Unsupervised Change Detection in Multi-temporal Satellite Images Based on Structural Patch Decomposition and k-means Clustering for Landslide Monitoring.
IUKM(2020)
摘要
This paper proposes a new, simple, and effective unsupervised change detection method for multi-temporal satellite imagery in the landslide monitoring system. The method combines a modified version of k-means clustering, so called the adaptive k-means clustering algorithm and a structural consistency map to binarize input multi-temporal satellite images. The absolute differences between pairs of contiguous binary images are used to construct a change map. The structural consistency map is generated by a method based on the structural patch decomposition. The evaluation results show that the proposed method is considerably better than a conventional method based on principal component analysis (PCA) and k-means clustering in terms of pixel error rate, correctness, and robustness against noise addition. For the pixel error rate and the correctness, the improvements are approximately \\(42.61\\%\\) and \\(13.92\\%\\), respectively. Also, when noises are added into the input images, the pixel error rate of the proposed method is improved by \\(98\\%\\) from that of the PCA-based method, and the correctness is improved by \\(77.27\\%\\).
更多查看译文
关键词
Change detection, Landslide monitoring, Structural patch decomposition, k-means clustering, Multi-temporal satellite imagery
AI 理解论文
溯源树
样例
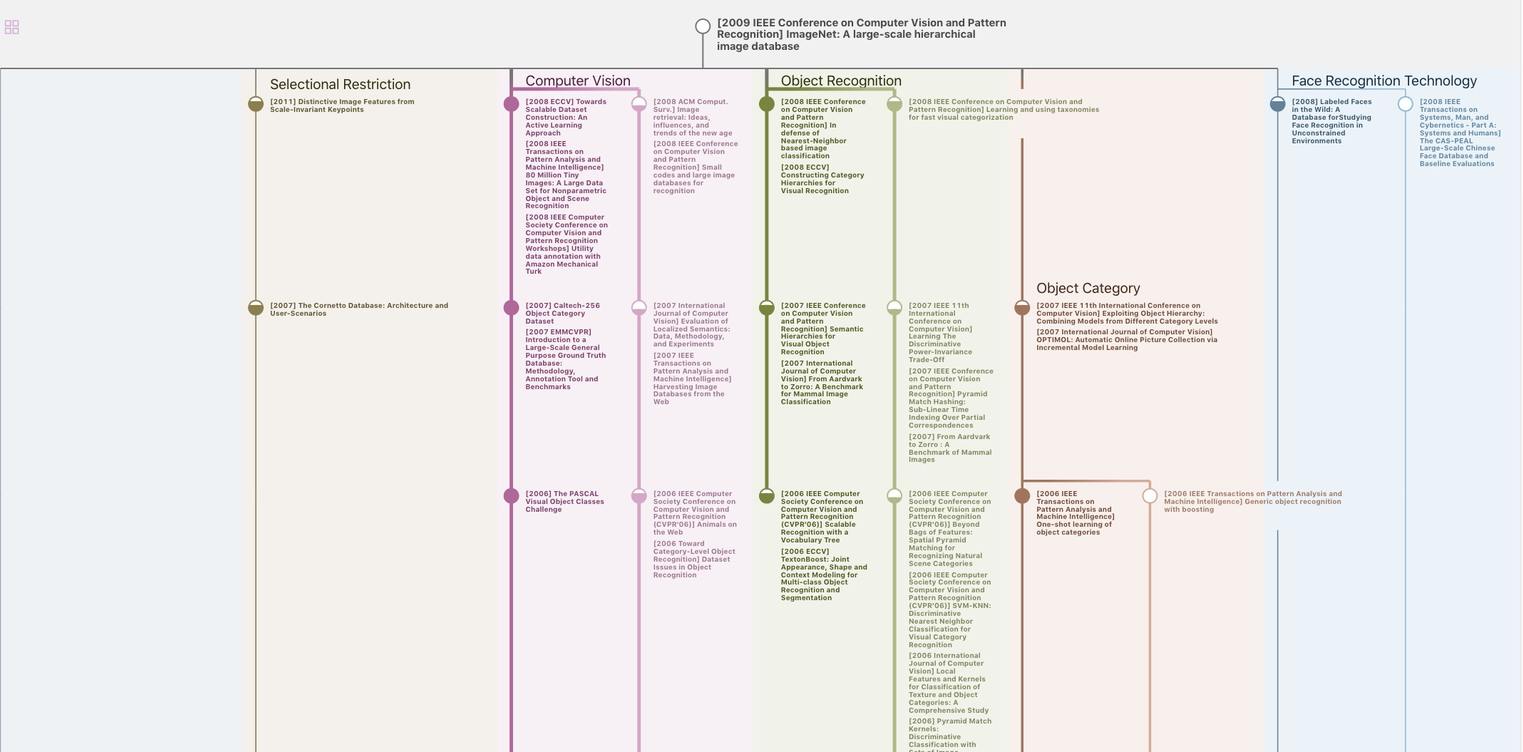
生成溯源树,研究论文发展脉络
Chat Paper
正在生成论文摘要