Stereo Disparity Estimation via Joint Supervised, Unsupervised, and Weakly Supervised Learning
2020 IEEE INTERNATIONAL CONFERENCE ON IMAGE PROCESSING (ICIP)(2020)
摘要
We propose SUW-Stereo, a deep-learning framework with joint supervised learning (S), unsupervised learning (U), and weakly-supervised learning (W) for disparity estimation. The supervised learning module optimizes a disparity estimation network by knowledge based on the ground-truth disparity. In contrast, the unsupervised learning module has no knowledge of the ground-truth disparity, but optimizes the disparity estimation network by predicting the current right image from the left image and estimated disparity. The weakly supervised learning uses other expert information to derive some information about the disparity, without using ground-truth disparity knowledge either. SUW-Stereo trains the deep-learning networks end-to-end with joint optimization of the desired SUW objectives. We demonstrate that SUW-Stereo can even improve the accuracy of current state-of-the-art networks such as AMNet or GANet.
更多查看译文
关键词
Disparity estimation, stereo, supervised learning, unsupervised learning, weakly supervised learning
AI 理解论文
溯源树
样例
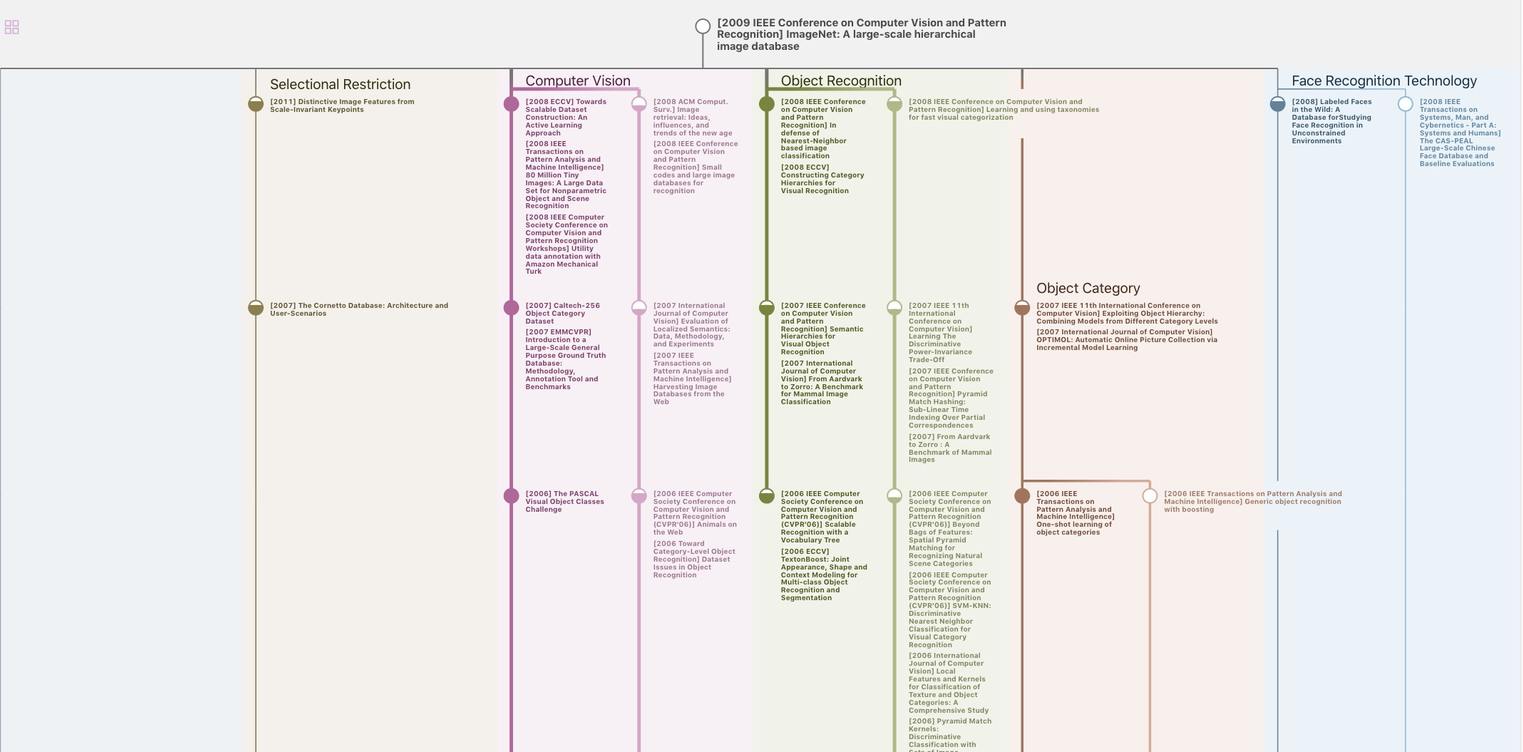
生成溯源树,研究论文发展脉络
Chat Paper
正在生成论文摘要