Deep Learning Using Augmentation via Registration - 1st Place Solution to the AutoImplant 2020 Challenge.
AutoImplant@MICCAI(2020)
摘要
Automatic cranial implant design can save clinicians time and resources by computing the implant shape and size from a single image of a defective skull. We aimed to improve upon previously proposed deep learning methods by augmenting the training data set using transformations that warped the images into different shapes and orientations. The transformations were computed by non-linearly registering the complete skull images between the 100 subjects in the training data set. The transformations were then applied to warp each of the defective and complete skull images so that the shape and orientation resembled that of a different subject in the training set. One hundred ninety-seven of the registrations failed, resulting in an augmented training set of 9,803 defective and complete skull image pairs. The augmented training set was used to train an ensemble of four U-Net models to predict the complete skull shape from the defective skulls using cross-validation. The ensemble of models performed very well and predicted the implant shapes with a mean dice similarity coefficient of 0.942 and a mean Hausdorff distance of 3.598mm for all 110 test cases. Our solution ranked first among all participants of the AutoImplant 2020 challenge. The code for this project is available at https://github.com/ellisdg/3DUnetCNN.
更多查看译文
关键词
Deep learning, Shape completion, Augmentation
AI 理解论文
溯源树
样例
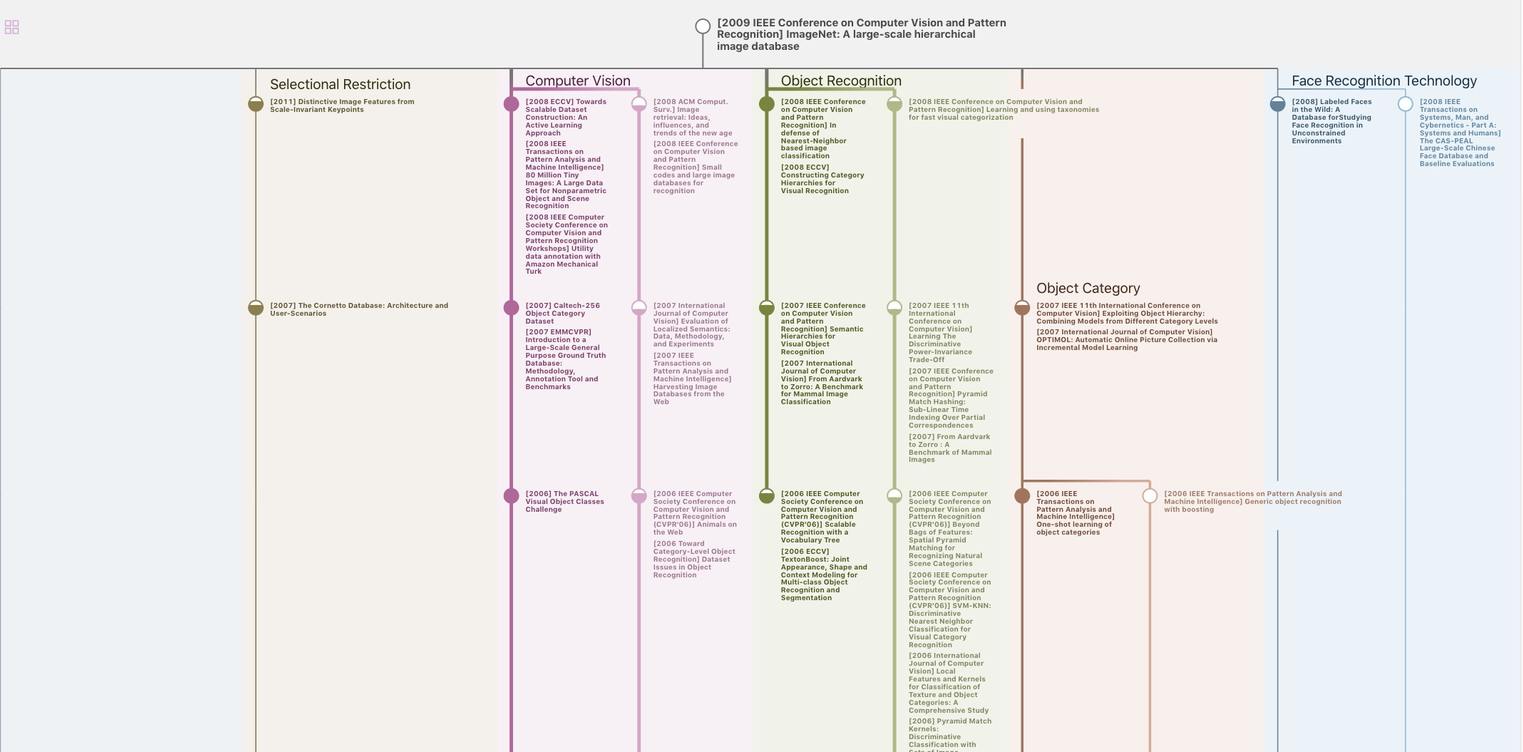
生成溯源树,研究论文发展脉络
Chat Paper
正在生成论文摘要