ST-LSTM: Spatio-Temporal Graph Based Long Short-Term Memory Network For Vehicle Trajectory Prediction
2020 IEEE INTERNATIONAL CONFERENCE ON IMAGE PROCESSING (ICIP)(2020)
摘要
Autonomous vehicles need the ability to predict the trajectory of surrounding vehicles, so as to make a rational decision planning, improve driving safety and ride comfort. In this paper, a new hierarchical Long Short-Term Memory (LSTM) based on Spatio-Temporal (ST) graph is proposed for vehicle trajectory prediction. Our ST-LSTM uses three layers of different LSTMs to capture the information of spatial, temporal and trajectory data, and LSTM-based encoder-decoder model as a whole, which is capable of accurately predicting future trajectories for vehicles on the highway. Our model trained and validated on the publicly available NGSIM US-101 and I-80 datasets. In comparison to state-of-art methods, our method could achieve a more accurate prediction trajectory over 5s time horizon.
更多查看译文
关键词
Spatio-Temporal (ST) graph, Long Short-Term Memory (LSTM), encoder-decoder model, trajectory prediction, autonomous vehicles
AI 理解论文
溯源树
样例
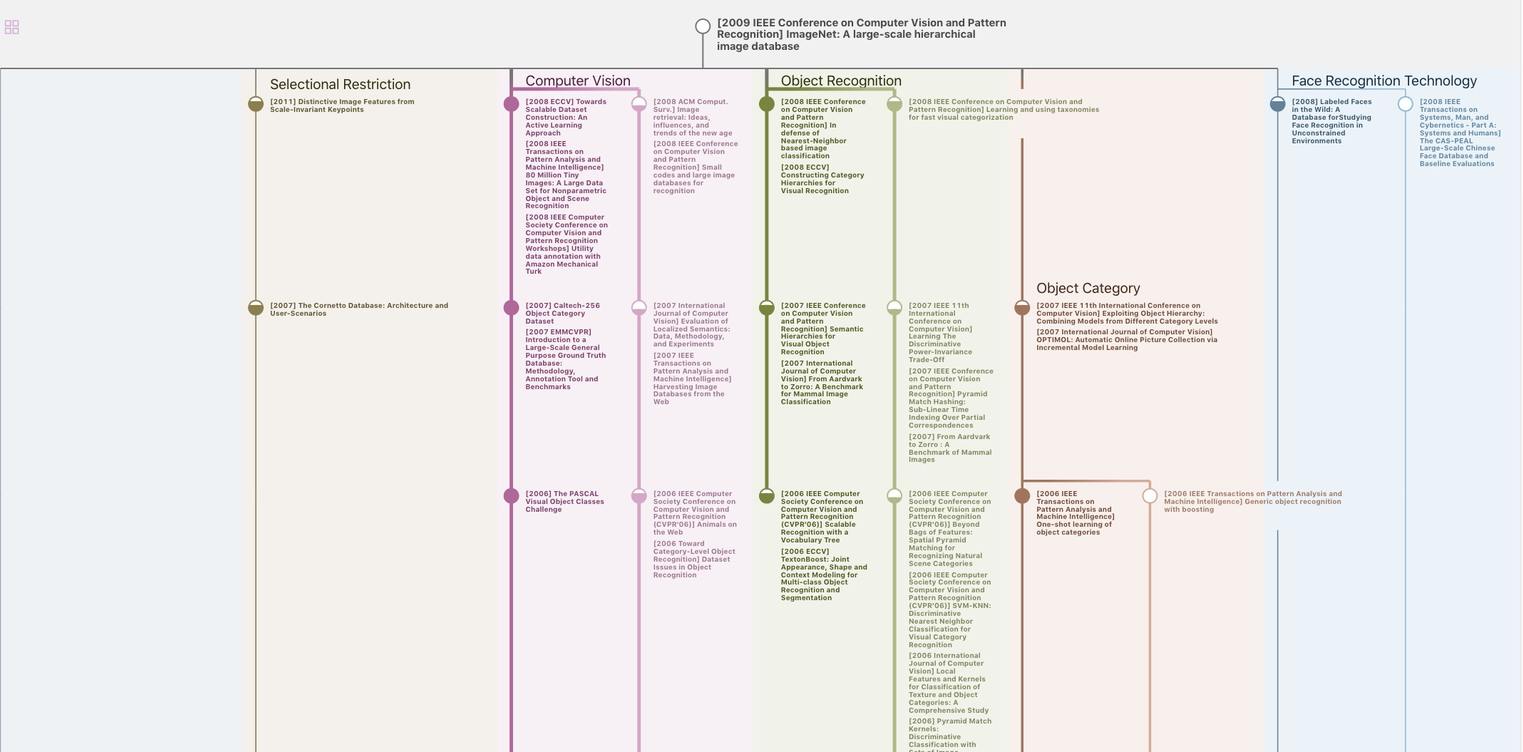
生成溯源树,研究论文发展脉络
Chat Paper
正在生成论文摘要