An Actor-Critic Approach for Legible Robot Motion Planner.
ICRA(2020)
摘要
In human-robot collaboration, it is crucial for the robot to make its intentions clear and predictable to the human partners. Inspired by the mutual learning and adaptation of human partners, we suggest an actor-critic approach for a legible robot motion planner. This approach includes two neural networks and a legibility evaluator: 1) A policy network based on deep reinforcement learning (DRL); 2) A Recurrent Neural Networks (RNNs) based sequence to sequence (Seq2Seq) model as a motion predictor; 3) A legibility evaluator that maps motion to legible reward. Through a series of human-subject experiments, we demonstrate that with a simple handicraft function and no real-human data, our method lead to improved collaborative performance against a baseline method and a non-prediction method.
更多查看译文
关键词
actor-critic approach,legible robot motion planner,human-robot collaboration,human partners,mutual learning,legibility evaluator,policy network,deep reinforcement learning,sequence model,Seq2Seq,motion predictor,maps motion,legible reward,human-subject experiments,real-human data,recurrent neural networks based sequence
AI 理解论文
溯源树
样例
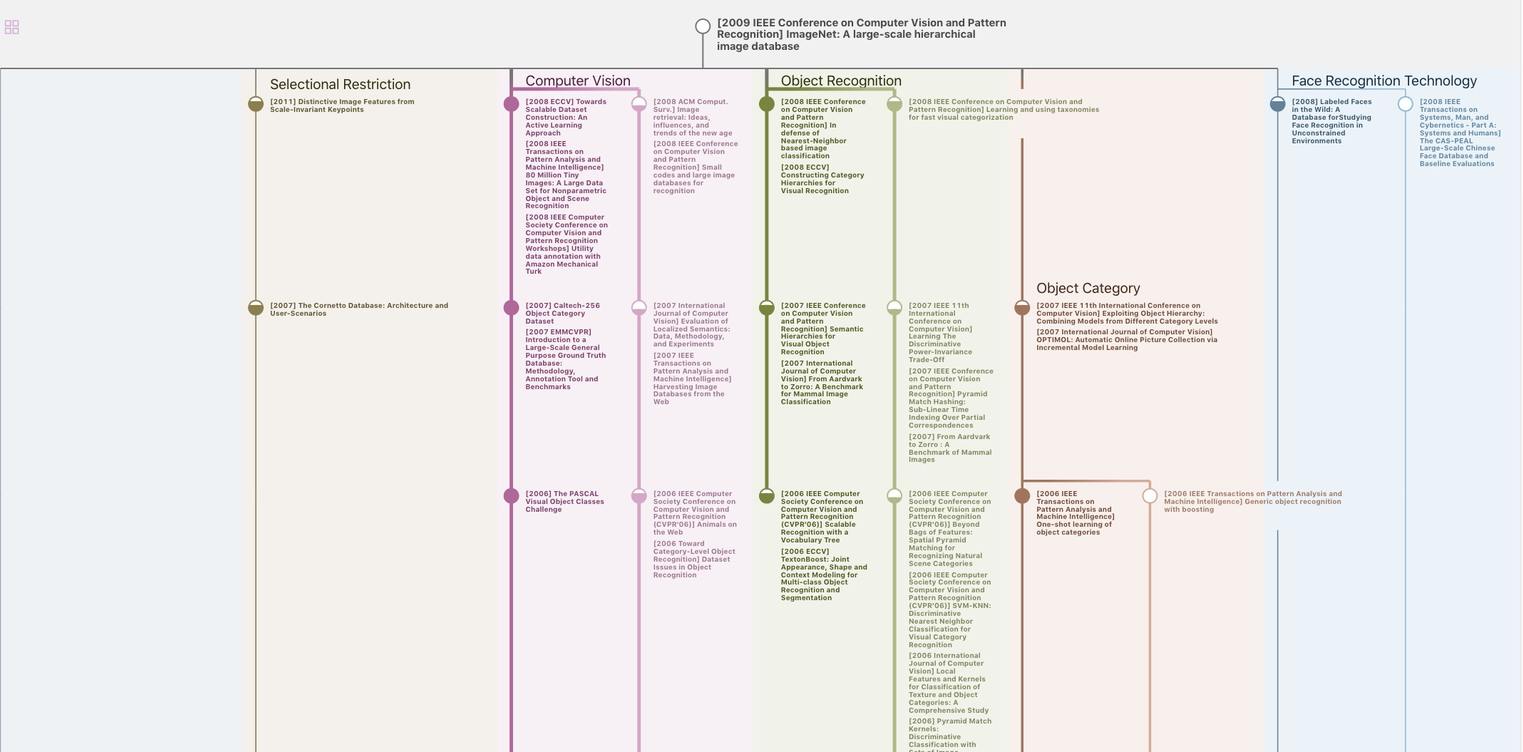
生成溯源树,研究论文发展脉络
Chat Paper
正在生成论文摘要