Multipath Parallel Hybrid Deep Neural Networks Framework for Remaining Useful Life Estimation
2020 IEEE International Conference on Prognostics and Health Management (ICPHM)(2020)
摘要
The paper introduces a multi-path parallel hybrid deep neural design for remaining useful life (RUL) estimation of critical infrastructure, referred to as the MPHD. The proposed framework integrates three noisy deep learning structures in parallel: (a) A noisy path uses Long Short-Term Memory (LSTM), (b) A noisy path uses Gated Recurrent Unit (GRU), and; (c) A noisy path uses Convolutional Neural Network (CNN), The proposed framework aims to collect different types of features from the most popular deep neural networks architectures and then utilizing a fusion center consists of noisy fully connected multilayer neural network, to combine the collected features of the three parallel paths and predict the RLU. The MPHD framework utilizes noisy training to improve accuracy, enhance robustness, and mitigate the overfitting problem associated with neural networks. The proposed model is evaluated by utilizing (CMAPSS) dataset, which is provided by NASA.
更多查看译文
关键词
C-MAPSS,Prognostics and health management,Remaining Useful Life,LSTM,GRU,CNN,Noisy training,Deep learning,Hybrid Models
AI 理解论文
溯源树
样例
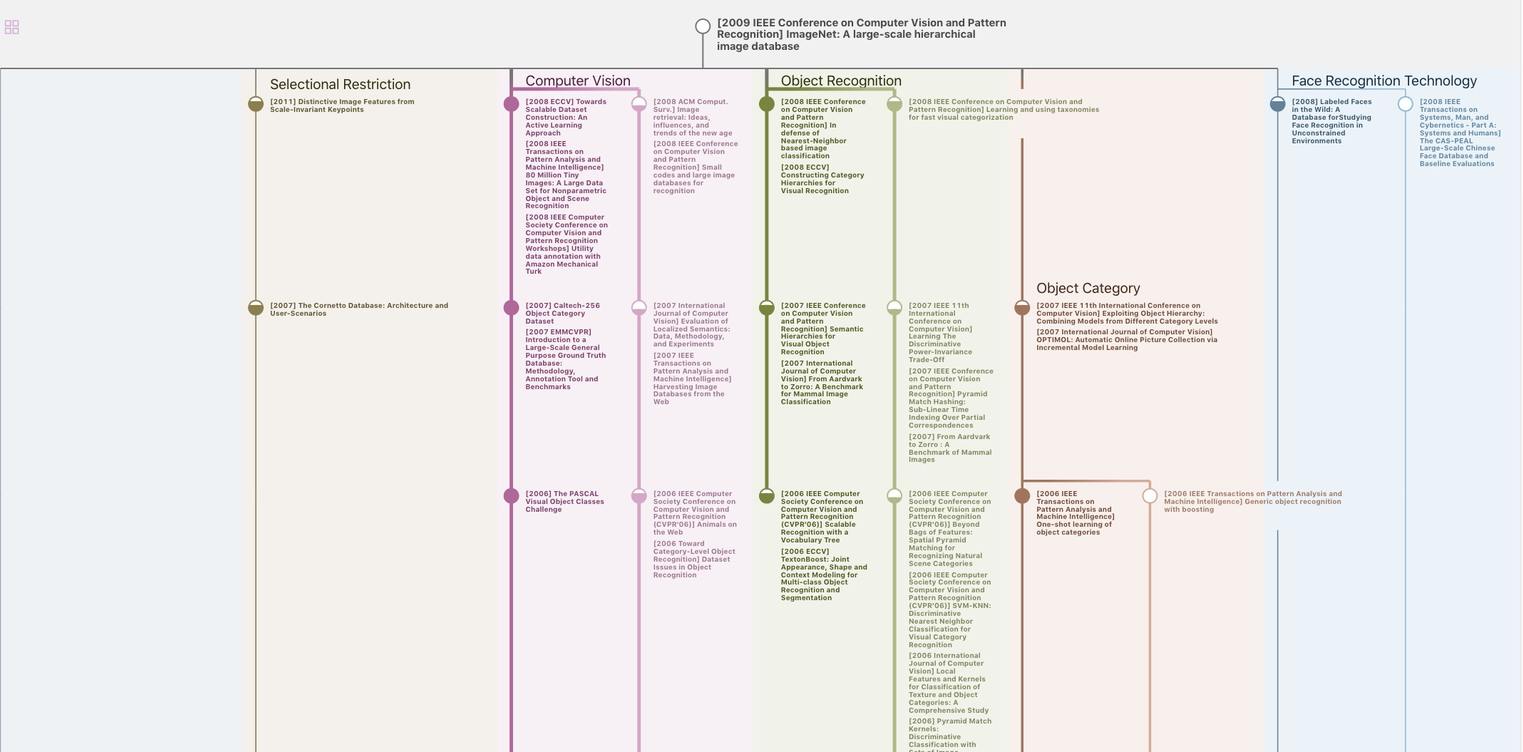
生成溯源树,研究论文发展脉络
Chat Paper
正在生成论文摘要