
关键词
decision trees,ordered sequential Monte Carlo training algorithm,learned policies,complex control tasks,deep neural networks,online Q-value distillation,policy extraction,earlier online policy distillation methods,accurate value function,partitioning rules,Q-BSP forests,tree structure,fully trained deep Q-networks,Q-BSP trees
AI 理解论文
溯源树
样例
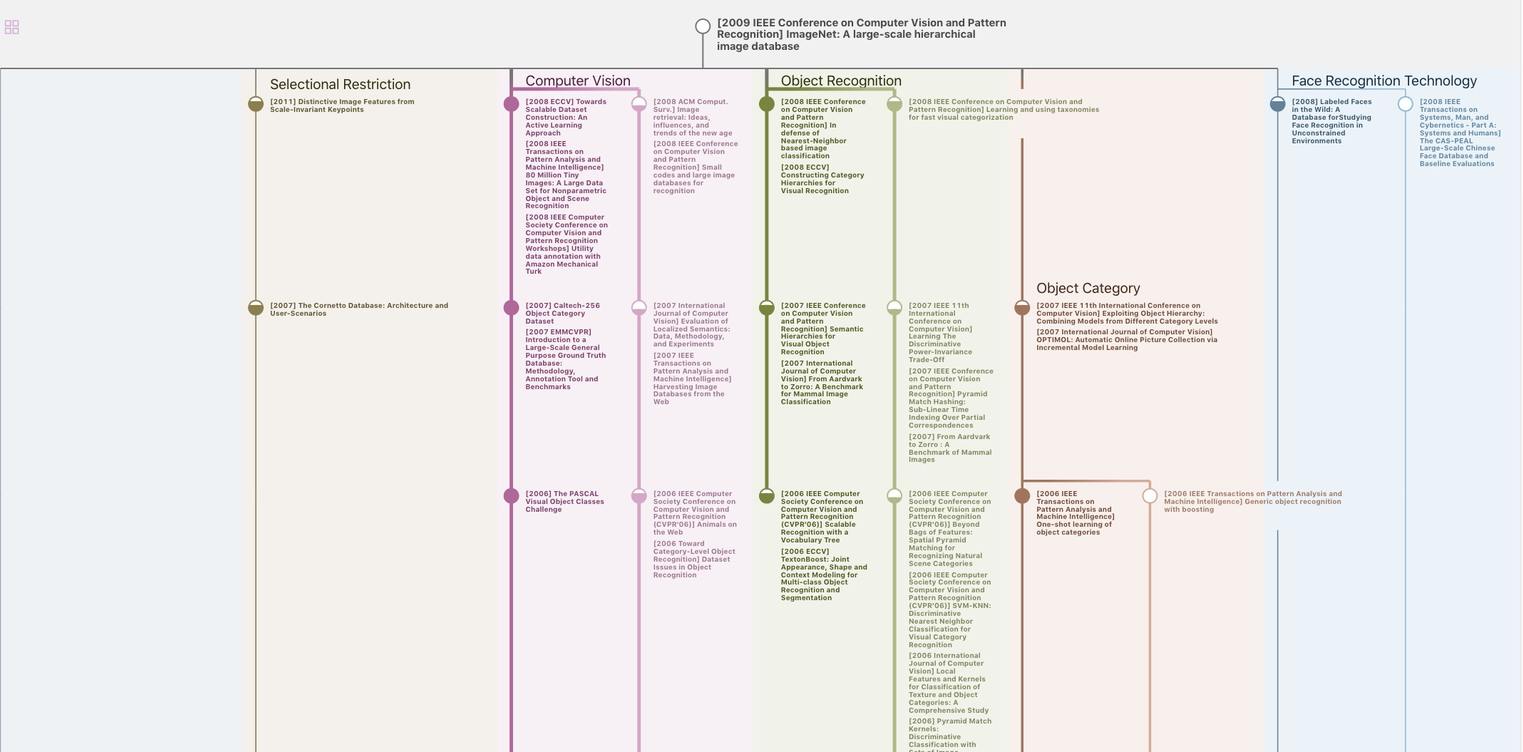
生成溯源树,研究论文发展脉络
Chat Paper
正在生成论文摘要