High Capacity Neural Block Classifiers With Logistic Neurons And Random Coding
2020 INTERNATIONAL JOINT CONFERENCE ON NEURAL NETWORKS (IJCNN)(2020)
摘要
We show that neural networks with logistic output neurons and random codewords can store and classify far more patterns than those that use softmax neurons and 1-in-K encoding. Logistic neurons can choose binary codewords from an exponentially large set of codewords. Random coding picks the binary or bipolar codewords for training such deep classifier models. This method searched for the bipolar codewords that minimized the mean of an inter-codeword similarity measure. The method used blocks of networks with logistic input and output layers and with few hidden layers. Adding such blocks gave deeper networks and reduced the problem of vanishing gradients. It also improved learning because the input and output neurons of an interior block must equal the input pattern's code word. Deep-sweep training of the neural blocks further improved the classification accuracy. The networks trained on the CIFAR-100 and the Caltech-256 image datasets. Networks with 40 output logistic neurons and random coding achieved much of the accuracy of 100 softmax neurons on the CIFAR-100 patterns. Sufficiently deep random-coded networks with just 80 or more logistic output neurons had better accuracy on the Caltech-256 dataset than did deep networks with 256 softmax output neurons.
更多查看译文
关键词
logistic network, blocking, random coding, deep sweep training, backpropagation invariance
AI 理解论文
溯源树
样例
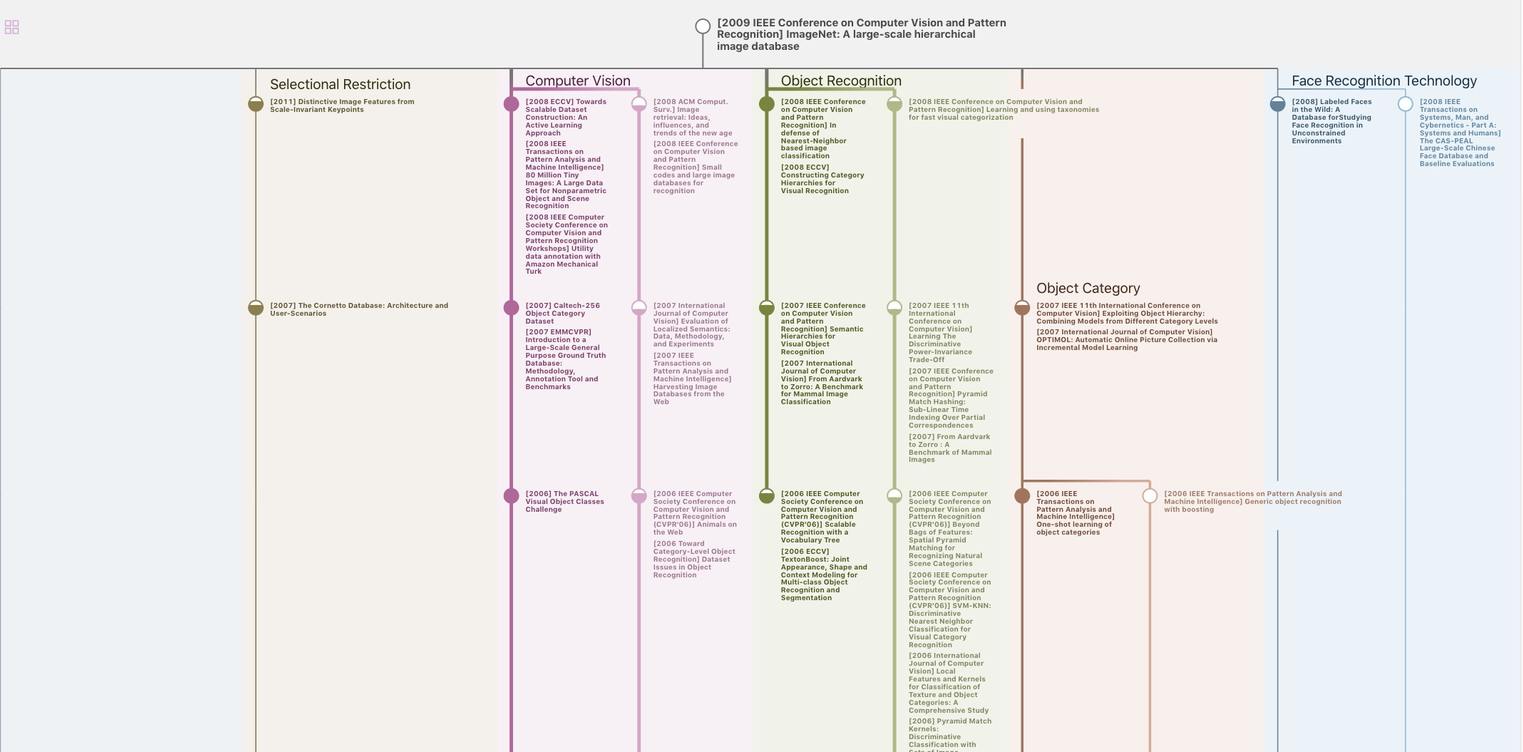
生成溯源树,研究论文发展脉络
Chat Paper
正在生成论文摘要