Generative Modeling of Environments with Scene Grammars and Variational Inference.
ICRA(2020)
摘要
In order to understand how a robot will perform in the open world, we aim to establish a quantitative understanding of the distribution of environments that a robot will face when when it is deployed. However, even restricting attention only to the distribution of objects in a scene, these distributions over environments are nontrivial: they describe mixtures of discrete and continuous variables related to the number, type, poses, and attributes of objects in the scene. We describe a probabilistic generative model that uses scene trees to capture hierarchical relationships between collections of objects, as well as a variational inference algorithm for tuning that model to best match a set of observed environments without any need for tediously labeled parse trees. We demonstrate that this model can accurately capture the distribution of a pair of nontrivial manipulation-relevant datasets and be deployed as a density estimator and outlier detector for novel environments.
更多查看译文
关键词
variational inference algorithm,observed environments,nontrivial manipulation-relevant datasets,scene grammars,discrete variables,continuous variables,probabilistic generative model,scene trees,hierarchical relationships,labeled parse trees
AI 理解论文
溯源树
样例
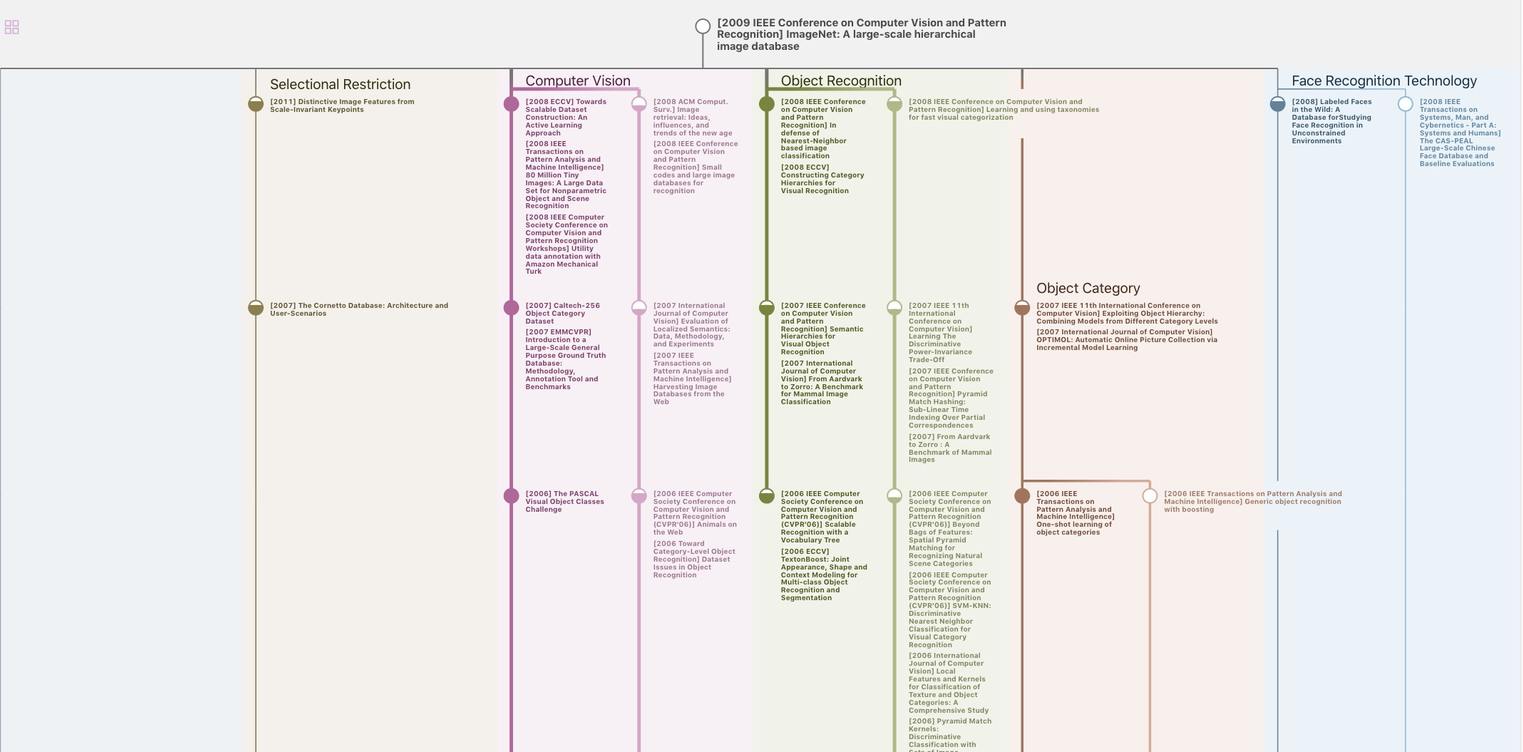
生成溯源树,研究论文发展脉络
Chat Paper
正在生成论文摘要