Variational Autoencoder Based Unsupervised Domain Adaptation For Semantic Segmentation
2020 IEEE INTERNATIONAL CONFERENCE ON IMAGE PROCESSING (ICIP)(2020)
摘要
Unsupervised domain adaptation, which transfers supervised knowledge from a labeled domain to an unlabeled domain, remains a tough problem in the field of computer vision, especially for semantic segmentation. Some methods inspired by adversarial learning and semi-supervised learning have been developed for unsupervised domain adaptation in semantic segmentation and achieved outstanding performances. In this paper, we propose a novel method for this task. Like adversarial learning-based methods using a discriminator to align the feature distributions from different domains, we employ a variational autoencoder to get to the same destination but in a non-adversarial manner. Since the two approaches are compatible, we also integrate an adversarial loss into our method. By further introducing pseudo labels, our method can achieve state-of-the-art performances on two benchmark adaptation scenarios, GTA5-to-CITYSCAPES and SYNTHIA-to-CITYSCAPES.
更多查看译文
关键词
Unsupervised domain adaptation, semantic segmentation, variational autoencoder, adversarial learning
AI 理解论文
溯源树
样例
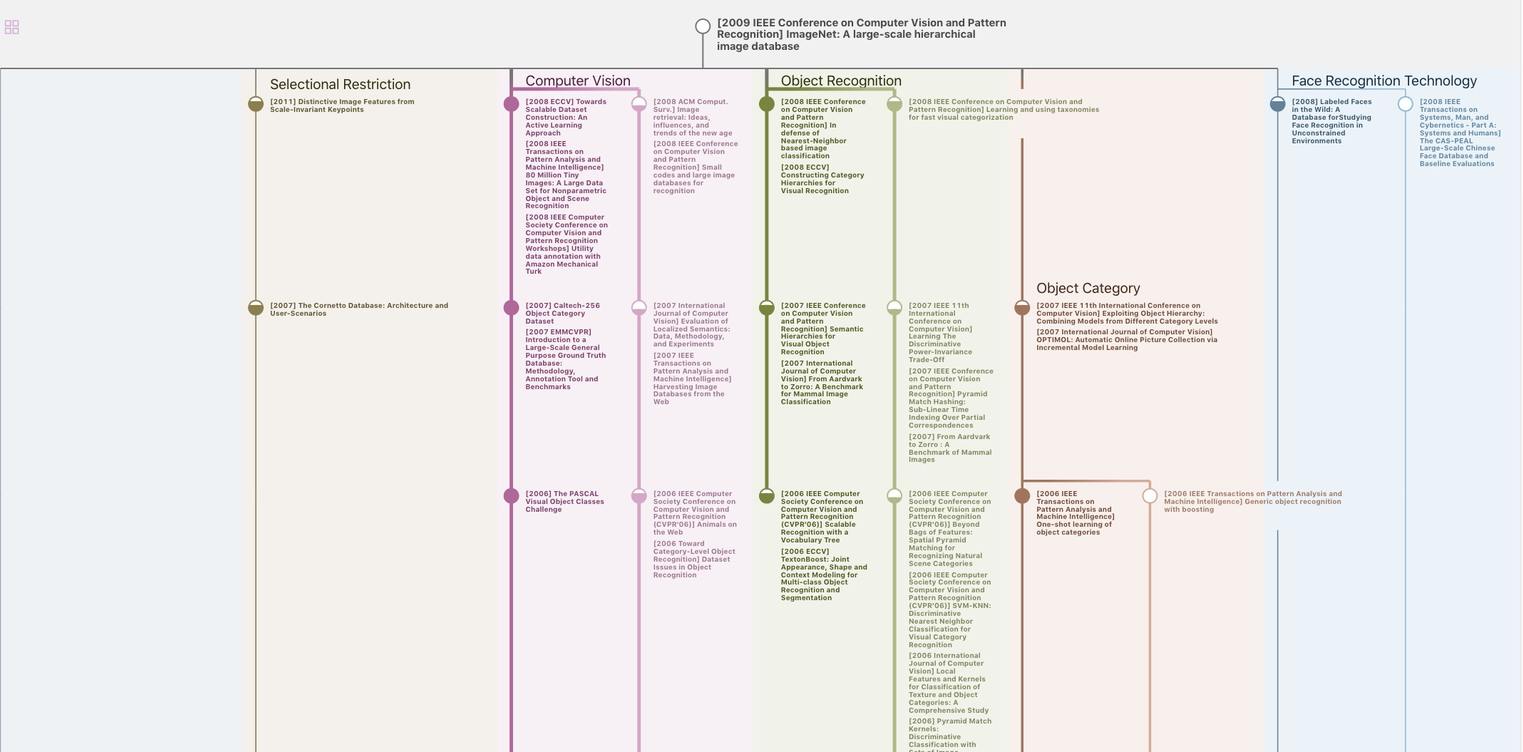
生成溯源树,研究论文发展脉络
Chat Paper
正在生成论文摘要