Experimental Comparison of Machine Learning Models in Malware Packing Detection
2020 21st Asia-Pacific Network Operations and Management Symposium (APNOMS)(2020)
摘要
Recently , malware is widely distributed by combining recent technologies such as packing, encoding and obfuscation to bypass anti-virus software. These kinds of technologies allow malware to survive longer, infect various computers and devices for longer periods of time, create a number of mutated malware, and make experts spend longer to analyze malware. Packers disrupt the reverse engineering process, making it difficult for security researchers to analyze new or unknown malware. Thus, we need to analyze as many malware as possible by first detecting the packed malware and analyzing not-packed malware, and then unpack the packed malware. Previously, the packing detection methods were based on mainly signature and entropy detection. However, these methods have increased the undetected rate with the appearance of custom packers. Due to these problems, there have been many research efforts on machine learning-based malware packing detection and classification. In this paper, we present an extensive experimental comparison of these machine learning-based algorithms. In particular, we extract a total of 13 important features and considers eight machine learning algorithms to detect the packing of malware. Experimental results show that we can also detect well malware packed by custom packers which did not studied in previous studies.
更多查看译文
关键词
Malware,Packing,Machine Learning,Security
AI 理解论文
溯源树
样例
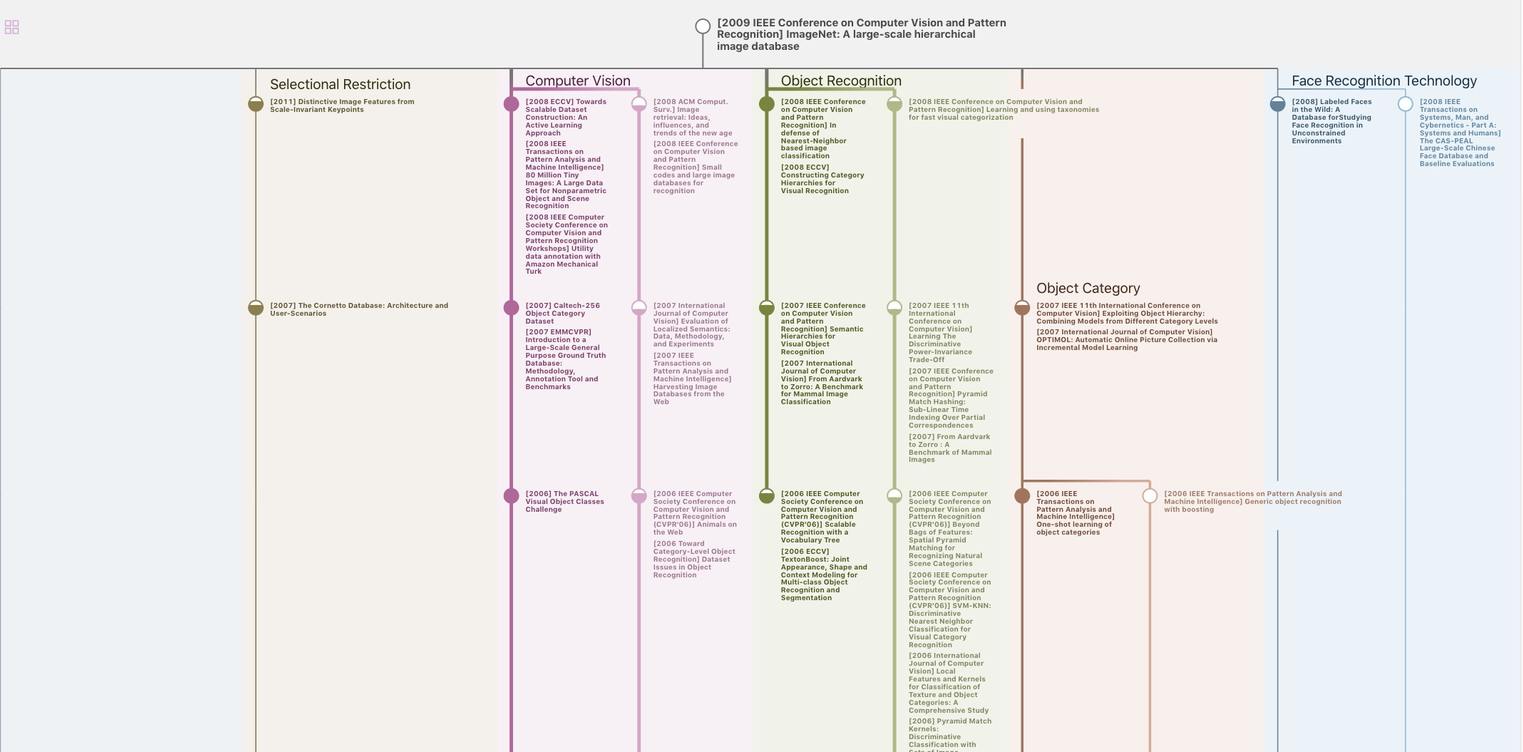
生成溯源树,研究论文发展脉络
Chat Paper
正在生成论文摘要