Gapnet: Generic-Attribute-Pose Network For Fine-Grained Visual Categorization Using Multi-Attribute Attention Module
2020 IEEE INTERNATIONAL CONFERENCE ON IMAGE PROCESSING (ICIP)(2020)
摘要
This paper proposes a multi-task learning framework for fine-grained visual categorization (FGVC) referred to as Generic-Attribute-Pose Network (GAPNet) that is capable of attending discriminating parts depending on the pose and part-attribute of an object using multi-attribute attention. FGVC is a challenging task that involves categorical data with small inter-class variation and large intra-class variation. Multi-Attribute Attention Module (MAAM) guides the GAPNet to focus on multiple parts of the image feature by emphasizing appropriate feature channels given both pose and part-attribute features. Experiments on Caltech-UCSD Birds and NABirds datasets demonstrate that GAPNet is competitive with other state-of-the-art methods, and ablation study on GAPNet conditioned on pose and part-attribute feature shows that GAPNet performs best when conditioned on both pose and part-attribute features.
更多查看译文
关键词
Fine-Grained Visual Categorization, Multi-Task Learning, Attention Mechanism
AI 理解论文
溯源树
样例
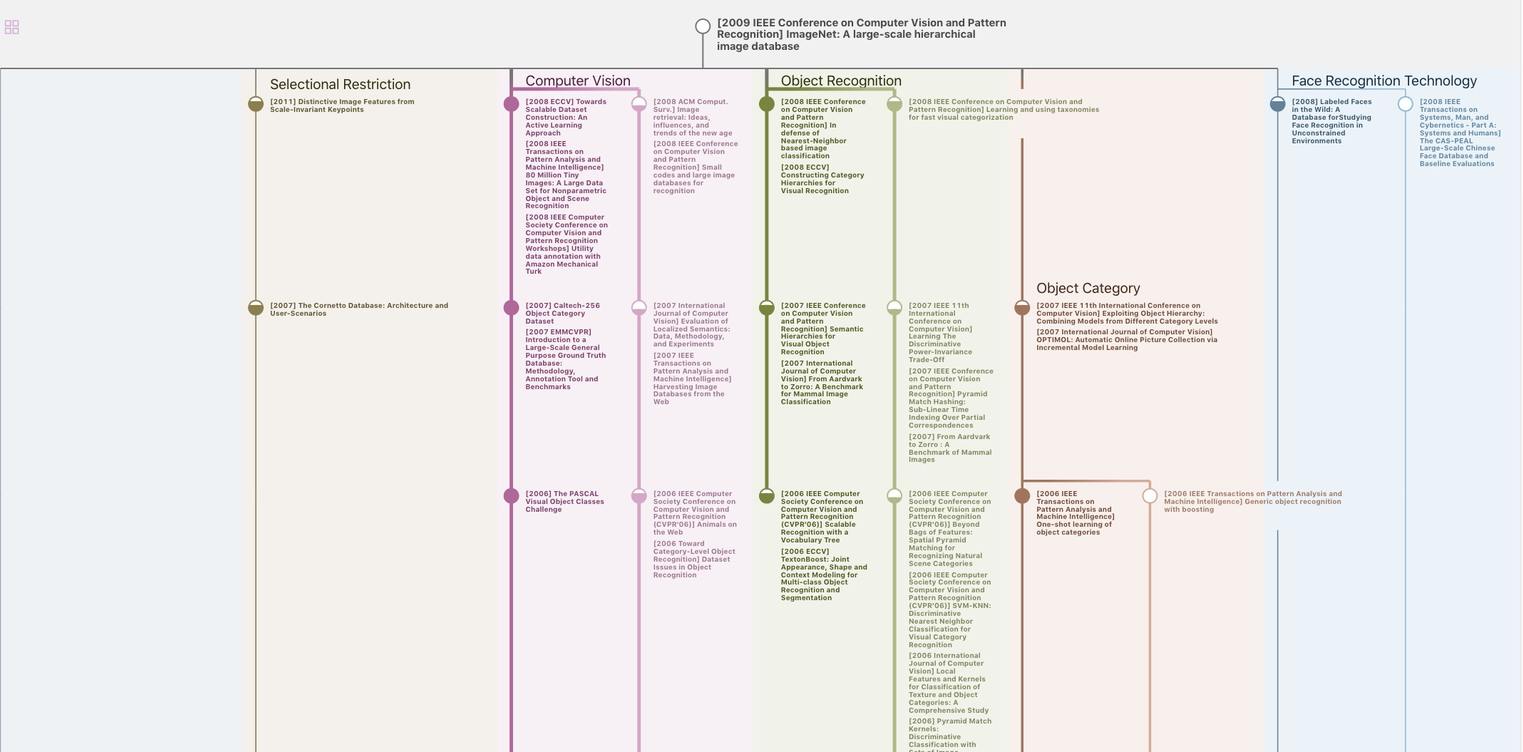
生成溯源树,研究论文发展脉络
Chat Paper
正在生成论文摘要